- Research Areas
- Special Projects
- Sponsors and Funding
My research focuses on the development of novel optimization methods and the application of those methods to solve complex decision-making problems primarily in healthcare and sports.
Inverse Optimization
The goal of inverse optimization is to “reverse engineer” parameters of an optimization model that make a given, observed decision optimal. If it is not possible to make the decision exactly optimal, e.g., the data is noisy or the model is an approximation, then a measure of suboptimality is typically minimized. Viewed through the lens of model fitting, my main interest is to develop new approaches for inverse optimization that optimize and measure data-model fit. Given the increasing amounts of data that are generated as the result of a decision process, I am also interested in finding innovative applications for inverse optimization.
RELEVANT PUBLICATIONS
24. | Conformal inverse optimization Proceedings Article Forthcoming B. Lin, E. Delage, T. C. Y. Chan In: Advances in Neural Information Processing Systems, Forthcoming. @inproceedings{ChanTCY.Oth011c, |
23. | Inverse optimization: Theory and applications Journal Article T. C. Y. Chan, R. Mahmood, I. Y. Zhu In: Operations Research, vol. 73, no. 2, pp. 1046-1074, 2025. @article{ChanTCY.J0128, Inverse optimization describes a process that is the "reverse" of traditional mathematical optimization. Unlike traditional optimization, which seeks to compute optimal decisions given an objective and constraints, inverse optimization takes decisions as input and determines an objective and/or constraints that render these decisions approximately or exactly optimal. In recent years, there has been an explosion of interest in the mathematics and applications of inverse optimization. This paper provides a comprehensive review of both the methodological and application-oriented literature in inverse optimization. |
22. | Inverse optimization on hierarchical networks: An application to breast cancer clinical pathways Journal Article T. C. Y. Chan, K. Forster, S. Habbous, C. Holloway, L. Ieraci, Y. Shalaby, N. Yousefi In: Health Care Management Science, vol. 25, pp. 590-622, 2022. @article{ChanTCY.J099, Clinical pathways are standardized processes that outline the steps required for managing a specific disease. However, patient pathways often deviate from clinical pathways. Measuring the concordance of patient pathways to clinical pathways is important for health system monitoring and informing quality improvement initiatives. In this paper, we develop an inverse optimization-based approach to measuring pathway concordance in breast cancer, a complex disease. We capture this complexity in a hierarchical network that models the patient’s journey through the health system. A novel inverse shortest path model is formulated and solved on this hierarchical network to estimate arc costs, which are used to form a concordance metric to measure the distance between patient pathways and shortest paths (i.e., clinical pathways). Using real breast cancer patient data from Ontario, Canada, we demonstrate that our concordance metric has a statistically significant association with survival for all breast cancer patient subgroups. We also use it to quantify the extent of patient pathway discordances across all subgroups, finding that patients undertaking additional clinical activities constitute the primary driver of discordance in the population. |
21. | Spatial price integration in competitive markets with capacitated transportation networks Journal Article J. R. Birge, T. C. Y. Chan, M. Pavlin, I. Zhu In: Operations Research, vol. 70, no. 3, pp. 1739-1761, 2022. @article{ChanTCY.J095, Spatial price integration is extensively studied in commodity markets as ameans of examining the degree of integration between regions of a geographically diverse market. Many commodity markets that are commonly studied are supported by stable and well-defined transportation networks. In this paper, we analyze the relationship between spatial price integration, that is, the distribution of prices across geographically distinct locations in the market and the features of the underlying transportation network. We characterize this relationship and show that price integration is strongly influenced by the characteristics of the network, especially when there are capacity constraints on links in the network. Our results are summarized using a price decomposition that explicitly isolates the influences of market forces (supply and demand), transportation costs, and capacity constraints among a set of equilibrium prices. We use these theoretical insights to develop a unique discrete optimization methodology to capture spatiotemporal price variations indicative of underlying network bottlenecks. We apply the methodology to gasoline prices in the southeastern United States, where the methodology effectively characterizes the price effects of a series of well-documented network and supply chain disruptions, providing important implications for operations and supply chain management. |
20. | Inverse mixed integer optimization: Polyhedral insights and trust region methods Journal Article M. Bodur, T. C. Y. Chan, I. Zhu In: INFORMS Journal on Computing, vol. 34, no. 3, pp. 1471-1488, 2022. @article{ChanTCY.J090, Inverse optimization—determining parameters of an optimization problem that render a given solution optimal—has received increasing attention in recent years. Although significant inverse optimization literature exists for convex optimization problems, there have been few advances for discrete problems, despite the ubiquity of applications that fundamentally rely on discrete decision making. In this paper, we present a new set of theoretical insights and algorithms for the general class of inverse mixed integer linear optimization problems. Specifically, a general characterization of optimality conditions is established and leveraged to design new cutting plane solution algorithms. Through an extensive set of computational experiments, we show that our methods provide substantial improvements over existing methods in solving the largest and most difficult instances to date. |
19. | An inverse optimization approach to measuring clinical pathway concordance Journal Article T. C. Y. Chan, M. Eberg, K. Forster, C. Holloway, L. Ieraci, Y. Shalaby, N. Yousefi In: Management Science, vol. 68, pp. 1882-1903, 2022. @article{ChanTCY.J088, Clinical pathways outline standardized processes in the delivery of care for a specific disease. Patient journeys through the healthcare system, however, can deviate substantially from these pathways. Given the positive benefits of clinical pathways, it is important to measure the concordance of patient pathways so that variations in health system performance or bottlenecks in the delivery of care can be detected, monitored, and acted upon. This paper proposes the first data-driven inverse optimization approach to measuring pathway concordance in any problem context. Our specific application considers clinical pathway concordance for stage III colon cancer. We develop a novel concordance metric and demonstrate using real patient data from Ontario, Canada that it has a statistically significant association with survival. Our methodological approach considers a patient’s journey as a walk in a directed graph, where the costs on the arcs are derived by solving an inverse shortest path problem. The inverse optimization model uses two sources of information to find the arc costs: reference pathways developed by a provincial cancer agency (primary) and data from real-world patient-related activity from patients with both positive and negative clinical outcomes (secondary). Thus, our inverse optimization framework extends existing models by including data points of both varying “primacy” and “alignment.” Data primacy is addressed through a two-stage approach to imputing the cost vector, whereas data alignment is addressed by a hybrid objective function that aims to minimize and maximize suboptimality error for different subsets of input data. |
18. | Socioeconomically equitable public defibrillator placement using mathematical optimization Journal Article K. H. B. Leung, S. C. Brooks, G. R. Clegg, T. C. Y. Chan In: Resuscitation, vol. 166, pp. 14-20, 2021. @article{ChanTCY.J085, Background: Mathematical optimization can be used to place automated external defibrillators (AEDs) in locations that maximize coverage of out-of-hospital cardiac arrests (OHCAs). We sought to determine whether optimization can improve alignment between AED locations and OHCA counts across levels of socioeconomic deprivation. Methods: All suspected OHCAs and registered AEDs in Scotland between Jan. 2011 and Sept. 2017 were included and mapped to a corresponding socioeconomic deprivation level using the Scottish Index of Multiple Deprivation (SIMD). We used mathematical optimization to determine optimal locations for placing 10%, 25%, 50%, and 100% additional AEDs, as well as locations for relocating existing AEDs. For each AED placement policy, we examined the impact on AED distribution and OHCA “coverage” (suspected OHCA occurring within 100 m of AED) with respect to SIMD quintiles. Results: We identified 49,432 suspected OHCAs and 1532 AEDs. The distribution of existing AED locations across SIMD quintiles significantly diered from the distribution of suspected OHCAs (P < 0.001). Optimization-guided AED placement increased coverage of suspected OHCAs compared to existing AED locations (all P < 0.001). Optimization resulted in more AED placements and increased OHCA coverage in areas of greater socioeconomic deprivation, such that resulting distributions across SIMD quintiles matched the shape of the OHCA count distribution. Optimally relocating existing AEDs achieved similar OHCA coverage levels to that of doubling the number of total AEDs. Conclusions: Mathematical optimization results in AED locations and suspected OHCA coverage that more closely resembles the suspected OHCA distribution and results in more equitable coverage across levels of socioeconomic deprivation. |
17. | OpenKBP: The Open-Access Knowledge-Based Planning Grand Challenge and Dataset Journal Article A. Babier, B. Zhang, R. Mahmood, K. L. Moore, T. G. Purdie, A. L. McNiven, T. C. Y. Chan In: Medical Physics, vol. 48, no. 9, pp. 5549-5561, 2021. @article{ChanTCY.J078, Purpose: To advance fair and consistent comparisons of dose prediction methods for knowledge-based planning (KBP) in radiation therapy research. Methods: We hosted OpenKBP, a 2020 AAPM Grand Challenge, and challenged participants to develop the best method for predicting the dose of contoured computed tomography (CT) images. The models were evaluated according to two separate scores: (a) dose score, which evaluates the full three-dimensional (3D) dose distributions, and (b) dose-volume histogram (DVH) score, which evaluates a set DVH metrics. We used these scores to quantify the quality of the models based on their out-of-sample predictions. To develop and test their models, participants were given the data of 340 patients who were treated for head-and-neck cancer with radiation therapy. The data were partitioned into training (n = 200), validation (n = 40), and testing (n = 100) datasets. All participants performed training and validation with the corresponding datasets during the first (validation) phase of the Challenge. In the second (testing) phase, the participants used their model on the testing data to quantify the out-of-sample performance, which was hidden from participants and used to determine the final competition ranking. Participants also responded to a survey to summarize their models. Results: The Challenge attracted 195 participants from 28 countries, and 73 of those participants formed 44 teams in the validation phase, which received a total of 1750 submissions. The testing phase garnered submissions from 28 of those teams, which represents 28 unique prediction methods. On average, over the course of the validation phase, participants improved the dose and DVH scores of their models by a factor of 2.7 and 5.7, respectively. In the testing phase one model achieved the best dose score (2.429) and DVH score (1.478), which were both significantly better than the dose score (2.564) and the DVH score (1.529) that was achieved by the runner-up models. Lastly, many of the top performing teams reported that they used generalizable techniques (e.g., ensembles) to achieve higher performance than their competition. Conclusion: OpenKBP is the first competition for knowledge-based planning research. The Challenge helped launch the first platform that enables researchers to compare KBP prediction methods fairly and consistently using a large open-source dataset and standardized metrics. OpenKBP has also democratized KBP research by making it accessible to everyone, which should help accelerate the progress of KBP research. The OpenKBP datasets are available publicly to help benchmark future KBP research. |
16. | An ensemble learning framework for model fitting and evaluation in inverse linear optimization Journal Article A. Babier, T. C. Y. Chan, T. Lee, R. Mahmood, D. Terekhov In: INFORMS Journal on Optimization, vol. 3, no. 2, pp. 119-138, 2021. @article{ChanTCY.J075, We develop a generalized inverse optimization framework for fitting the cost vector of a single linear optimization problem given an ensemble of observed decisions. We unify multiple variants in the inverse optimization literature under a common template and derive assumption-free and exact solution methods for each variant. We extend a goodness-of-fit metric previously introduced for the problem with a single observed decision to this new setting, proving and numerically demonstrating several important properties. Finally, to illustrate our framework, we develop a novel inverse optimization-driven procedure for automated radiation therapy treatment planning. Here, the inverse optimization model leverages the combined power of an ensemble of dose predictions produced by different machine learning models to construct clinical treatment plans that better trade off between the competing clinical objectives that are used for plan evaluation in practice. |
15. | The importance of evaluating the complete automated knowledge-based planning pipeline Journal Article A. Babier, R. Mahmood, A. L. McNiven, A. Diamant, T. C. Y. Chan In: Physica Medica, vol. 72, pp. 73-79, 2020. @article{ChanTCY.J064, We determine how prediction methods combine with optimization methods in two-stage knowledge-based planning (KBP) pipelines to produce radiation therapy treatment plans. We trained two dose prediction methods, a generative adversarial network (GAN) and a random forest (RF) with the same 130 treatment plans. The models were applied to 87 out-of-sample patients to create two sets of predicted dose distributions that were used as input to two optimization models. The first optimization model, inverse planning (IP), estimates weights for dose-objectives from a predicted dose distribution and generates new plans using conventional inverse planning. The second optimization model, dose mimicking (DM), minimizes the sum of one-sided quadratic penalties between the predictions and the generated plans using several dose-objectives. Altogether, four KBP pipelines (GAN-IP, GAN-DM, RF-IP, and RF-DM) were constructed and benchmarked against the corresponding clinical plans using clinical criteria; the error of both prediction methods was also evaluated. The best performing plans were GAN-IP plans, which satisfied the same criteria as their corresponding clinical plans (78%) more often than any other KBP pipeline. However, GAN did not necessarily provide the best prediction for the second-stage optimization models. Specifically, both the RF-IP and RF-DM plans satisfied the same criteria as the clinical plans 25% and 15% more often than GAN-DM plans (the worst performing plans), respectively. GAN predictions also had a higher mean absolute error (3.9 Gy) than those from RF (3.6 Gy). We find that state-of-the-art prediction methods when paired with different optimization algorithms, produce treatment plans with considerable variation in quality. |
14. | Knowledge-based automated planning with three-dimensional generative adversarial networks Journal Article A. Babier, R. Mahmood, A. L. McNiven, A. Diamant, T. C. Y. Chan In: Medical Physics, vol. 47, pp. 297-306, 2020. @article{ChanTCY.J059, Purpose: To develop a knowledge-based automated planning pipeline that generates treatment plans without feature engineering, using deep neural network architectures for predicting three-dimensional (3D) dose. Methods: Our knowledge-based automated planning (KBAP) pipeline consisted of a knowledge-based planning (KBP) method that predicts dose for a contoured computed tomography (CT) image followed by two optimization models that learn objective function weights and generate fluence-based plans, respectively. We developed a novel generative adversarial network (GAN)-based KBP approach, a 3D GAN model, which predicts dose for the full 3D CT image at once and accounts for correlations between adjacent CT slices. Baseline comparisons were made against two state-of-the-art deep learning–based KBP methods from the literature. We also developed an additional benchmark, a two-dimensional (2D) GAN model which predicts dose to each axial slice independently. For all models, we investigated the impact of multiplicatively scaling the predictions before optimization, such that the predicted dose distributions achieved all target clinical criteria. Each KBP model was trained on 130 previously delivered oropharyngeal treatment plans. Performance was tested on 87 out-of-sample previously delivered treatment plans. All KBAP plans were evaluated using clinical planning criteria and compared to their corresponding clinical plans. KBP prediction quality was assessed using dose-volume histogram (DVH) differences from the corresponding clinical plans. Results: The best performing KBAP plans were generated using predictions from the 3D GAN model that were multiplicatively scaled. These plans satisfied 77% of all clinical criteria, compared to the clinical plans, which satisfied 67% of all criteria. In general, multiplicatively scaling predictions prior to optimization increased the fraction of clinical criteria satisfaction by 11% relative to the plans generated with nonscaled predictions. Additionally, these KBAP plans satisfied the same criteria as the clinical plans 84% and 8% more frequently as compared to the two benchmark methods, respectively. Conclusions: We developed the first knowledge-based automated planning framework using a 3D generative adversarial network for prediction. Our results, based on 217 oropharyngeal cancer treatment plans, demonstrated superior performance in satisfying clinical criteria and generated more realistic plans as compared to the previous state-of-the-art approaches. |
13. | Inverse optimization for the recovery of constraint parameters Journal Article T. C. Y. Chan, N. Kaw In: European Journal of Operational Research, vol. 282, pp. 415-427, 2020. @article{ChanTCY.J057, Most inverse optimization models impute unspecified parameters of an objective function to make an observed solution optimal for a given optimization problem with a fixed feasible set. We propose two approaches to impute unspecified left-hand-side constraint coefficients in addition to a cost vector for a given linear optimization problem. The first approach identifies parameters minimizing the duality gap, while the second minimally perturbs prior estimates of the unspecified parameters to satisfy strong duality, if it is possible to satisfy the optimality conditions exactly. We apply these two approaches to the general linear optimization problem. We also use them to impute unspecified parameters of the uncertainty set for robust linear optimization problems under interval and cardinality constrained uncertainty. Each inverse optimization model we propose is nonconvex, but we show that a globally optimal solution can be obtained either in closed form or by solving a linear number of linear or convex optimization problems. |
12. | Inverse optimization: Closed-form solutions, geometry, and goodness of fit Journal Article T. C. Y. Chan, T. Lee, D. Terekhov In: Management Science, vol. 65, pp. 1115-1135, 2019. @article{ChanTCY.J050, In classical inverse linear optimization, one assumes that a given solution is a candidate to be optimal. Real data are imperfect and noisy, so there is no guarantee that this assumption is satisfied. Inspired by regression, this paper presents a unified framework for cost function estimation in linear optimization comprising a general inverse optimization model and a corresponding goodness-of-fit metric. Although our inverse optimization model is nonconvex, we derive a closed-form solution and present the geometric intuition. Our goodness-of-fit metric, ρ, the coefficient of complementarity, has similar properties to R^2 from regression and is quasi-convex in the input data, leading to an intuitive geometric interpretation. While ρ is computable in polynomial time, we derive a lower bound that possesses the same properties, is tight for several important model variations, and is even easier to compute. We demonstrate the application of our framework for model estimation and evaluation in production planning and cancer therapy. |
11. | The importance of evaluating the complete knowledge-based automated planning pipeline Proceedings Article A. Babier, R. Mahmood, A. Diamant, A. McNiven, T. C. Y. Chan In: Proceedings of the International Conference on the use of Computers in Radiation Therapy, 2019. @inproceedings{ChanTCY.Oth007c, |
10. | A small number of objective function weight vectors is sufficient for automated treatment planning in prostate cancer Journal Article A. Goli, J. J. Boutilier, M. B. Sharpe, T. Craig, T. C. Y. Chan In: Physics in Medicine and Biology, vol. 63, no. Article No. 195004, 2018. @article{ChanTCY.J045, Current practice for treatment planning optimization can be both inefficient and time consuming. In this paper, we propose an automated planning methodology that aims to combine both explorative and prescriptive approaches for improving the efficiency and the quality of the treatment planning process. Given a treatment plan, our explorative approach explores trade-offs between different objectives and finds an acceptable region for objective function weights via inverse optimization. Intuitively, the shape and size of these regions describe how 'sensitive' a patient is to perturbations in objective function weights. We then develop an integer programming-based prescriptive approach that exploits the information encoded by these regions to find a set of five representative objective function weight vectors such that for each patient there exists at least one representative weight vector that can produce a high quality treatment plan. Using 315 patients from Princess Margaret Cancer Centre, we show that the produced treatment plans are comparable and, for 96% of cases, improve upon the inversely optimized plans that are generated from the historical clinical treatment plans. |
9. | Inverse optimization of objective function weights for treatment planning using clinical dose-volume histograms Journal Article A. Babier, J. J. Boutilier, M. B. Sharpe, A. L. McNiven, T. C. Y. Chan In: Physics in Medicine and Biology, vol. 63, no. Article No. 105004, 2018. @article{ChanTCY.J044, We developed and evaluated a novel inverse optimization (IO) model to estimate objective function weights from clinical dose-volume histograms (DVHs). These weights were used to solve a treatment planning problem to generate 'inverse plans' that had similar DVHs to the original clinical DVHs. Our methodology was applied to 217 clinical head and neck cancer treatment plans that were previously delivered at Princess Margaret Cancer Centre in Canada. Inverse plan DVHs were compared to the clinical DVHs using objective function values, dose-volume differences, and frequency of clinical planning criteria satisfaction. Median differences between the clinical and inverse DVHs were within 1.1 Gy. For most structures, the difference in clinical planning criteria satisfaction between the clinical and inverse plans was at most 1.4%. For structures where the two plans differed by more than 1.4% in planning criteria satisfaction, the difference in average criterion violation was less than 0.5 Gy. Overall, the inverse plans were very similar to the clinical plans. Compared with a previous inverse optimization method from the literature, our new inverse plans typically satisfied the same or more clinical criteria, and had consistently lower fluence heterogeneity. Overall, this paper demonstrates that DVHs, which are essentially summary statistics, provide sufficient information to estimate objective function weights that result in high quality treatment plans. However, as with any summary statistic that compresses three-dimensional dose information, care must be taken to avoid generating plans with undesirable features such as hotspots; our computational results suggest that such undesirable spatial features were uncommon. Our IO-based approach can be integrated into the current clinical planning paradigm to better initialize the planning process and improve planning efficiency. It could also be embedded in a knowledge-based planning or adaptive radiation therapy framework to automatically generate a new plan given a predicted or updated target DVH, respectively. |
8. | Knowledge-based automated planning for oropharyngeal cancer Journal Article A. Babier, J. J. Boutilier, A. L. McNiven, T. C. Y. Chan In: Medical Physics, vol. 45, pp. 2875-2883, 2018. @article{ChanTCY.J043, Purpose: The purpose of this study was to automatically generate radiation therapy plans for oropharynx patients by combining knowledge-based planning (KBP) predictions with an inverse optimization (IO) pipeline. Methods: We developed two KBP approaches, the bagging query (BQ) method and the generalized principal component analysis-based (gPCA) method, to predict achievable dose–volume histograms (DVHs). These approaches generalize existing methods by predicting physically feasible organ-at-risk (OAR) and target DVHs in sites with multiple targets. Using leave-one-out cross validation, we applied both models to a large dataset of 217 oropharynx patients. The predicted DVHs were input into an IO pipeline that generated treatment plans (BQ and gPCA plans) via an intermediate step that estimated objective function weights for an inverse planning model. The KBP predictions were compared to the clinical DVHs for benchmarking. To assess the complete pipeline, we compared the BQ and gPCA plans to both the predictions and clinical plans. To isolate the effect of the KBP predictions, we put clinical DVHs through the IO pipeline to produce clinical inverse optimized (CIO) plans. This approach also allowed us to estimate the complexity of the clinical plans. The BQ and gPCA plans were benchmarked against the CIO plans using DVH differences and clinical planning criteria. Iso-complexity plans (relative to CIO) were also generated and evaluated. Results: The BQ method tended to predict that less dose is delivered than what was observed in the clinical plans while the gPCA predictions were more similar to clinical DVHs. Both populations of KBP predictions were reproduced with inverse plans to within a median DVH difference of 3 Gy. Clinical planning criteria for OARs were satisfied most frequently by the BQ plans (74.4%), by 6.3% points more than the clinical plans. Meanwhile, target criteria were satisfied most frequently by the gPCA plans (90.2%), and by 21.2% points more than clinical plans. However, once the complexity of the plans was constrained to that of the CIO plans, the performance of the BQ plans degraded significantly. In contrast, the gPCA plans still satisfied more clinical criteria than both the clinical and CIO plans, with the most notable improvement being in target criteria. Conclusion: Our automated pipeline can successfully use DVH predictions to generate high-quality plans without human intervention. Between the two KBP methods, gPCA plans tend to achieve comparable performance as clinical plans, even when controlling for plan complexity, whereas BQ plans tended to underperform. |
7. | Trade-off preservation in inverse multi-objective convex optimization Journal Article T. C. Y. Chan, T. Lee In: European Journal of Operational Research, vol. 270, pp. 25-39, 2018. @article{ChanTCY.J040, Given an input solution that may not be Pareto optimal, we present a new inverse optimization methodology for multi-objective convex optimization that determines a weight vector producing a weakly Pareto optimal solution that preserves the decision maker's trade-off intention encoded in the input solution. We introduce a notion of trade-off preservation, which we use as a measure of similarity for approximating the input solution, and show its connection with minimizing an optimality gap. We propose a linear approximation to the inverse model and a successive linear programming algorithm that balance between trade-off preservation and computational efficiency, and show that our model encompasses many of the existing inverse optimization models from the literature. We demonstrate the proposed method using clinical data from prostate cancer radiation therapy. |
6. | Automated treatment planning in radiation therapy using generative adversarial networks Proceedings Article R. Mahmood, A. Babier, A. McNiven, A. Diamant, T. C. Y. Chan In: Proceedings of the 3rd Machine Learning for Healthcare Conference, pp. 484-499, PMLR, 2018. @inproceedings{ChanTCY.Oth006c, Knowledge-based planning (KBP) is an automated approach to radiation therapy treatment planning that involves predicting desirable treatment plans before they are then corrected to deliverable ones. We propose a generative adversarial network (GAN) approach for predicting desirable 3D dose distributions that eschews the previous paradigms of site-specific feature engineering and predicting low-dimensional representations of the plan. Experiments on a dataset of oropharyngeal cancer patients show that our approach significantly outperforms previous methods on several clinical satisfaction criteria and similarity metrics. |
5. | Models for predicting objective function weights in prostate cancer IMRT Journal Article J. J. Boutilier, T. Lee, T. Craig, M. B. Sharpe, T. C. Y. Chan In: Medical Physics, vol. 42, pp. 1586-1595, 2015. @article{ChanTCY.J021, Purpose: To develop and evaluate the clinical applicability of advanced machine learning models that simultaneously predict multiple optimization objective function weights from patient geometry for intensity-modulated radiation therapy of prostate cancer. Methods: A previously developed inverse optimization method was applied retrospectively to determine optimal objective function weights for 315 treated patients. The authors used an overlap volume ratio (OV) of bladder and rectum for different PTV expansions and overlap volume histogram slopes (OVSR and OVSB for the rectum and bladder, respectively) as explanatory variables that quantify patient geometry. Using the optimal weights as ground truth, the authors trained and applied three prediction models: logistic regression (LR), multinomial logistic regression (MLR), and weighted K-nearest neighbor (KNN). The population average of the optimal objective function weights was also calculated. Results: The OV at 0.4 cm and OVSR at 0.1 cm features were found to be the most predictive of the weights. The authors observed comparable performance (i.e., no statistically significant difference) between LR, MLR, and KNN methodologies, with LR appearing to perform the best. All three machine learning models outperformed the population average by a statistically significant amount over a range of clinical metrics including bladder/rectum V53Gy, bladder/rectum V70Gy, and dose to the bladder, rectum, CTV, and PTV. When comparing the weights directly, the LR model predicted bladder and rectum weights that had, on average, a 73% and 74% relative improvement over the population average weights, respectively. The treatment plans resulting from the LR weights had, on average, a rectum V70Gy that was 35% closer to the clinical plan and a bladder V70Gy that was 29% closer, compared to the population average weights. Similar results were observed for all other clinical metrics. Conclusions: The authors demonstrated that the KNN and MLR weight prediction methodologies perform comparably to the LR model and can produce clinical quality treatment plans by simultaneously predicting multiple weights that capture trade-offs associated with sparing multiple OARs. |
4. | Generalized inverse multi-objective optimization with application to cancer therapy Journal Article T. C. Y. Chan, T. Craig, T. Lee, M. B. Sharpe In: Operations Research, vol. 62, pp. 680-695, 2014. @article{ChanTCY.J018, We generalize the standard method of solving inverse optimization problems to allow for the solution of inverse problems that would otherwise be ill posed or infeasible. In multiobjective linear optimization, given a solution that is not a weakly efficient solution to the forward problem, our method generates objective function weights that make the given solution a near-weakly efficient solution. Our generalized inverse optimization model specializes to the standard model when the given solution is weakly efficient and retains the complexity of the underlying forward problem. We provide a novel interpretation of our inverse formulation as the dual of the well-known Benson's method and by doing so develop a new connection between inverse optimization and Pareto surface approximation techniques. We apply our method to prostate cancer data obtained from Princess Margaret Cancer Centre in Toronto, Canada. We demonstrate that clinically acceptable treatments can be generated using a small number of objective functions and inversely optimized weights—current treatments are designed using a complex formulation with a large parameter space in a trial-and-error reoptimization process. We also show that our method can identify objective functions that are most influential in treatment plan optimization. |
3. | Predicting objective function weights from patient anatomy in prostate IMRT treatment planning Journal Article T. Lee, M. Hummad, T. C. Y. Chan, T. Craig, M. B. Sharpe In: Medical Physics, vol. 40, no. Article No. 121706, 2013. @article{ChanTCY.J015, Purpose: Intensity-modulated radiation therapy (IMRT) treatment planning typically combines multiple criteria into a single objective function by taking a weighted sum. The authors propose a statistical model that predicts objective function weights from patient anatomy for prostate IMRT treatment planning. This study provides a proof of concept for geometry-driven weight determination. Methods: A previously developed inverse optimization method (IOM) was used to generate optimal objective function weights for 24 patients using their historical treatment plans (i.e., dose distributions). These IOM weights were around 1% for each of the femoral heads, while bladder and rectum weights varied greatly between patients. A regression model was developed to predict a patient's rectum weight using the ratio of the overlap volume of the rectum and bladder with the planning target volume at a 1 cm expansion as the independent variable. The femoral head weights were fixed to 1% each and the bladder weight was calculated as one minus the rectum and femoral head weights. The model was validated using leave-one-out cross validation. Objective values and dose distributions generated through inverse planning using the predicted weights were compared to those generated using the original IOM weights, as well as an average of the IOM weights across all patients. Results: The IOM weight vectors were on average six times closer to the predicted weight vectors than to the average weight vector, using l_2 distance. Likewise, the bladder and rectum objective values achieved by the predicted weights were more similar to the objective values achieved by the IOM weights. The difference in objective value performance between the predicted and average weights was statistically significant according to a one-sided sign test. For all patients, the difference in rectum V54.3 Gy, rectum V70.0 Gy, bladder V54.3 Gy, and bladder V70.0 Gy values between the dose distributions generated by the predicted weights and IOM weights was less than 5 percentage points. Similarly, the difference in femoral head V54.3 Gy values between the two dose distributions was less than 5 percentage points for all but one patient. Conclusions: This study demonstrates a proof of concept that patient anatomy can be used to predict appropriate objective function weights for treatment planning. In the long term, such geometry-driven weights may serve as a starting point for iterative treatment plan design or may provide information about the most clinically relevant region of the Pareto surface to explore. |
2. | Examining the LEED rating system using inverse optimization Journal Article S. D. O. Turner, T. C. Y. Chan In: Journal of Solar Energy Engineering, vol. 135, no. Article No. 040901, 2013. @article{ChanTCY.J014, The Leadership in Energy and Environmental Design (LEED) rating system is the most recognized green building certification program in North America. In order to be LEED certified, a building must earn a sufficient number of points, which are obtained through achieving certain credits or design elements. In LEED versions 1 and 2, each credit was worth one point. In version 3, the LEED system changed so that certain credits were worth more than one point. In this study, we develop an inverse optimization approach to examine how building designers intrinsically valued design elements in LEED version 2. Because of the change in the point system between version 2 and version 3, we aim to determine whether building designers actually valued each credit equally, and if not, whether their valuations matched the values in version 3. Due to the large dimensionality of the inverse optimization problem, we develop an approximation to improve tractability. We apply our method to 306 different LEED-certified buildings in the continental United States. We find that building designers did not value all credits equally and that other factors such as cost, building type, and size, and certification level play a role in how the credits are valued. Overall, inverse optimization may provide a new method to assess historical data and support the design of future versions of LEED. |
1. | Examining the LEED rating system using approximate inverse optimization Proceedings Article S. D. O. Turner, T. C. Y. Chan In: Proceedings of the ASME 2012 International Mechanical Engineering Congress and Exposition, 2012. @inproceedings{ChanTCY.Oth004c, The Leadership in Energy and Environmental Design (LEED) rating system is the most recognized green building certification program in North America. In order to be LEED certified, a building must earn a certain number of points, which are obtained through achieving certain credits or design elements. Prior to LEED version 3, each credit was worth one point. In this study, we develop an inverse optimization approach to examine how building designers intrinsically valued design elements in LEED version 2. Due to the large dimensionality of the inverse optimization problem, we develop an approximation to improve tractability. We apply our method to 18 different LEED-certified buildings in the United States. We find that building designers did not value all credits equally and that other factors such as cost and certification level play a role in how the credits are valued. Overall, inverse optimization may provide a new method to assess historical data and support the design of future versions of LEED. |
Robust Optimization
Robust optimization is used to optimize in the face of uncertainty. I am interested in theory, modeling, solution methods, and applications of robust optimization. The majority of my work to date has been in robust optimization, especially applied to radiation therapy treatment planning.
RELEVANT PUBLICATIONS
23. | Robust direct aperture optimization for radiation therapy treatment planning Journal Article D. A. Ripsman, T. G. Purdie, T. C. Y. Chan, H. Mahmoudzadeh In: INFORMS Journal on Computing, vol. 34, no. 4, pp. 2017-2038, 2022. @article{ChanTCY.J093, Intensity-modulated radiation therapy (IMRT) allows for the design of customized, highly-conformal treatments for cancer patients. Creating IMRT treatment plans, however, is a mathematically complex process, which is often tackled in multiple, simpler stages. This sequential approach typically separates radiation dose requirements from mechanical deliverability considerations, which may result in suboptimal treatment quality. For patient health to be considered paramount, holistic models must address these plan elements concurrently, eliminating quality loss between stages. This combined direct aperture optimization (DAO) approach is rarely paired with uncertainty mitigation techniques, such as robust optimization, due to the inherent complexity of both parts. This paper outlines a robust DAO (RDAO) model and discusses novel methodologies for efficiently integrating salient constraints. Since the highly-complex RDAO model is difficult to solve, an original candidate plan generation (CPG) heuristic is proposed. The CPG produces rapid, high-quality, feasible plans, which are immediately clinically viable, and can also be used to generate a feasible incumbent solution for warm starting the RDAO model. Computational results obtained using clinical patient datasets with motion uncertainty show the benefit of incorporating the CPG, both in terms of first incumbent solution and final output plan quality. |
22. | Ambulance Emergency Response Optimization in developing countries Journal Article J. J. Boutilier, T. C. Y. Chan In: Operations Research, vol. 68, pp. 1315-1334, 2020. @article{ChanTCY.J060, The lack of emergency medical transportation is viewed as the main barrier to the access and availability of emergency medical care in low- and middle-income countries (LMICs). In this paper, we present a robust optimization approach to optimize both the location and routing of emergency response vehicles, accounting for uncertainty in travel times and spatial demand characteristic of LMICs. We traveled to Dhaka, Bangladesh, the sixth largest and third most densely populated city in the world, to conduct field research resulting in the collection of two unique data sets that inform our approach. These data are leveraged to estimate demand for emergency medical services in an LMIC setting and to predict the travel time between any two locations in the road network for different times of day and days of the week. We combine our prediction-optimization framework with a simulation model and real data to provide an in-depth investigation into three policy- related questions. First, we demonstrate that outpost locations optimized for weekday rush hour lead to good performance for all times of day and days of the week. Second, we find that the performance of the current system could be replicated using one third of the current outpost locations and one half of the current number of ambulances. Finally, we show that a fleet of small ambulances has the potential to significantly outperform tra- ditional ambulance vans. In particular, they are able to capture approximately three times more demand while reducing the median average response time by roughly 10%–18% over the entire week and 24%–35% during rush hour because of increased routing flexibility offered by more nimble vehicles on a larger road network. Our results provide practical insights for emergency response optimization that can be leveraged by hospital-based and private ambulance providers in Dhaka and other urban centers in developing countries. |
21. | Process flexibility in baseball: The value of positional flexibility Journal Article T. C. Y. Chan, D. Fearing In: Management Science, vol. 65, pp. 1642-1666, 2019. @article{ChanTCY.J052, This paper introduces the formal study of process flexibility to the novel domain of sports analytics. In baseball, positional flexibility is the analogous concept to process flexibility from manufacturing. We study the flexibility of players (plants) on a baseball team who produce innings-played at different positions (products). We develop models and metrics to evaluate expected and worst-case performance under injury risk (capacity uncertainty) with continuous player-position capabilities. Using Major League Baseball data, we quantify the impact of flexibility on team and individual performance and explore the player chains that arise when injuries occur. We discover that top teams can attribute at least one to two wins per season to flexibility alone, generally as a result of long subchains in the infield or outfield. The least robust teams to worst-case injury, those whose performance is driven by one or two star players, are over four times as fragile as the most robust teams. We evaluate several aspects of individual flexibility, such as how much value individual players bring to their team in terms of average and worst-case performance. Finally, we demonstrate the generalizability of our framework for player evaluation by quantifying the value of potential free agent additions and uncovering the true 'MVP' of a team. |
20. | Robust radiotherapy planning Journal Article J. Unkelbach, M. Alber, M. Bangert, R. Bokrantz, T. C. Y. Chan, J. Deasy, A. Fredriksson, B. L. Gorissen, M. van Herk, W. Liu, H. Mahmoudzadeh, O. Nohadani, J. V. Siebers, M. Witte, H. Xu In: Physics in Medicine and Biology, vol. 63, no. Article No. 22TR02, 2018. @article{ChanTCY.J049, Motion and uncertainty in radiotherapy is traditionally handled via margins. The clinical target volume (CTV) is expanded to a larger planning target volume (PTV), which is irradiated to the prescribed dose. However, the PTV concept has several limitations, especially in proton therapy. Therefore, robust and probabilistic optimization methods have been developed that directly incorporate motion and uncertainty into treatment plan optimization for intensity modulated radiotherapy (IMRT) and intensity modulated proton therapy (IMPT). Thereby, the explicit definition of a PTV becomes obsolete and treatment plan optimization is directly based on the CTV. Initial work focused on random and systematic setup errors in IMRT. Later, inter-fraction prostate motion and intra-fraction lung motion became a research focus. Over the past ten years, IMPT has emerged as a new application for robust planning methods. In proton therapy, range or setup errors may lead to dose degradation and misalignment of dose contributions from different beams – a problem that cannot generally be addressed by margins. Therefore, IMPT has led to the first implementations of robust planning methods in commercial planning systems, making these methods available for clinical use. This paper first summarizes the limitations of the PTV concept. Subsequently, robust optimization methods are introduced and their applications in IMRT and IMPT planning are reviewed. |
19. | Robust defibrillator deployment under cardiac arrest location uncertainty via row-and-column generation Journal Article T. C. Y. Chan, Z.-J. Shen, A. Siddiq In: Operations Research, vol. 66, pp. 358-379, 2018. @article{ChanTCY.J038, Sudden cardiac arrest is a significant public health concern. Successful treatment of cardiac arrest is extremely time sensitive, and use of an automated external defibrillator (AED) where possible significantly increases the probability of survival. Placement of AEDs in public locations can improve survival by enabling bystanders to treat victims of cardiac arrest prior to the arrival of emergency medical responders, thus shortening the time between collapse and treatment. However, since the exact locations of future cardiac arrests cannot be known a priori, AEDs must be placed strategically in public locations to ensure their accessibility in the event of an out-of-hospital cardiac arrest emergency. In this paper, we propose a data-driven optimization model for deploying AEDs in public spaces while accounting for uncertainty in future cardiac arrest locations. Our approach involves discretizing a continuous service area into a large set of scenarios, where the probability of cardiac arrest at each location is itself uncertain. We model uncertainty in the spatial risk of cardiac arrest using a polyhedral uncertainty set that we calibrate using historical cardiac arrest data. We propose a solution technique based on row-and-column generation that exploits the structure of the uncertainty set, allowing the algorithm to scale gracefully with the total number of scenarios. Using real cardiac arrest data from the City of Toronto, we conduct an extensive numerical study on AED deployment public locations. We find that hedging against cardiac arrest location uncertainty can produce AED deployments that outperform an intuitive sample average approximation by 9%–15% and cuts the performance gap with respect to an ex post model by half. Our findings suggest that accounting for cardiac arrest location uncertainty can lead to improved accessibility of AEDs during cardiac arrest emergencies and the potential for improved survival outcomes. |
18. | Stability and continuity in robust optimization Journal Article T. C. Y. Chan, P. A. Mar In: SIAM Journal on Optimization, vol. 27, pp. 817-841, 2017. @article{ChanTCY.J036, We consider the stability of robust optimization (RO) problems with respect to perturbations in their uncertainty sets. In particular, we focus on robust linear optimization problems, including those with an infinite number of constraints, and consider uncertainty in both the cost function and constraints. We prove Lipschitz continuity of the optimal value and ε-approximate optimal solution set with respect to the Hausdorff distance between uncertainty sets. The Lipschitz constant can be calculated from the problem data. In addition, we prove closedness and upper semicontinuity for the optimal solution set with respect to the uncertainty set. In order to prove these results, we develop a novel transformation that maps RO problems to linear semi-infinite optimization (LSIO) problems in such a way that the distance between uncertainty sets of two RO problems correspond to a measure of distance between their equivalent LSIO problems. Using this isometry we leverage LSIO and variational analysis stability results to obtain stability results for RO problems. |
17. | Robust optimization methods Book Chapter T. C. Y. Chan, P. A. C. Mar In: Terlaky, T.; Anjos, M.; Ahmed, S. (Ed.): Advances and Trends in Optimization with Engineering Applications, Chapter 25, pp. 333-344, SIAM, Philadelphia, 2017, ISBN: 978-1-61197-468-3. @inbook{ChanTCY.Oth010, Uncertainty in the parameters of an optimization problem can have a large impact on the quality of the resulting solutions, even rendering such solutions infeasible if unexpected scenarios arise [212]. Robust optimization is one method for optimizing in the presence of uncertainty. The last 15 years have seen an explosion in activity in robust optimization driven by initial work done to explicitly reformulate several different optimization models under set-based uncertainty as tractable mathematical optimization problems. The success of robust optimization can be attributed to its computational tractability and its ability to produce quality solutions for a large class of problems. From a modeling viewpoint, robust optimization conveniently does not require complete knowledge of the probability distribution of the uncertain parameters. |
16. | Robust wind farm layout optimization Book Chapter P. Y. Zhang, J. Y. J. Kuo, D. Romero, T. C. Y. Chan, C. H. Amon In: Terlaky, T.; Anjos, M.; Ahmed, S. (Ed.): Advances and Trends in Optimization with Engineering Applications, Chapter 28, pp. 367-374, SIAM, Philadelphia, 2017, ISBN: 978-1-61197-468-3. @inbook{ChanTCY.Oth009, The number of commercial-scale wind farms and total installed capacity have grown dramatically over the past two decades [1958]. Relying on an essentially free but intermittent energy source and spanning large areas of land or water, wind farms face unique challenges in their design, installation, and operations. Two types of optimization problems arise naturally from this context: (a) optimization of aggregated energy output by strategic turbine placement and (b) optimization of electrical dispatch policies to reduce the economic impact of wind intermittency. In this chapter, we apply robust optimization to the former and discuss the potential benefits on both total energy output and variability in energy output, which can impact dispatch policies. |
15. | Constraint generation methods for robust optimization in radiation therapy Journal Article H. Mahmoudzadeh, T. G. Purdie, T. C. Y. Chan In: Operations Research for Health Care, vol. 8, pp. 85-90, 2016. @article{ChanTCY.J027, We develop a constraint generation solution method for robust optimization problems in radiation therapy in which the problems include a large number of robust constraints. Each robust constraint must hold for any realization of an uncertain parameter within a given uncertainty set. Because the problems are large scale, the robust counterpart is computationally challenging to solve. To address this challenge, we explore different strategies of adding constraints in a constraint generation solution approach. We motivate and demonstrate our approach using robust intensity-modulated radiation therapy treatment planning for breast cancer. We use clinical data to compare the computational efficiency of our constraint generation strategies with that of directly solving the robust counterpart. |
14. | Robust PET-guided intensity-modulated radiation therapy Journal Article H. Li, J. P. Bissonnette, T. Purdie, T. C. Y. Chan In: Medical Physics, vol. 42, pp. 4863-4871, 2015. @article{ChanTCY.J025, Purpose: Functional image guided intensity-modulated radiation therapy has the potential to improve cancer treatment quality by basing treatment parameters such as heterogeneous dose distributions information derived from imaging. However, such heterogeneous dose distributions are subject to imaging uncertainty. In this paper, the authors develop a robust optimization model to design plans that are desensitized to imaging uncertainty. Methods: Starting from the pretreatment fluorodeoxyglucose-positron emission tomography scans, the authors use the raw voxel standard uptake values (SUVs) as input into a series of intermediate functions to transform the SUV into a desired dose. The calculated desired doses were used as an input into a robust optimization model to generate beamlet intensities. For each voxel, the authors assume that the true SUV cannot be observed but instead resides in an interval centered on the nominal (i.e., observed) SUV. Then the authors evaluated the nominal and robust solutions through a simulation study. The simulation considered the effect of the true SUV being different from the nominal SUV on the quality of the treatment plan. Treatment plans were compared on the metrics of objective function value and tumor control probability (TCP). Results: Computational results demonstrate the potential for improvements in tumor control probability and deviation from the desired dose distribution compared to a nonrobust model while maintaining acceptable tissue dose. Conclusions: Robust optimization can help design treatment plans that are more stable in the presence of image value uncertainties. |
13. | The perils of adapting to dose errors in radiation therapy Journal Article V. V. Mišić, T. C. Y. Chan In: PLOS ONE, vol. 10, no. Article No. e0125335, 2015. @article{ChanTCY.J024, We consider adaptive robust methods for lung cancer that are also dose-reactive, wherein the treatment is modified after each treatment session to account for the dose delivered in prior treatment sessions. Such methods are of interest because they potentially allow for errors in the delivered dose to be corrected as the treatment progresses, thereby ensuring that the tumor receives a sufficient dose at the end of the treatment. We show through a computational study with real lung cancer patient data that while dose reaction is beneficial with respect to the final dose distribution, it may lead to exaggerated daily underdose and overdose relative to non-reactive methods that grows as the treatment progresses. However, by combining dose reaction with a mechanism for updating an estimate of the uncertainty, the magnitude of this growth can be mitigated substantially. The key finding of this paper is that reacting to dose errors – an adaptation strategy that is both simple and intuitively appealing – may backfire and lead to treatments that are clinically unacceptable. |
12. | Adaptive and robust radiation therapy in the presence of drift Journal Article P. A. Mar, T. C. Y. Chan In: Physics in Medicine and Biology, vol. 60, pp. 3599-3615, 2015. @article{ChanTCY.J023, Combining adaptive and robust optimization in radiation therapy has the potential to mitigate the negative effects of both intrafraction and interfraction uncertainty over a fractionated treatment course. A previously developed adaptive and robust radiation therapy (ARRT) method for lung cancer was demonstrated to be effective when the sequence of breathing patterns was well-behaved. In this paper, we examine the applicability of the ARRT method to less well-behaved breathing patterns. We develop a novel method to generate sequences of probability mass functions that represent different types of drift in the underlying breathing pattern. Computational results derived from applying the ARRT method to these sequences demonstrate that the ARRT method is effective for a much broader class of breathing patterns than previously demonstrated. |
11. | Robust optimization methods for cardiac sparing in tangential breast IMRT Journal Article H. Mahmoudzadeh, J. Lee, T. C. Y. Chan, T. G. Purdie In: Medical Physics, vol. 42, pp. 2212-2222, 2015. @article{ChanTCY.J022, Purpose: In left-sided tangential breast intensity modulated radiation therapy (IMRT), the heart may enter the radiation field and receive excessive radiation while the patient is breathing. The patient's breathing pattern is often irregular and unpredictable. We verify the clinical applicability of a heart-sparing robust optimization approach for breast IMRT. We compare robust optimized plans with clinical plans at free-breathing and clinical plans at deep inspiration breath-hold (DIBH) using active breathing control (ABC). Methods: Eight patients were included in the study with each patient simulated using 4D-CT. The 4D-CT image acquisition generated ten breathing phase datasets. An average scan was constructed using all the phase datasets. Two of the eight patients were also imaged at breath-hold using ABC. The 4D-CT datasets were used to calculate the accumulated dose for robust optimized and clinical plans based on deformable registration. We generated a set of simulated breathing probability mass functions, which represent the fraction of time patients spend in different breathing phases. The robust optimization method was applied to each patient using a set of dose-influence matrices extracted from the 4D-CT data and a model of the breathing motion uncertainty. The goal of the optimization models was to minimize the dose to the heart while ensuring dose constraints on the target were achieved under breathing motion uncertainty. Results: Robust optimized plans were improved or equivalent to the clinical plans in terms of heart sparing for all patients studied. The robust method reduced the accumulated heart dose (D10cc) by up to 801 cGy compared to the clinical method while also improving the coverage of the accumulated whole breast target volume. On average, the robust method reduced the heart dose (D10cc) by 364 cGy and improved the optBreast dose (D99%) by 477 cGy. In addition, the robust method had smaller deviations from the planned dose to the accumulated dose. The deviation of the accumulated dose from the planned dose for the optBreast (D99%) was 12 cGy for robust versus 445 cGy for clinical. The deviation for the heart (D10cc) was 41 cGy for robust and 320 cGy for clinical. Conclusions: The robust optimization approach can reduce heart dose compared to the clinical method at free-breathing and can potentially reduce the need for breath-hold techniques. |
10. | A robust-CVaR optimization approach with application to breast cancer therapy Journal Article T. C. Y. Chan, H. Mahmoudzadeh, T. G. Purdie In: European Journal of Operational Research, vol. 238, pp. 876-885, 2014. @article{ChanTCY.J020, We present a framework to optimize the conditional value-at-risk (CVaR) of a loss distribution under uncertainty. Our model assumes that the loss distribution is dependent on the state of some system and the fraction of time spent in each state is uncertain. We develop and compare two robust-CVaR formulations that take into account this type of uncertainty. We motivate and demonstrate our approach using radiation therapy treatment planning of breast cancer, where the uncertainty is in the patient's breathing motion and the states of the system are the phases of the patient's breathing cycle. We use a CVaR representation of the tails of the dose distribution to the points in the body and account for uncertainty in the patient's breathing pattern that affects the overall dose distribution. |
9. | Adaptive and robust radiation therapy optimization for lung cancer Journal Article T. C. Y. Chan, V. V. Mišić In: European Journal of Operational Research, vol. 231, pp. 745-756, 2013. @article{ChanTCY.J012, A previous approach to robust intensity-modulated radiation therapy (IMRT) treatment planning for moving tumors in the lung involves solving a single planning problem before the start of treatment and using the resulting solution in all of the subsequent treatment sessions. In this paper, we develop an adaptive robust optimization approach to IMRT treatment planning for lung cancer, where information gathered in prior treatment sessions is used to update the uncertainty set and guide the reoptimization of the treatment for the next session. Such an approach allows for the estimate of the uncertain effect to improve as the treatment goes on and represents a generalization of existing robust optimization and adaptive radiation therapy methodologies. Our method is computationally tractable, as it involves solving a sequence of linear optimization problems. We present computational results for a lung cancer patient case and show that using our adaptive robust method, it is possible to attain an improvement over the traditional robust approach in both tumor coverage and organ sparing simultaneously. We also prove that under certain conditions our adaptive robust method is asymptotically optimal, which provides insight into the performance observed in our computational study. The essence of our method – solving a sequence of single-stage robust optimization problems, with the uncertainty set updated each time – can potentially be applied to other problems that involve multi-stage decisions to be made under uncertainty. |
8. | The value of flexibility in baseball roster construction Proceedings Article T. C. Y. Chan, D. S. Fearing In: Proceedings of the 7th Annual MIT Sloan Sports Analytics Conference, 2013. @inproceedings{ChanTCY.Oth005c, Drawing inspiration from the theory of production flexibility in manufacturing networks, we provide the first optimization-based analysis of the value of positional flexibility (the ability of a player to play multiple positions) for a major league baseball team in the presence of injury risk. First, we develop novel statistical models to estimate (1) the likelihood and duration of player injuries during the regular season, and (2) fielding abilities at secondary fielding positions. Next, we develop a robust optimization model to calculate the degradation in team performance due to injuries. Finally, we apply this model to measure the difference in performance between a team with players who have positional flexibility and a team that does not. We find that using 2012 rosters, flexibility was expected to create from 3% (White Sox) to 15% (Cubs) in value for each team, measured in runs above replacement. In analyzing the results, we find that platoon advantages (e.g., having left-handed batters face right-handed pitchers) form an important component of flexibility. As a secondary finding, based on our statistical analysis of injuries, we find that the likelihood of injury increases with age, but the duration of injury does not. |
7. | Motion-compensating intensity maps in intensity-modulated radiation therapy Journal Article T. C. Y. Chan In: IIE Transactions on Healthcare Systems Engineering, vol. 3, pp. 1-22, 2013. @article{ChanTCY.J008, Managing the effects of tumor motion during radiation therapy is critical to ensuring that a robust treatment is delivered to a cancer patient. Tumor motion due to patient breathing may result in the tumor moving in and out of the beam of radiation, causing the edge of the tumor to be underdosed. One approach to managing the effects of motion is to increase the intensity of the radiation delivered at the edge of the tumor—an edge-enhanced intensity map—which decreases the likelihood of underdosing that area. A second approach is to use a margin, which increases the volume of irradiation surrounding the tumor, also with the aim of reducing the risk of underdosage. In this paper, we characterize the structure of optimal solutions within these two classes of intensity maps. We prove that the ratio of the tumor size to the standard deviation of motion characterizes the structure of an optimal edge-enhanced intensity map. Similar results are derived for a three-dimensional margin case. Furthermore, we extend our analysis by considering a robust version of the problem where the parameters of the underlying motion distribution are not known with certainty, but lie in pre-specified intervals. We show that the robust counterpart of the uncertain 3D margin problem has a very similar structure to the nominal (no uncertainty) problem. |
6. | Optimal margin and edge-enhanced intensity maps in the presence of motion and uncertainty Journal Article T. C. Y. Chan, J. N. Tsitsiklis, T. Bortfeld In: Physics in Medicine and Biology, vol. 55, pp. 515-533, 2010. @article{ChanTCY.J006, In radiation therapy, intensity maps involving margins have long been used to counteract the effects of dose blurring arising from motion. More recently, intensity maps with increased intensity near the edge of the tumour (edge enhancements) have been studied to evaluate their ability to offset similar effects that affect tumour coverage. In this paper, we present a mathematical methodology to derive margin and edge-enhanced intensity maps that aim to provide tumour coverage while delivering minimum total dose. We show that if the tumour is at most about twice as large as the standard deviation of the blurring distribution, the optimal intensity map is a pure scaling increase of the static intensity map without any margins or edge enhancements. Otherwise, if the tumour size is roughly twice (or more) the standard deviation of motion, then margins and edge enhancements are preferred, and we present formulae to calculate the exact dimensions of these intensity maps. Furthermore, we extend our analysis to include scenarios where the parameters of the motion distribution are not known with certainty, but rather can take any value in some range. In these cases, we derive a similar threshold to determine the structure of an optimal margin intensity map. |
5. | Experimental evaluation of a robust optimization method for IMRT of moving targets Journal Article C. Vrančić, A. Trofimov, T. C. Y. Chan, G. C. Sharp, T. Bortfeld In: Physics in Medicine and Biology, vol. 54, pp. 2901-2914, 2009. @article{ChanTCY.J005, Internal organ motion during radiation therapy, if not considered appropriately in the planning process, has been shown to reduce target coverage and increase the dose to healthy tissues. Standard planning approaches, which use safety margins to handle intrafractional movement of the tumor, are typically designed based on the maximum amplitude of motion, and are often overly conservative. Comparable coverage and reduced dose to healthy organs appear achievable with robust motion-adaptive treatment planning, which considers the expected probability distribution of the average target position and the uncertainty of its realization during treatment delivery. A dosimetric test of a robust optimization method for IMRT was performed, using patient breathing data. External marker motion data acquired from respiratory-gated radiotherapy patients were used to build and test the framework for robust optimization. The motion trajectories recorded during radiation treatment itself are not strictly necessary to generate the initial version of a robust treatment plan, but can be used to adapt the plan during the course of treatment. Single-field IMRT plans were optimized to deliver a uniform dose to a rectangular area. During delivery on a linear accelerator, a computer-driven motion phantom reproduced the patients' breathing patterns and a two-dimensional ionization detector array measured the dose delivered. The dose distributions from robust-optimized plans were compared to those from standard plans, which used a margin expansion. Dosimetric tests confirmed the improved sparing of the non-target area with robust planning, which was achieved without compromising the target coverage. The maximum dose in robust plans did not exceed 110% of the prescription, while the minimum target doses were comparable in standard and robust plans. In test courses, optimized for a simplified target geometry, and delivered to a phantom that moved in one dimension with an average amplitude of 17 mm, the robust treatment design produced a reduction of more than 12% of the integral dose to non-target areas, compared to the standard plan using 10 mm margin expansion. |
4. | Robust management of motion uncertainty in intensity modulated radiation therapy Journal Article T. Bortfeld, T. C. Y. Chan, A. Trofimov, J. N. Tsitsiklis In: Operations Research, vol. 56, pp. 1461-1473, 2008. @article{ChanTCY.J004, Radiation therapy is subject to uncertainties that need to be accounted for when determining a suitable treatment plan for a cancer patient. For lung and liver tumors, the presence of breathing motion during treatment is a challenge to the effective and reliable delivery of the radiation. In this paper, we build a model of motion uncertainty using probability density functions that describe breathing motion, and provide a robust formulation of the problem of optimizing intensity-modulated radiation therapy. We populate our model with real patient data and measure the robustness of the resulting solutions on a clinical lung example. Our robust framework generalizes current mathematical programming formulations that account for motion, and gives insight into the trade-off between sparing the healthy tissues and ensuring that the tumor receives sufficient dose. For comparison, we also compute solutions to a nominal (no uncertainty) and margin (worst-case) formulation. In our experiments, we found that the nominal solution typically underdosed the tumor in the unacceptable range of 6% to 11%, whereas the robust solution underdosed by only 1% to 2% in the worst case. In addition, the robust solution reduced the total dose delivered to the main organ-at-risk (the left lung) by roughly 11% on average, as compared to the margin solution. |
3. | Optimization under uncertainty in radiation therapy PhD Thesis T. C. Y. Chan Sloan School of Management, MIT, 2007. @phdthesis{Chan2007, In the context of patient care for life-threatening illnesses, the presence of uncertainty may compromise the quality of a treatment. In this thesis, we investigate robust approaches to managing uncertainty in radiation therapy treatments for cancer. In the first part of the thesis, we study the effect of breathing motion uncertainty on intensity-modulated radiation therapy treatments of a lung tumor. We construct a robust framework that generalizes current mathematical programming formulations that account for motion. This framework gives insight into the trade-off between sparing the healthy tissues and ensuring that the tumor receives sufficient dose. With this trade-off in mind, we show that our robust solution outperforms a nominal (no uncertainty) solution and a margin (worst-case) solution on a clinical case. Next, we perform an in-depth study into the structure of different intensity maps that were witnessed in the first part of the thesis. We consider parameterized intensity maps and investigate their ability to deliver a sufficient dose to the tumor in the presence of motion that follows a Gaussian distribution. We characterize the structure of optimal intensity maps in terms of certain conditions on the problem parameters. Finally, in the last part of the thesis, we study intensity-modulated proton therapy under uncertainty in the location of maximum dose deposited by the beamlets of radiation. We provide a robust formulation for the optimization of proton-based treatments and show that it outperforms traditional formulations in the face of uncertainty. In our computational experiments, we see evidence that optimal robust solutions use the physical characteristics of the proton beam to create dose distributions that are far less sensitive to the underlying uncertainty. |
2. | Accounting for range uncertainties in the optimization of intensity modulated proton therapy Journal Article J. Unkelbach, T. C. Y. Chan, T. Bortfeld In: Physics in Medicine and Biology, vol. 52, pp. 2755-2773, 2007. @article{ChanTCY.J002, Treatment plans optimized for intensity modulated proton therapy (IMPT) may be sensitive to range variations. The dose distribution may deteriorate substantially when the actual range of a pencil beam does not match the assumed range. We present two treatment planning concepts for IMPT which incorporate range uncertainties into the optimization. The first method is a probabilistic approach. The range of a pencil beam is assumed to be a random variable, which makes the delivered dose and the value of the objective function a random variable too. We then propose to optimize the expectation value of the objective function. The second approach is a robust formulation that applies methods developed in the field of robust linear programming. This approach optimizes the worst case dose distribution that may occur, assuming that the ranges of the pencil beams may vary within some interval. Both methods yield treatment plans that are considerably less sensitive to range variations compared to conventional treatment plans optimized without accounting for range uncertainties. In addition, both approaches—although conceptually different—yield very similar results on a qualitative level. |
1. | A robust approach to IMRT optimization Journal Article T. C. Y. Chan, T. Bortfeld, J. N. Tsitsiklis In: Physics in Medicine and Biology, vol. 51, pp. 2567-2583, 2006. @article{ChanTCY.J001, Managing uncertainty is a major challenge in radiation therapy treatment planning, including uncertainty induced by intrafraction motion, which is particularly important for tumours in the thorax and abdomen. Common methods to account for motion are to introduce a margin or to convolve the static dose distribution with a motion probability density function. Unlike previous work in this area, our development does not assume that the patient breathes according to a fixed distribution, nor is the patient required to breathe the same way throughout the treatment. Despite this generality, we create a robust optimization framework starting from the convolution method that is robust to fluctuations in breathing motion, yet spares healthy tissue better than a margin solution. We describe how to generate the data for our model using breathing motion data and we test our model on a computer phantom using data from real patients. In our numerical results, the robust solution delivers approximately 38% less dose to the healthy tissue than the margin solution, while providing the same level of protection against breathing uncertainty. |
Machine Learning
RELEVANT PUBLICATIONS
36. | Got (optimal) milk? Pooling donations in human milk banks with machine learning and optimization Journal Article Forthcoming T. C. Y. Chan, R. Mahmood, D. O'Connor, D. Stone, S. Unger, R. Wong, I. Y. Zhu In: Manufacturing & Service Operations Management, Forthcoming. @article{ChanTCY.J0127, |
35. | Learning to optimize contextually constrained problems for real-time decision-generation Journal Article A. Babier, T. C. Y. Chan, A. Diamant, R. Mahmood In: Management Science, vol. 71, no. 2, pp. 1165-1186, 2025. @article{ChanTCY.J0126, The topic of learning to solve optimization problems has received interest from both the operations research and machine learning communities where each considers contrasting approaches: conditional stochastic optimization frameworks solved using provably optimal structured models versus deep learning models that leverage large data sets to yield empirically effective decision estimators. In this work, we combine the best of both worlds to solve the problem of learning to generate decisions to instances of continuous optimization problems where the feasible set varies with contextual features. We propose a novel framework for training a generative model to estimate optimal decisions by combining interior point methods and adversarial learning which we further embed within an active learning algorithm. Decisions generated by our model satisfy in-sample and out-of-sample optimality guarantees. Finally, we investigate case studies in portfolio optimization and personalized treatment design, demonstrating that our approach yields significant advantages over predict-then-optimize and supervised deep learning techniques, respectively. |
34. | Lung- and diaphragm-protective strategies in acute respiratory failure: An in silico trial Journal Article D. Ratano, B. Zhang, J. Dianti, D. Georgopoulos, L. J. Brochard, T. C. Y. Chan, E. C. Goligher In: Intensive Care Medicine Experimental, vol. 12, pp. 20, 2024. @article{ChanTCY.J0116, Background: Lung- and diaphragm-protective (LDP) ventilation may prevent diaphragm atrophy and patient self-inflicted lung injury in acute respiratory failure, but feasibility is uncertain. The objectives of this study were to estimate the proportion of patients achieving LDP targets in different modes of ventilation, and to identify predictors of need for extracorporeal carbon dioxide removal (ECCO2R) to achieve LDP targets. Methods: An in silico clinical trial was conducted using a previously published mathematical model of patient–ventilator interaction in a simulated patient population (n = 5000) with clinically relevant physiological characteristics. Ventilation and sedation were titrated according to a pre-defined algorithm in pressure support ventilation (PSV) and proportional assist ventilation (PAV+) modes, with or without adjunctive ECCO2R, and using ECCO2R alone (without ventilation or sedation). Random forest modelling was employed to identify patient-level factors associated with achieving targets. Results: After titration, the proportion of patients achieving targets was lower in PAV+ vs. PSV (37% vs. 43%, odds ratio 0.78, 95% CI 0.73–0.85). Adjunctive ECCO2R substantially increased the probability of achieving targets in both PSV and PAV+ (85% vs. 84%). ECCO2R alone without ventilation or sedation achieved LDP targets in 9%. The main determinants of success without ECCO2R were lung compliance, ventilatory ratio, and strong ion difference. In silico trial results corresponded closely with the results obtained in a clinical trial of the LDP titration algorithm (n = 30). Conclusions: In this in silico trial, many patients required ECCO2R in combination with mechanical ventilation and sedation to achieve LDP targets. ECCO2R increased the probability of achieving LDP targets in patients with intermediate degrees of derangement in elastance and ventilatory ratio. |
33. | AutoLTS: Automating cycling stress assessment via contrastive learning and spatial post-processing Proceedings Article B. Lin, S. Saxe, T. C. Y. Chan In: Proceedings of the AAAI Conference on Artificial Intelligence, Vol. 38, pp. 22222-22230, 2024. @inproceedings{ChanTCY.Oth008c, Cycling stress assessment, which quantifies cyclists’ perceived stress imposed by the built environment and motor traffics, increasingly informs cycling infrastructure planning and cycling route recommendation. However, currently calculating cycling stress is slow and data-intensive, which hinders its broader application. In this paper, We propose a deep learning framework to support accurate, fast, and large-scale cycling stress assessments for urban road networks based on street-view images. Our framework features i) a contrastive learning approach that leverages the ordinal relation- ship among cycling stress labels, and ii) a post-processing technique that enforces spatial smoothness into our predictions. On a dataset of 39,153 road segments collected in Toronto, Canada, our results demonstrate the effectiveness of our deep learning framework and the value of using image data for cycling stress assessment in the absence of high-quality road geometry and motor traffic data. |
32. | Implementing artificial intelligence in Canadian primary care: Barriers and strategies identified through a national deliberative dialogue Journal Article K. Darcel, T. Upshaw, A. Craig-Neil, J. Macklin, C. Steele Gray, T. C. Y. Chan, J. Gibson, A. Pinto In: PLOS One, vol. 18, no. 2, pp. e0281733, 2023. @article{ChanTCY.J0106, Background: With large volumes of longitudinal data in electronic medical records from diverse patients, primary care is primed for disruption by artificial intelligence (AI) technology. With AI applications in primary care still at an early stage in Canada and most countries, there is a unique opportunity to engage key stakeholders in exploring how AI would be used and what implementation would look like. Objective: To identify the barriers that patients, providers, and health leaders perceive in relation to implementing AI in primary care and strategies to overcome them. Design: 12 virtual deliberative dialogues. Dialogue data were thematically analyzed using a combination of rapid ethnographic assessment and interpretive description techniques. Setting: Virtual sessions Participants: Participants from eight provinces in Canada, including 22 primary care service users, 21 interprofessional providers, and 5 health system leaders Results: The barriers that emerged from the deliberative dialogue sessions were grouped into four themes: (1) system and data readiness, (2) the potential for bias and inequity, (3) the regulation of AI and big data, and (4) the importance of people as technology enablers. Strategies to overcome the barriers in each of these themes were highlighted, where participatory co-design and iterative implementation were voiced most strongly by participants. Limitations: Only five health system leaders were included in the study and no self-identifying Indigenous people. This is a limitation as both groups may have provided unique perspectives to the study objective. Conclusions: These findings provide insight into the barriers and facilitators associated with implementing AI in primary care settings from different perspectives. This will be vital as decisions regarding the future of AI in this space is shaped. |
31. | 3D dose prediction for Gamma Knife radiosurgery using deep learning and data modification Journal Article B. Zhang, A. Babier, T. C. Y. Chan, M. Ruschin In: Physica Medica, vol. 106, pp. 102533, 2023. @article{ChanTCY.J0104, Purpose: To develop a machine learning-based, 3D dose prediction methodology for Gamma Knife (GK) radiosurgery. The methodology accounts for cases involving targets of any number, size, and shape. Methods: Data from 322 GK treatment plans was modified by isolating and cropping the contoured MRI and clinical dose distributions based on tumor location, then scaling the resulting tumor spaces to a standard size. An accompanying 3D tensor was created for each instance to account for tumor size. The modified dataset for 272 patients was used to train both a generative adversarial network (GAN-GK) and a 3D U-Net model (U-Net-GK). Unmodified data was used to train equivalent baseline models. All models were used to predict the dose distribution of 50 out-of-sample patients. Prediction accuracy was evaluated using gamma, with criteria of 4%/2mm, 3%/3mm, 3%/1mm and 1%/1mm. Prediction quality was assessed using coverage, selectivity, and conformity indices. Results: The predictions resulting from GAN-GK and U-Net-GK were similar to their clinical counterparts, with average gamma (4%/2mm) passing rates of 84.9 ± 15.3% and 83.1 ± 17.2%, respectively. In contrast, the gamma passing rate of baseline models were significantly worse than their respective GK-specific models (p < 0.001) at all criterion levels. The quality of GK-specific predictions was also similar to that of clinical plans. Conclusion: Deep learning models can use GK-specific data modification to predict 3D dose distributions for GKRS plans with a large range in size, shape, or number of targets. Standard deep learning models applied to unmodified GK data generated poorer predictions. |
30. | Priorities for artificial intelligence in primary care: A Canadian deliberative dialogue with patients, providers, and health system leaders Journal Article T. L. Upshaw, A. Craig-Neil, J. Macklin, C. Steele Gray, T. C. Y. Chan, J. Gibson, A. D. Pinto In: Journal of the American Board of Family Medicine, vol. 36, no. 2, pp. 210-220, 2023. @article{ChanTCY.J104, BACKGROUND: Artificial intelligence (AI) implementation in primary care is limited. Those set to be most impacted by AI technology in this setting should guide the application. We organized a national deliberative dialogue with primary care stakeholders from across Canada to explore how they thought AI should be applied in primary care. METHODS: We conducted 12 virtual deliberative dialogues with 22 patients, 21 interprofessional primary care providers, and 5 health system leaders from eight Canadian provinces to identify shared priorities for applying AI in primary care. Dialogue data were thematically analyzed using interpretive description approaches. RESULTS: Participants thought that AI should be first applied in primary care to documentation, practice operations, and triage tasks, in hopes of improving efficiency while maintaining person-centred delivery, relationships, and access. They viewed complex AI-driven clinical decision support and proactive care tools as impactful but recognized potential risks to patient safety, given numerous external limitations. Appropriate training and implementation support were the most important external enablers of safe, effective, and patient-centred use of AI in primary care settings. INTERPRETATION: Our findings offer an agenda for the future application of AI in primary care that is grounded in the shared values of patients and providers. We propose that, from conception, AI developers should work with primary care stakeholders as co-design partners, developing tools that respond to their shared priorities. |
29. | Introducing and integrating machine learning in an operations research curriculum: An application-driven course Journal Article J. J. Boutilier, T. C. Y. Chan In: INFORMS Transactions on Education, vol. 23, no. 2, pp. 64-83, 2023. @article{ChanTCY.J0103, Artificial intelligence (AI) and operations research (OR) have long been intertwined because of their synergistic relationship. Given the increasing popularity of AI and machine learning in particular, we face growing demand for educational offerings in this area from our students. This paper describes two courses that introduce machine learning concepts to undergraduate, predominantly industrial engineering and operations research students. Instead of taking a methods-first approach, these courses use real-world applications to motivate, introduce, and explore these machine learning techniques and highlight meaningful overlap with operations research. Significant hands-on coding experience is used to build student proficiency with the techniques. Student feedback indicates that these courses have greatly increased student interest in machine learning and appreciation of the real-world impact that analytics can have and helped students develop practical skills that they can apply. We believe that similar application-driven courses that connect machine learning and operations research would be valuable additions to undergraduate OR curricula broadly. |
28. | A machine learning approach to solving large bilevel and stochastic programs: Application to cycling network design Miscellaneous T. C. Y. Chan, B. Lin, S. Saxe 2022. @misc{ChanTCY.Pre017, We present a novel machine learning-based approach to solving bilevel programs that involve a large number of independent followers, which as a special case include two-stage stochastic programming. We propose an optimization model that explicitly considers a sampled subset of followers and exploits a machine learning model to estimate the objective values of unsampled followers. Unlike existing approaches, we embed machine learning model training into the optimization problem, which allows us to employ general follower features that can not be represented using leader decisions. We prove bounds on the optimality gap of the generated leader decision as measured by the original objective function that considers the full follower set. We then develop follower sampling algorithms to tighten the bounds and a representation learning approach to learn follower features, which can be used as inputs to the embedded machine learning model. Using synthetic instances of a cycling network design problem, we compare the computational performance of our approach versus baseline methods. Our approach provides more accurate predictions for follower objective values, and more importantly, generates leader decisions of higher quality. Finally, we perform a real-world case study on cycling infrastructure planning, where we apply our approach to solve a network design problem with over one million followers. Our approach presents favorable performance compared to the current cycling network expansion practices. |
27. | Sparse flexible design: A machine learning approach Journal Article T. C. Y. Chan, D. Letourneau, B. Potter In: Flexible Services and Manufacturing Journal, vol. 34, pp. 1066-1116, 2022. @article{ChanTCY.J091, For a general production network, state-of-the-art methods for constructing sparse flexible designs are heuristic in nature, typically computing a proxy for the quality of unseen networks and using that estimate in a greedy manner to modify a current design. This paper develops two machine learning-based approaches to constructing sparse flexible designs that leverage a neural network to accurately and quickly predict the performance of large numbers of candidate designs. We demonstrate that our heuristics are competitive with existing approaches and produce high-quality solutions for both balanced and unbalanced networks. Finally, we introduce a novel application of process flexibility in healthcare operations to demonstrate the effectiveness of our approach in a large numerical case study. We study the flexibility of linear accelerators that deliver radiation to treat various types of cancer. We demonstrate how clinical constraints can be easily absorbed into the machine learning subroutine and how our sparse flexible treatment networks meet or beat the performance of those designed by state-of-the-art methods. |
26. | Predicting protein and fat content in human donor milk using machine learning Journal Article R. K. Wong, M. A. Pitino, R. Mahmood, I. Y. Zhu, D. Stone, D. L. O'Connor, S. Unger, T. C. Y. Chan In: Journal of Nutrition, vol. 151, pp. 2075-2083, 2021. @article{ChanTCY.J084, Background: Donor milk is the standard of care for hospitalized very low birth weight (VLBW) infants when mother’s milk is unavailable; however, growth of donor milk–fed infants is frequently suboptimal. Variability in nutrient composition of donated milk complicates the production of a uniform pooled product and, subsequently, the provision of adequate nutrition to promote optimal growth and development of VLBW infants. We reasoned a machine learning approach to construct batches using characteristics of the milk donation might be an effective strategy in reducing the variability in donor milk product composition. Objective: The objective of this study was to identify whether machine learning models can accurately predict donor milk macronutrient content. We focused on predicting fat and protein, given their well-established importance in VLBW infant growth outcomes. Methods: Samples of donor milk, consisting of 272 individual donations and 61 pool samples, were collected from the Rogers Hixon Ontario Human Milk Bank and analyzed for macronutrient content. Four different machine learning models were constructed using independent variable groups associated with donations, donors, and donor-pumping practices. A baseline model was established using lactation stage and infant gestational status. Predictions were made for individual donations and resultant pools. Results: Machine learning models predicted protein of individual donations and pools with a mean absolute error (MAE) of 0.16 g/dL and 0.10 g/dL, respectively. Individual donation and pooled fat predictions had an MAE of 0.91 g/dL and 0.42 g/dL, respectively. At both the individual donation and pool levels, protein predictions were significantly more accurate than baseline, whereas fat predictions were competitive with baseline. Conclusions: Machine learning models can provide accurate predictions of macronutrient content in donor milk. The macronutrient content of pooled milk had a lower prediction error, reinforcing the value of pooling practices. Future research should examine how macronutrient content predictions can be used to facilitate milk bank pooling strategies. |
25. | Machine learning-based dispatch of drone-delivered defibrillators for out-of-hospital cardiac arrest Journal Article J. Chu, K. H. B. Leung, P. Snobelen, G. Nevils, I. R. Drennan, S. Cheskes, T. C. Y. Chan In: Resuscitation, vol. 162, pp. 120-127, 2021. @article{ChanTCY.J083, Background: Drone-delivered defibrillators have the potential to significantly reduce response time for out-of-hospital cardiac arrest (OHCA). However, optimal policies for the dispatch of such drones are not yet known. We sought to develop dispatch rules for a network of defibrillator-carrying drones. Methods: We identified all suspected OHCAs in Peel Region, Ontario, Canada from Jan. 2015 to Dec. 2019. We developed drone dispatch rules based on the difference between a predicted ambulance response time to a calculated drone response time for each OHCA. Ambulance response times were predicted using linear regression and neural network models, while drone response times were calculated using drone specifications from recent pilot studies and the literature. We evaluated the dispatch rules based on response time performance and dispatch decisions, comparing them to two baseline policies of never dispatching and always dispatching drones. Results: A total of 3573 suspected OHCAs were included in the study with median and mean historical ambulance response times of 5.8 and 6.2 min. All machine learning-based dispatch rules significantly reduced the median response time to 3.9min and mean response time to 4.14.2min (all P < 0.001) and were non-inferior to universally dispatching drones (all P < 0.001) while reducing the number of drone flights by up to 30%. Dispatch rules with more drone flights achieved higher sensitivity but lower specificity and accuracy. Conclusion: Machine learning-based dispatch rules for drone-delivered defibrillators can achieve similar response time reductions as universal drone dispatch while substantially reducing the number of trips. |
24. | Peripheral inflammatory biomarkers define biotypes of bipolar depression Journal Article Y. Lee, R. B. Mansur, E. Brietzke, D. Kapogiannis, F. Delgado-Peraza, J. J. Boutilier, T. C. Y. Chan, N. E. Carmona, J. D. Rosenblat, J. G. Lee, V. Maletic, M. Vinberg, T. Suppes, B. I. Goldstein, A. V. Ravindran, V. H. Taylor, S. Chawla, C. Nogueras-Ortiz, V. E. Cosgrove, N. E. Kramer, R. Ho, C. A. Raison, R. S. McIntyre In: Molecular Psychiatry, vol. 26, pp. 3395-3406, 2021. @article{ChanTCY.J080, We identified biologically relevant moderators of response to tumor necrosis factor (TNF)-α inhibitor, infliximab, among 60 individuals with bipolar depression. Data were derived from a 12-week, randomized, placebo-controlled clinical trial secondarily evaluating the efficacy of infliximab on a measure of anhedonia (i.e., Snaith–Hamilton Pleasure Scale). Three inflammatory biotypes were derived from peripheral cytokine measurements using an iterative, machine learning-based approach. Infliximab-randomized participants classified as biotype 3 exhibited lower baseline concentrations of pro- and anti-inflammatory cytokines and soluble TNF receptor-1 and reported greater pro-hedonic improvements, relative to those classified as biotype 1 or 2. Pretreatment biotypes also moderated changes in neuroinflammatory substrates relevant to infliximab’s hypothesized mechanism of action. Neuronal origin-enriched extracellular vesicle (NEV) protein concentrations were reduced to two factors using principal axis factoring: phosphorylated nuclear factorκB (p-NFκB), Fas-associated death domain (p-FADD), and IκB kinase (p-IKKα/β) and TNF receptor-1 (TNFR1) comprised factor “NEV1,” whereas phosphorylated insulin receptor substrate-1 (p-IRS1), p38 mitogen-activated protein kinase (p-p38), and c-Jun N-terminal kinase (p-JNK) constituted “NEV2”. Among infliximab-randomized subjects classified as biotype 3, NEV1 scores were decreased at weeks 2 and 6 and increased at week 12, relative to baseline, and NEV2 scores increased over time. Decreases in NEV1 scores and increases in NEV2 scores were associated with greater reductions in anhedonic symptoms in our classification and regression tree model (r² = 0.22, RMSE = 0.08). Our findings provide preliminary evidence supporting the hypothesis that the pro-hedonic effects of infliximab require modulation of multiple TNF-α signaling pathways, including NF-κB, IRS1, and MAPK. |
23. | OpenKBP: The Open-Access Knowledge-Based Planning Grand Challenge and Dataset Journal Article A. Babier, B. Zhang, R. Mahmood, K. L. Moore, T. G. Purdie, A. L. McNiven, T. C. Y. Chan In: Medical Physics, vol. 48, no. 9, pp. 5549-5561, 2021. @article{ChanTCY.J078, Purpose: To advance fair and consistent comparisons of dose prediction methods for knowledge-based planning (KBP) in radiation therapy research. Methods: We hosted OpenKBP, a 2020 AAPM Grand Challenge, and challenged participants to develop the best method for predicting the dose of contoured computed tomography (CT) images. The models were evaluated according to two separate scores: (a) dose score, which evaluates the full three-dimensional (3D) dose distributions, and (b) dose-volume histogram (DVH) score, which evaluates a set DVH metrics. We used these scores to quantify the quality of the models based on their out-of-sample predictions. To develop and test their models, participants were given the data of 340 patients who were treated for head-and-neck cancer with radiation therapy. The data were partitioned into training (n = 200), validation (n = 40), and testing (n = 100) datasets. All participants performed training and validation with the corresponding datasets during the first (validation) phase of the Challenge. In the second (testing) phase, the participants used their model on the testing data to quantify the out-of-sample performance, which was hidden from participants and used to determine the final competition ranking. Participants also responded to a survey to summarize their models. Results: The Challenge attracted 195 participants from 28 countries, and 73 of those participants formed 44 teams in the validation phase, which received a total of 1750 submissions. The testing phase garnered submissions from 28 of those teams, which represents 28 unique prediction methods. On average, over the course of the validation phase, participants improved the dose and DVH scores of their models by a factor of 2.7 and 5.7, respectively. In the testing phase one model achieved the best dose score (2.429) and DVH score (1.478), which were both significantly better than the dose score (2.564) and the DVH score (1.529) that was achieved by the runner-up models. Lastly, many of the top performing teams reported that they used generalizable techniques (e.g., ensembles) to achieve higher performance than their competition. Conclusion: OpenKBP is the first competition for knowledge-based planning research. The Challenge helped launch the first platform that enables researchers to compare KBP prediction methods fairly and consistently using a large open-source dataset and standardized metrics. OpenKBP has also democratized KBP research by making it accessible to everyone, which should help accelerate the progress of KBP research. The OpenKBP datasets are available publicly to help benchmark future KBP research. |
22. | Risk stratification for early detection of diabetes and hypertension in resource-limited settings: Machine learning analysis Journal Article J. J. Boutilier, T. C. Y. Chan, M. Ranjan, S. Deo In: Journal of Medical Internet Research, vol. 23, no. Article No. e20123, 2021. @article{ChanTCY.J077, Background: The impending scale up of noncommunicable disease screening programs in low- and middle-income countries coupled with limited health resources require that such programs be as accurate as possible at identifying patients at high risk. Objective: The aim of this study was to develop machine learning–based risk stratification algorithms for diabetes and hypertension that are tailored for the at-risk population served by community-based screening programs in low-resource settings. Methods: We trained and tested our models by using data from 2278 patients collected by community health workers through door-to-door and camp-based screenings in the urban slums of Hyderabad, India between July 14, 2015 and April 21, 2018. We determined the best models for predicting short-term (2-month) risk of diabetes and hypertension (a model for diabetes and a model for hypertension) and compared these models to previously developed risk scores from the United States and the United Kingdom by using prediction accuracy as characterized by the area under the receiver operating characteristic curve (AUC) and the number of false negatives. Results: We found that models based on random forest had the highest prediction accuracy for both diseases and were able to outperform the US and UK risk scores in terms of AUC by 35.5% for diabetes (improvement of 0.239 from 0.671 to 0.910) and 13.5% for hypertension (improvement of 0.094 from 0.698 to 0.792). For a fixed screening specificity of 0.9, the random forest model was able to reduce the expected number of false negatives by 620 patients per 1000 screenings for diabetes and 220 patients per 1000 screenings for hypertension. This improvement reduces the cost of incorrect risk stratification by US $1.99 (or 35%) per screening for diabetes and US $1.60 (or 21%) per screening for hypertension. Conclusions: In the next decade, health systems in many countries are planning to spend significant resources on noncommunicable disease screening programs and our study demonstrates that machine learning models can be leveraged by these programs to effectively utilize limited resources by improving risk stratification. |
21. | Predicting depression from hearing loss using machine learning Journal Article M. G. Crowson, K. H. Franck, L. C. Rosella, T. C. Y. Chan In: Ear and Hearing, vol. 42, pp. 982-989, 2021. @article{ChanTCY.J076, Objectives: Hearing loss is the most common sensory loss in humans and carries an enhanced risk of depression. No prior studies have attempted a contemporary machine learning approach to predict depression using subjective and objective hearing loss predictors. The objective was to deploy supervised machine learning to predict scores on a validated depression scale using subjective and objective audiometric variables and other health determinant predictors. Design: A large predictor set of health determinants from the National Health and Nutrition Examination Survey 2015–2016 database was used to predict adults’ scores on a validated instrument to screen for the presence and severity of depression (Patient Health Questionnaire-9 [PHQ-9]). After model training, the relative influence of individual predictors on depression scores was stratified and analyzed. Model prediction performance was determined by prediction error metrics. Results: The test set mean absolute error was 3.03 (95% confidence interval: 2.91 to 3.14) and 2.55 (95% confidence interval: 2.48 to 2.62) on datasets with audiology-only predictors and all predictors, respectively, on the PHQ-9’s 27-point scale. Participants’ self-reported frustration when talking to members of family or friends due to hearing loss was the fifth-most influential of all predictors. Of the top 10 most influential audiometric predictors, five were related to social contexts, two for significant noise exposure, two objective audiometric parameters, and one presence of bothersome tinnitus. Conclusions: Machine learning algorithms can accurately predict PHQ-9 depression scale scores from National Health and Nutrition Examination Survey data. The most influential audiometric predictors of higher scores on a validated depression scale were social dynamics of hearing loss and not objective audiometric testing. Such models could be useful in predicting depression scale scores at the point-of-care in conjunction with a standard audiologic assessment. |
20. | Sampling from the complement of a polyhedron: An MCMC algorithm for data augmentation Journal Article T. C. Y. Chan, A. Diamant, R. Mahmood In: Operations Research Letters, vol. 48, pp. 744–751, 2020. @article{ChanTCY.J072, We present an MCMC algorithm for sampling from the complement of a polyhedron. Our approach is based on the Shake-and-bake algorithm for sampling from the boundary of a set and provably covers the complement. We use this algorithm for data augmentation in a machine learning task of classifying a hidden feasible set in a data-driven optimization pipeline. Numerical results on simulated and MIPLIB instances demonstrate that our algorithm, along with a supervised learning technique, outperforms conventional unsupervised baselines. |
19. | AutoAudio: Deep learning for automatic audiogram interpretation Journal Article M. G. Crowson, J. W. Lee, A. Hamour, R. Mahmood, V. Lin, D. L. Tucci, T. C. Y. Chan In: Journal of Medical Systems, vol. 44 (Article No. 163), 2020. @article{ChanTCY.J070, Hearing loss is the leading human sensory system loss, and one of the leading causes for years lived with disability with significant effects on quality of life, social isolation, and overall health. Coupled with a forecast of increased hearing loss burden worldwide, national and international health organizations have urgently recommended that access to hearing evaluation be expanded to meet demand. The objective of this study was to develop ‘AutoAudio’ – a novel deep learning proof-of-concept model that accurately and quickly interprets diagnostic audiograms. Adult audiogram reports representing normal, conductive, mixed and sensorineural morphologies were used to train different neural network architectures. Image augmentation techniques were used to increase the training image set size. Classification accuracy on a separate test set was used to assess model performance. The architecture with the highest out-of-training set accuracy was ResNet-101 at 97.5%. Neural network training time varied between 2 to 7 h depending on the depth of the neural network architecture. Each neural network architecture produced misclassifications that arose from failures of the model to correctly label the audiogram with the appropriate hearing loss type. The most commonly misclassified hearing loss type were mixed losses. Re-engineering the process of hearing testing with a machine learning innovation may help enhance access to the growing worldwide population that is expected to require audiologist services. Our results suggest that deep learning may be a transformative technology that enables automatic and accurate audiogram interpretation. |
18. | Machine learning as a catalyst for value-based health care Journal Article M. G. Crowson, T. C. Y. Chan In: Journal of Medical Systems, vol. 44 (Article No. 139), 2020. @article{ChanTCY.J068, |
17. | Machine learning for pattern detection in cochlear implant FDA adverse event reports Journal Article M. G. Crowson, A. Hamour, V. Lin, J. M. Chen, T. C. Y. Chan In: Cochlear Implants International, vol. 21, pp. 313-322, 2020. @article{ChanTCY.J067, Importance: Medical device performance and safety databases can be analyzed for patterns and novel opportunities for improving patient safety and/or device design. Objective: The objective of this analysis was to use supervised machine learning to explore patterns in reported adverse events involving cochlear implants. Design: Adverse event reports for the top three CI manufacturers were acquired for the analysis. Four supervised machine learning algorithms were used to predict which adverse event description pattern corresponded with a specific cochlear implant manufacturer and adverse event type. Setting: U.S. government public database. Participants: Adult and pediatric cochlear patients. Exposure: Surgical placement of a cochlear implant. Main Outcome Measure: Classification prediction accuracy (% correct predictions). Results: Most adverse events involved patient injury (n=16,736), followed by device malfunction (n= 10,760), and death (n = 16). The random forest, linear SVC, naïve Bayes and logistic algorithms were able to predict the specific CI manufacturer based on the adverse event narrative with an average accuracy of 74.8%, 86.0%, 88.5% and 88.6%, respectively. Conclusions & relevance: Using supervised machine learning algorithms, our classification models were able to predict the CI manufacturer and event type with high accuracy based on patterns in adverse event text descriptions. |
16. | The importance of evaluating the complete automated knowledge-based planning pipeline Journal Article A. Babier, R. Mahmood, A. L. McNiven, A. Diamant, T. C. Y. Chan In: Physica Medica, vol. 72, pp. 73-79, 2020. @article{ChanTCY.J064, We determine how prediction methods combine with optimization methods in two-stage knowledge-based planning (KBP) pipelines to produce radiation therapy treatment plans. We trained two dose prediction methods, a generative adversarial network (GAN) and a random forest (RF) with the same 130 treatment plans. The models were applied to 87 out-of-sample patients to create two sets of predicted dose distributions that were used as input to two optimization models. The first optimization model, inverse planning (IP), estimates weights for dose-objectives from a predicted dose distribution and generates new plans using conventional inverse planning. The second optimization model, dose mimicking (DM), minimizes the sum of one-sided quadratic penalties between the predictions and the generated plans using several dose-objectives. Altogether, four KBP pipelines (GAN-IP, GAN-DM, RF-IP, and RF-DM) were constructed and benchmarked against the corresponding clinical plans using clinical criteria; the error of both prediction methods was also evaluated. The best performing plans were GAN-IP plans, which satisfied the same criteria as their corresponding clinical plans (78%) more often than any other KBP pipeline. However, GAN did not necessarily provide the best prediction for the second-stage optimization models. Specifically, both the RF-IP and RF-DM plans satisfied the same criteria as the clinical plans 25% and 15% more often than GAN-DM plans (the worst performing plans), respectively. GAN predictions also had a higher mean absolute error (3.9 Gy) than those from RF (3.6 Gy). We find that state-of-the-art prediction methods when paired with different optimization algorithms, produce treatment plans with considerable variation in quality. |
15. | Predicting post-operative cochlear implant performance using supervised machine learning Journal Article M. G. Crowson, P. Dixon, J. W. Lee, D. Shipp, T. Le, V. Lin, J. Chen, T. C. Y. Chan In: Otology & Neurotology, vol. 41, pp. e1013-e1023, 2020. @article{ChanTCY.J062, Objectives: To predict post-operative cochlear implant performance with heterogeneous text and numerical variables using supervised machine learning techniques. Study Design: A supervised machine learning approach comprising neural networks and decision tree-based ensemble algorithms were used to predict 1-year post-operative cochlear implant performance based on retrospective data. Setting: Tertiary referral center. Patients: One thousand six hundred four adults who received one cochlear implant from 1989 to 2019. Two hundred eighty two text and numerical objective demographic, audiometric, and patient-reported outcome survey instrument variables were included. Outcome Measures: Outcomes for post-operative cochlear implant performance were discrete Hearing in Noise Test (HINT; %) performance and binned HINT performance classification (‘‘High,’’ ‘‘Mid,’’ and ‘‘Low’’ performers). Algorithm performance was assessed using hold-out validation datasets and were compared using root mean square error (RMSE) in the units of the target variable and classification accuracy. Results: The neural network 1-year HINT prediction RMSE and classification accuracy were 0.57 and 95.4%, respectively, with only numerical variable inputs. Using both text and numerical variables, neural networks predicted post-operative HINT with a RMSE of 25.0%, and classification accuracy of 73.3%. When applied to numerical variables only, the XGBoost algorithm produced a 1-year HINT score prediction performance RMSE of 25.3%. We identified over 20 influential variables including preoperative sentence-test performance, age at surgery, as well as specific tinnitus handicap inventory (THI), Short Form 36 (SF-36), and health utilities index (HUI) question responses as the highest influencers of post-operative HINT. Conclusion: Our results suggest that supervised machine learning can predict post-operative cochlear implant performance and identify preoperative factors that significantly influence that performance. These algorithms can help improve the understanding of the diverse factors that impact functional performance from heterogeneous data sources. |
14. | Ambulance Emergency Response Optimization in developing countries Journal Article J. J. Boutilier, T. C. Y. Chan In: Operations Research, vol. 68, pp. 1315-1334, 2020. @article{ChanTCY.J060, The lack of emergency medical transportation is viewed as the main barrier to the access and availability of emergency medical care in low- and middle-income countries (LMICs). In this paper, we present a robust optimization approach to optimize both the location and routing of emergency response vehicles, accounting for uncertainty in travel times and spatial demand characteristic of LMICs. We traveled to Dhaka, Bangladesh, the sixth largest and third most densely populated city in the world, to conduct field research resulting in the collection of two unique data sets that inform our approach. These data are leveraged to estimate demand for emergency medical services in an LMIC setting and to predict the travel time between any two locations in the road network for different times of day and days of the week. We combine our prediction-optimization framework with a simulation model and real data to provide an in-depth investigation into three policy- related questions. First, we demonstrate that outpost locations optimized for weekday rush hour lead to good performance for all times of day and days of the week. Second, we find that the performance of the current system could be replicated using one third of the current outpost locations and one half of the current number of ambulances. Finally, we show that a fleet of small ambulances has the potential to significantly outperform tra- ditional ambulance vans. In particular, they are able to capture approximately three times more demand while reducing the median average response time by roughly 10%–18% over the entire week and 24%–35% during rush hour because of increased routing flexibility offered by more nimble vehicles on a larger road network. Our results provide practical insights for emergency response optimization that can be leveraged by hospital-based and private ambulance providers in Dhaka and other urban centers in developing countries. |
13. | Knowledge-based automated planning with three-dimensional generative adversarial networks Journal Article A. Babier, R. Mahmood, A. L. McNiven, A. Diamant, T. C. Y. Chan In: Medical Physics, vol. 47, pp. 297-306, 2020. @article{ChanTCY.J059, Purpose: To develop a knowledge-based automated planning pipeline that generates treatment plans without feature engineering, using deep neural network architectures for predicting three-dimensional (3D) dose. Methods: Our knowledge-based automated planning (KBAP) pipeline consisted of a knowledge-based planning (KBP) method that predicts dose for a contoured computed tomography (CT) image followed by two optimization models that learn objective function weights and generate fluence-based plans, respectively. We developed a novel generative adversarial network (GAN)-based KBP approach, a 3D GAN model, which predicts dose for the full 3D CT image at once and accounts for correlations between adjacent CT slices. Baseline comparisons were made against two state-of-the-art deep learning–based KBP methods from the literature. We also developed an additional benchmark, a two-dimensional (2D) GAN model which predicts dose to each axial slice independently. For all models, we investigated the impact of multiplicatively scaling the predictions before optimization, such that the predicted dose distributions achieved all target clinical criteria. Each KBP model was trained on 130 previously delivered oropharyngeal treatment plans. Performance was tested on 87 out-of-sample previously delivered treatment plans. All KBAP plans were evaluated using clinical planning criteria and compared to their corresponding clinical plans. KBP prediction quality was assessed using dose-volume histogram (DVH) differences from the corresponding clinical plans. Results: The best performing KBAP plans were generated using predictions from the 3D GAN model that were multiplicatively scaled. These plans satisfied 77% of all clinical criteria, compared to the clinical plans, which satisfied 67% of all criteria. In general, multiplicatively scaling predictions prior to optimization increased the fraction of clinical criteria satisfaction by 11% relative to the plans generated with nonscaled predictions. Additionally, these KBAP plans satisfied the same criteria as the clinical plans 84% and 8% more frequently as compared to the two benchmark methods, respectively. Conclusions: We developed the first knowledge-based automated planning framework using a 3D generative adversarial network for prediction. Our results, based on 217 oropharyngeal cancer treatment plans, demonstrated superior performance in satisfying clinical criteria and generated more realistic plans as compared to the previous state-of-the-art approaches. |
12. | Predicting plays in the National Football League Journal Article C. Fernandes, R. Yakubov, Y. Li, A. Prasad, T. C. Y. Chan In: Journal of Sports Analytics, vol. 6, pp. 35-43, 2020. @article{ChanTCY.J058, This paper aims to develop an interpretable machine learning model to predict plays (pass versus rush) in the National Football League that will be useful for players and coaches in real time. Using data from the 2013–2014 to 2016– 2017 NFL regular seasons, which included 1034 games and 130,344 pass/rush plays, we first develop and compare several machine learning models to determine the maximum possible prediction accuracy. The best performing model, a neural network, achieves a prediction accuracy of 75.3%, which is competitive with the state-of-the-art methods applied to other datasets. Then, we search over a family of simple decision tree models to identify one that captures 86% of the prediction accuracy of the neural network yet can be easily memorized and implemented in an actual game. We extend the analysis to building decision tree models tailored for each of the 32 NFL teams, obtaining accuracies ranging from 64.7% to 82.5%. Overall, our decision tree models can be a useful tool for coaches and players to improve their chances of stopping an offensive play. |
11. | A contemporary review of machine learning in otolaryngology-head & neck surgery Journal Article M. G. Crowson, J. Ranisau, A. Eskander, A. Babier, B. Xu, R. R. Kahmke, J. M. Chen, T. C. Y. Chan In: Laryngoscope, vol. 130, pp. 45-51, 2020. @article{ChanTCY.J056, One of the key challenges with big data is leveraging the complex network of information to yield useful clinical insights. The confluence of massive amounts of health data and a desire to make inferences and insights on these data has produced a substantial amount of interest in machine-learning analytic methods. There has been a drastic increase in the otolaryngology literature volume describing novel applications of machine learning within the past 5 years. In this timely contemporary review, we provide an overview of popular machine-learning techniques, and review recent machine-learning applications in otolaryngology–head and neck surgery including neurotology, head and neck oncology, laryngology, and rhinology. Investigators have realized significant success in validated models with model sensitivities and specificities approaching 100%. Challenges remain in the implementation of machine-learning algorithms. This may be in part the unfamiliarity of these techniques to clinician leaders on the front lines of patient care. Spreading awareness and confidence in machine learning will follow with further validation and proof-of-value analyses that demonstrate model performance superiority over established methods. We are poised to see a greater influx of machine-learning applications to clinical problems in otolaryngology–head and neck surgery, and it is prudent for providers to understand the potential benefits and limitations of these technologies. |
10. | Machine learning & cochlear implantation – A structured review of opportunities and challenges Journal Article M. G. Crowson, V. Lin, J. M. Chen, T. C. Y. Chan In: Otology & Neurotology, vol. 41, pp. e36-e45, 2019. @article{ChanTCY.J054, Objective: The use of machine learning technology to automate intellectual processes and boost clinical process efficiency in medicine has exploded in the past 5 years. Machine learning excels in automating pattern recognition and in adapting learned representations to new settings. Moreover, machine learning techniques have the advantage of incorporating complexity and are free from many of the limitations of traditional deterministic approaches. Cochlear implants (CI) are a unique fit for machine learning techniques given the need for optimization of signal processing to fit complex environmental scenarios and individual patients' CI MAPping. However, there are many other opportunities where machine learning may assist in CI beyond signal processing. The objective of this review was to synthesize past applications of machine learning technologies for pediatric and adult CI and describe novel opportunities for research and development. Data sources: The PubMed/MEDLINE, EMBASE, Scopus, and ISI Web of Knowledge databases were mined using a directed search strategy to identify the nexus between CI and artificial intelligence/machine learning literature. Study selection: Non-English language articles, articles without an available abstract or full-text, and nonrelevant articles were manually appraised and excluded. Included articles were evaluated for specific machine learning methodologies, content, and application success. Data synthesis: The database search identified 298 articles. Two hundred fifty-nine articles (86.9%) were excluded based on the available abstract/full-text, language, and relevance. The remaining 39 articles were included in the review analysis. There was a marked increase in year-over-year publications from 2013 to 2018. Applications of machine learning technologies involved speech/signal processing optimization (17; 43.6% of articles), automated evoked potential measurement (6; 15.4%), postoperative performance/efficacy prediction (5; 12.8%), and surgical anatomy location prediction (3; 7.7%), and 2 (5.1%) in each of robotics, electrode placement performance, and biomaterials performance. Conclusion: The relationship between CI and artificial intelligence is strengthening with a recent increase in publications reporting successful applications. Considerable effort has been directed toward augmenting signal processing and automating postoperative MAPping using machine learning algorithms. Other promising applications include augmenting CI surgery mechanics and personalized medicine approaches for boosting CI patient performance. Future opportunities include addressing scalability and the research and clinical communities' acceptance of machine learning algorithms as effective techniques. |
9. | The importance of evaluating the complete knowledge-based automated planning pipeline Proceedings Article A. Babier, R. Mahmood, A. Diamant, A. McNiven, T. C. Y. Chan In: Proceedings of the International Conference on the use of Computers in Radiation Therapy, 2019. @inproceedings{ChanTCY.Oth007c, |
8. | Applications of machine learning algorithms to predict therapeutic outcomes in depression: A meta-analysis and systematic review Journal Article Y. Lee, R.-M. Ragguett, R. B. Mansur, J. J. Boutilier, J. D. Rosenblat, A. Trevizol, E. Brietzke, K. Lin, Z. Pan, M. Subramaniapillai, T. C. Y. Chan, D. Fus, C. Park, N. Musial, H. Zuckerman, V. C.-H. Chen, R. Ho, C. Rong, R. S. McIntyre In: Journal of Affective Disorders, vol. 241, pp. 519-532, 2018. @article{ChanTCY.J048, Background: No previous study has comprehensively reviewed the application of machine learning algorithms in mood disorders populations. Herein, we qualitatively and quantitatively evaluate previous studies of machine learning-devised models that predict therapeutic outcomes in mood disorders populations. Methods: We searched Ovid MEDLINE/PubMed from inception to February 8, 2018 for relevant studies that included adults with bipolar or unipolar depression; assessed therapeutic outcomes with a pharmacological, neuromodulatory, or manual-based psychotherapeutic intervention for depression; applied a machine learning algorithm; and reported predictors of therapeutic response. A random-effects meta-analysis of proportions and meta-regression analyses were conducted. Results: We identified 639 records: 75 full-text publications were assessed for eligibility; 26 studies (n = 17, 499) and 20 studies (n = 6325) were included in qualitative and quantitative review, respectively. Classification algorithms were able to predict therapeutic outcomes with an overall accuracy of 0.82 (95% confidence interval [CI] of [0.77, 0.87]). Pooled estimates of classification accuracy were significantly greater (p < 0.01) in models informed by multiple data types (e.g., composite of phenomenological patient features and neuroimaging or peripheral gene expression data; pooled proportion [95% CI] = 0.93[0.86, 0.97]) when compared to models with lower-dimension data types (pooled proportion = 0.68[0.62, 0.74] to 0.85[0.81, 0.88]). Limitations: Most studies were retrospective; differences in machine learning algorithms and their implementation (e.g., cross-validation, hyperparameter tuning); cannot infer importance of individual variables fed into learning algorithm. Conclusions: Machine learning algorithms provide a powerful conceptual and analytic framework capable of integrating multiple data types and sources. An integrative approach may more effectively model neurobiological components as functional modules of pathophysiology embedded within the complex, social dynamics that influence the phenomenology of mental disorders. |
7. | Knowledge-based automated planning for oropharyngeal cancer Journal Article A. Babier, J. J. Boutilier, A. L. McNiven, T. C. Y. Chan In: Medical Physics, vol. 45, pp. 2875-2883, 2018. @article{ChanTCY.J043, Purpose: The purpose of this study was to automatically generate radiation therapy plans for oropharynx patients by combining knowledge-based planning (KBP) predictions with an inverse optimization (IO) pipeline. Methods: We developed two KBP approaches, the bagging query (BQ) method and the generalized principal component analysis-based (gPCA) method, to predict achievable dose–volume histograms (DVHs). These approaches generalize existing methods by predicting physically feasible organ-at-risk (OAR) and target DVHs in sites with multiple targets. Using leave-one-out cross validation, we applied both models to a large dataset of 217 oropharynx patients. The predicted DVHs were input into an IO pipeline that generated treatment plans (BQ and gPCA plans) via an intermediate step that estimated objective function weights for an inverse planning model. The KBP predictions were compared to the clinical DVHs for benchmarking. To assess the complete pipeline, we compared the BQ and gPCA plans to both the predictions and clinical plans. To isolate the effect of the KBP predictions, we put clinical DVHs through the IO pipeline to produce clinical inverse optimized (CIO) plans. This approach also allowed us to estimate the complexity of the clinical plans. The BQ and gPCA plans were benchmarked against the CIO plans using DVH differences and clinical planning criteria. Iso-complexity plans (relative to CIO) were also generated and evaluated. Results: The BQ method tended to predict that less dose is delivered than what was observed in the clinical plans while the gPCA predictions were more similar to clinical DVHs. Both populations of KBP predictions were reproduced with inverse plans to within a median DVH difference of 3 Gy. Clinical planning criteria for OARs were satisfied most frequently by the BQ plans (74.4%), by 6.3% points more than the clinical plans. Meanwhile, target criteria were satisfied most frequently by the gPCA plans (90.2%), and by 21.2% points more than clinical plans. However, once the complexity of the plans was constrained to that of the CIO plans, the performance of the BQ plans degraded significantly. In contrast, the gPCA plans still satisfied more clinical criteria than both the clinical and CIO plans, with the most notable improvement being in target criteria. Conclusion: Our automated pipeline can successfully use DVH predictions to generate high-quality plans without human intervention. Between the two KBP methods, gPCA plans tend to achieve comparable performance as clinical plans, even when controlling for plan complexity, whereas BQ plans tended to underperform. |
6. | Automated treatment planning in radiation therapy using generative adversarial networks Proceedings Article R. Mahmood, A. Babier, A. McNiven, A. Diamant, T. C. Y. Chan In: Proceedings of the 3rd Machine Learning for Healthcare Conference, pp. 484-499, PMLR, 2018. @inproceedings{ChanTCY.Oth006c, Knowledge-based planning (KBP) is an automated approach to radiation therapy treatment planning that involves predicting desirable treatment plans before they are then corrected to deliverable ones. We propose a generative adversarial network (GAN) approach for predicting desirable 3D dose distributions that eschews the previous paradigms of site-specific feature engineering and predicting low-dimensional representations of the plan. Experiments on a dataset of oropharyngeal cancer patients show that our approach significantly outperforms previous methods on several clinical satisfaction criteria and similarity metrics. |
5. | The value of nodal information in predicting lung cancer relapse using 4DPET/4DCT Journal Article H. Li, N. Becker, S. Raman, T. C. Y. Chan, J.-P. Bissonnette In: Medical Physics, vol. 42, pp. 4727-4733, 2015. @article{ChanTCY.J026, Purpose: There is evidence that computed tomography (CT) and positron emission tomography (PET) imaging metrics are prognostic and predictive in nonsmall cell lung cancer (NSCLC) treatment outcomes. However, few studies have explored the use of standardized uptake value (SUV)-based image features of nodal regions as predictive features. The authors investigated and compared the use of tumor and node image features extracted from the radiotherapy target volumes to predict relapse in a cohort of NSCLC patients undergoing chemoradiation treatment. Methods: A prospective cohort of 25 patients with locally advanced NSCLC underwent 4DPET/4DCT imaging for radiation planning. Thirty-seven image features were derived from the CT-defined volumes and SUVs of the PET image from both the tumor and nodal target regions. The machine learning methods of logistic regression and repeated stratified five-fold cross-validation (CV) were used to predict local and overall relapses in 2 yr. The authors used well-known feature selection methods (Spearman's rank correlation, recursive feature elimination) within each fold of CV. Classifiers were ranked on their Matthew's correlation coefficient (MCC) after CV. Area under the curve, sensitivity, and specificity values are also presented. Results: For predicting local relapse, the best classifier found had a mean MCC of 0.07 and was composed of eight tumor features. For predicting overall relapse, the best classifier found had a mean MCC of 0.29 and was composed of a single feature: the volume greater than 0.5 times the maximum SUV (N). Conclusions: The best classifier for predicting local relapse had only tumor features. In contrast, the best classifier for predicting overall relapse included a node feature. Overall, the methods showed that nodes add value in predicting overall relapse but not local relapse. |
4. | Models for predicting objective function weights in prostate cancer IMRT Journal Article J. J. Boutilier, T. Lee, T. Craig, M. B. Sharpe, T. C. Y. Chan In: Medical Physics, vol. 42, pp. 1586-1595, 2015. @article{ChanTCY.J021, Purpose: To develop and evaluate the clinical applicability of advanced machine learning models that simultaneously predict multiple optimization objective function weights from patient geometry for intensity-modulated radiation therapy of prostate cancer. Methods: A previously developed inverse optimization method was applied retrospectively to determine optimal objective function weights for 315 treated patients. The authors used an overlap volume ratio (OV) of bladder and rectum for different PTV expansions and overlap volume histogram slopes (OVSR and OVSB for the rectum and bladder, respectively) as explanatory variables that quantify patient geometry. Using the optimal weights as ground truth, the authors trained and applied three prediction models: logistic regression (LR), multinomial logistic regression (MLR), and weighted K-nearest neighbor (KNN). The population average of the optimal objective function weights was also calculated. Results: The OV at 0.4 cm and OVSR at 0.1 cm features were found to be the most predictive of the weights. The authors observed comparable performance (i.e., no statistically significant difference) between LR, MLR, and KNN methodologies, with LR appearing to perform the best. All three machine learning models outperformed the population average by a statistically significant amount over a range of clinical metrics including bladder/rectum V53Gy, bladder/rectum V70Gy, and dose to the bladder, rectum, CTV, and PTV. When comparing the weights directly, the LR model predicted bladder and rectum weights that had, on average, a 73% and 74% relative improvement over the population average weights, respectively. The treatment plans resulting from the LR weights had, on average, a rectum V70Gy that was 35% closer to the clinical plan and a bladder V70Gy that was 29% closer, compared to the population average weights. Similar results were observed for all other clinical metrics. Conclusions: The authors demonstrated that the KNN and MLR weight prediction methodologies perform comparably to the LR model and can produce clinical quality treatment plans by simultaneously predicting multiple weights that capture trade-offs associated with sparing multiple OARs. |
3. | Predicting objective function weights from patient anatomy in prostate IMRT treatment planning Journal Article T. Lee, M. Hummad, T. C. Y. Chan, T. Craig, M. B. Sharpe In: Medical Physics, vol. 40, no. Article No. 121706, 2013. @article{ChanTCY.J015, Purpose: Intensity-modulated radiation therapy (IMRT) treatment planning typically combines multiple criteria into a single objective function by taking a weighted sum. The authors propose a statistical model that predicts objective function weights from patient anatomy for prostate IMRT treatment planning. This study provides a proof of concept for geometry-driven weight determination. Methods: A previously developed inverse optimization method (IOM) was used to generate optimal objective function weights for 24 patients using their historical treatment plans (i.e., dose distributions). These IOM weights were around 1% for each of the femoral heads, while bladder and rectum weights varied greatly between patients. A regression model was developed to predict a patient's rectum weight using the ratio of the overlap volume of the rectum and bladder with the planning target volume at a 1 cm expansion as the independent variable. The femoral head weights were fixed to 1% each and the bladder weight was calculated as one minus the rectum and femoral head weights. The model was validated using leave-one-out cross validation. Objective values and dose distributions generated through inverse planning using the predicted weights were compared to those generated using the original IOM weights, as well as an average of the IOM weights across all patients. Results: The IOM weight vectors were on average six times closer to the predicted weight vectors than to the average weight vector, using l_2 distance. Likewise, the bladder and rectum objective values achieved by the predicted weights were more similar to the objective values achieved by the IOM weights. The difference in objective value performance between the predicted and average weights was statistically significant according to a one-sided sign test. For all patients, the difference in rectum V54.3 Gy, rectum V70.0 Gy, bladder V54.3 Gy, and bladder V70.0 Gy values between the dose distributions generated by the predicted weights and IOM weights was less than 5 percentage points. Similarly, the difference in femoral head V54.3 Gy values between the two dose distributions was less than 5 percentage points for all but one patient. Conclusions: This study demonstrates a proof of concept that patient anatomy can be used to predict appropriate objective function weights for treatment planning. In the long term, such geometry-driven weights may serve as a starting point for iterative treatment plan design or may provide information about the most clinically relevant region of the Pareto surface to explore. |
2. | Split personalities of NHL players: Using clustering, projection and regression to measure individual point shares Proceedings Article T. C. Y. Chan, D. C. Novati In: Proceedings of the 6th Annual MIT Sloan Sports Analytics Conference, 2012. @inproceedings{ChanTCY.Oth003c, Recent literature in hockey analytics has considered the use of clustering to determine specific categories or types of NHL players. Regression analysis has then been used to measure the contribution of each of these player types to team performance. This paper uses a combination of clustering, projection and regression methods to individualize the classification of NHL players. Instead of assigning each player to only one type, the overall "personality" of the player is split into fractional components representing different player types. The result is a unique make-up for each player, which is used to quantify his individual contributions to his team's performance, a metric known as "point shares". Top ranked players in terms of point shares tend to be winners of major NHL awards, are leaders in scoring, and have the highest salaries. High point shares in a contract year may also factor into salary increases. Overall, a better understanding of individual NHL player characteristics may provide a foundation for deeper, data-driven player analysis. |
1. | Quantifying the contribution of NHL player types to team performance Journal Article T. C. Y. Chan, J. A. Cho, D. C. Novati In: Interfaces, vol. 42, pp. 131-145, 2012. @article{ChanTCY.J007, In this paper, we use k-means clustering to define distinct player types for each of the three positions on a National Hockey League (NHL) team and then use regression to determine a quantitative relationship between team performance and the player types identified in the clustering. Using NHL regular-season data from 2005–2010, we identify four forward types, four defensemen types, and three goalie types. Goalies tend to contribute the most to team performance, followed by forwards and then defensemen. We also show that once we account for salary cap and playing-time information, the value of different player types may become similar. Lastly, we illustrate how to use the regression results to analyze trades and their impact on team performance. |
Radiation Therapy
Radiation therapy is one of the primary techniques used to treat cancer. Beams of radiation are delivered from different angles around a patient, targeting a tumor at their intersection while aiming to spare nearby critical organs. I deploy a combination of robust optimization, inverse optimization, and machine learning to improve the quality of treatments and the efficiency of the treatment planning process.
RELEVANT PUBLICATIONS
43. | Impact of surgeon-radiation oncology dyads in oral cavity cancer outcomes Journal Article J. Wihlidal, A. O. Esemezie, S. H. Huang, E. Watson, R. W. Gilbert, J. Waldron, P. J. Gullane, A. Hope, J. C. Irish, B. O’Sullivan, D. B. Chepeha, J. J. H. Kim, D. Brown, B. C. J. Cho, I. J. Witterick, E. Monteiro, J. C. Davies, J. Ringash, D. P. Goldstein, S. Bratman, A. Bayley, J. R. de Almeida, T. C. Y. Chan, A. Hosni, C. M. K. L. Yao In: Annals of Surgical Oncology, vol. 32, no. 2, pp. 1270-1279, 2025. @article{ChanTCY.J131, Background. Multidisciplinary care is paramount in patient-specific decision making, especially as pertaining to oral cavity squamous cell cancer (OCSCC) treatment. Protracted surgery-postoperative-radiation (S-PORT) has a detrimental impact on OCSCC patients’ outcomes. This study examined the impact of surgeon-radiation oncologist dyads on the treatment of OCSCC, focusing on S-PORT interval and disease specific outcomes. Methods. All OCSCC patients treated in a tertiary cancer center between 2009 to 2017 were included. Patients were categorized into “dyad” and “nondyad” groups defined as whether they were treated by a paired surgeon–radiation oncology team with joint multidisciplinary clinic or shared >30% patient volumes. Univariate and multivariate logistic regression were performed to identify factors associated with a prolonged S-PORT time interval (≥8 weeks). Overall survival and locoregional recurrence were estimated and compared. Results. A total of 444 OCSCC were eligible. Treatment by a dyad was significantly less likely associated with S-PORT ≥ 8 weeks (odds ratio [OR]unadjusted: 0.65; 95% confidence interval [CI] 0.44–0.96; p = 0.03). Obtaining pre-operative radiation oncology consultation also decreased the S-PORT interval. Advanced T-category and the need for free tissue flap reconstruction increased the likelihood of prolonged S-PORT on univariate but not multivariate analysis. No significant differences were observed in overall survival or locoregional recurrence by dyad status nor S-PORT (p > 0.05). Conclusions. Surgeon-radiation oncology dyads significantly minimized time from surgery to postoperative radiation in OCSCC. While improvement in overall survival or locoregional recurrence was not observed, these findings support close knit collaborative multidisciplinary treatment care models, including dyad-based care. |
42. | Synergizing radiation oncology and operations research Book Chapter S. Kim, T. C. Y. Chan In: Fox, C. J.; Munbodh, R. (Ed.): Workflow Optimization in Radiation Oncology: From Theory to Clinical Implementation, no. Medical Physics Monograph 41, Chapter 7, Medical Physics Publishing, Madison, 2024, ISBN: 978-1-951134-31-0. @inbook{ChanTCY.Oth012, |
41. | Knowledge-based planning for Gamma Knife Journal Article B. Zhang, A. Babier, M. Ruschin, T. C. Y. Chan In: Medical Physics, vol. 51, no. 5, pp. 3131-3818, 2024. @article{ChanTCY.J0117, Background: Current methods for Gamma Knife (GK) treatment planning utilizes either manual forward planning, where planners manually place shots in a tumor to achieve a desired dose distribution, or inverse planning, whereby the dose delivered to a tumor is optimized for multiple objectives based on established metrics. For other treatment modalities like IMRT and VMAT, there has been a recent push to develop knowledge-based planning (KBP) pipelines to address the limitations presented by forward and inverse planning. However, no complete KBP pipeline has been created for GK. Purpose: To develop a novel (KBP) pipeline, using inverse optimization (IO) with 3D dose predictions for GK. Methods: Data were obtained for 349 patients from Sunnybrook Health Sciences Centre. A 3D dose prediction model was trained using 322 patients, based on a previously published deep learning methodology, and dose predictions were generated for the remaining 27 out-of-sample patients. A generalized IO model was developed to learn objective function weights from dose predictions. These weights were then used in an inverse planning model to generate deliverable treatment plans. A dose mimicking (DM) model was also implemented for comparison.The quality of the resulting plans was compared to their clinical counterparts using standard GK quality metrics.The performance of the models was also characterized with respect to the dose predictions. Results: Across all quality metrics, plans generated using the IO pipeline performed at least as well as or better than the respective clinical plans. The average conformity and gradient indices of IO plans was 0.737±0.158 and 3.356±1.030 respectively, compared to 0.713±0.124 and 3.452±1.123 for the clinical plans. IO plans also performed better than DM plans for five of the six quality metrics. Plans generated using IO also have average treatment times comparable to that of clinical plans.With regards to the dose predictions, predictions with higher conformity tend to result in higher quality KBP plans. Conclusions: Plans resulting from an IO KBP pipeline are, on average, of equal or superior quality compared to those obtained through manual planning. The results demonstrate the potential for the use of KBP to generate GK treatment with minimal human intervention. |
40. | Negative externality on service level across priority classes: Evidence from a radiology workflow platform Journal Article S. Lagzi, B. F. Quiroga, G. Romero, N. Howard, T. C. Y. Chan In: Journal of Operations Management, vol. 69, no. 8, pp. 1209-1377, 2023. @article{ChanTCY.J0107, We study the potential negative impact of imbalanced compensation schemes on firm performance. We analyze data from a radiology workflow platform that connects off-site radiologists with hospitals. These radiologists select tasks from a common pool, while service level is defined by priority-specific turnaround time targets. However, imbalances between pay and workload of different tasks could result in higher priority tasks with low pay-to-workload ratio receiving poorer service. We investigate this hypothesis, showing turnaround time is decreasing in pay-to-workload for lower priority tasks, whereas it is increasing in workload for high-priority tasks. Crucially, we find evidence of an externality effect: Having many economically attractive tasks with low priority can lead to longer turnaround times for higher priority tasks, increasing their likelihood of delay, thus partially defeating the purpose of the priority classes. |
39. | 3D dose prediction for Gamma Knife radiosurgery using deep learning and data modification Journal Article B. Zhang, A. Babier, T. C. Y. Chan, M. Ruschin In: Physica Medica, vol. 106, pp. 102533, 2023. @article{ChanTCY.J0104, Purpose: To develop a machine learning-based, 3D dose prediction methodology for Gamma Knife (GK) radiosurgery. The methodology accounts for cases involving targets of any number, size, and shape. Methods: Data from 322 GK treatment plans was modified by isolating and cropping the contoured MRI and clinical dose distributions based on tumor location, then scaling the resulting tumor spaces to a standard size. An accompanying 3D tensor was created for each instance to account for tumor size. The modified dataset for 272 patients was used to train both a generative adversarial network (GAN-GK) and a 3D U-Net model (U-Net-GK). Unmodified data was used to train equivalent baseline models. All models were used to predict the dose distribution of 50 out-of-sample patients. Prediction accuracy was evaluated using gamma, with criteria of 4%/2mm, 3%/3mm, 3%/1mm and 1%/1mm. Prediction quality was assessed using coverage, selectivity, and conformity indices. Results: The predictions resulting from GAN-GK and U-Net-GK were similar to their clinical counterparts, with average gamma (4%/2mm) passing rates of 84.9 ± 15.3% and 83.1 ± 17.2%, respectively. In contrast, the gamma passing rate of baseline models were significantly worse than their respective GK-specific models (p < 0.001) at all criterion levels. The quality of GK-specific predictions was also similar to that of clinical plans. Conclusion: Deep learning models can use GK-specific data modification to predict 3D dose distributions for GKRS plans with a large range in size, shape, or number of targets. Standard deep learning models applied to unmodified GK data generated poorer predictions. |
38. | OpenKBP-Opt: An international and reproducible evaluation of 76 knowledge-based planning pipelines Journal Article A. Babier, R. Mahmood, B. Zhang, V. C. L. Alves, A. M. Barragán-Montero, J. Beaudry, C. E. Cardenas, Y. Chang, Z. Chen, J. Chun, K. Diaz, H. D. Eraso, E. Faustmann, S. Gaj, S. Gay, M. Gronberg, B. Guo, J. He, G. Heilemann, S. Hira, Y. Huang, F. Ji, D. Jiang, J. C. Jimenez Giraldo, H. Lee, J. Lian, S. Liu, K.-C. Liu, J. Marrugo, K. Miki, K. Nakamura, T. Netherton, D. Nguyen, H. Nourzadeh, A. F. I. Osman, Z. Peng, J. D. Quinto Muñoz, C. Ramsl, D. J. Rhee, J. D. Rodriguez, H. Shan, J. V. Siebers, M. H. Soomro, K. Sun, A. Usuga Hoyos, C. Valderrama, R. Verbeek, E. Wang, S. Willems, Q. Wu, X. Xu, S. Yang, L. Yuan, X. Zhu, L. Zimmermann, K. L. Moore, T. G. Purdie, A. L. McNiven, T. C. Y. Chan In: Physics in Medicine and Biology, vol. 67, no. Article No. 185012, 2022. @article{ChanTCY.J099, Objective. To establish an open framework for developing plan optimization models for knowledge-based planning (KBP). Approach. Our framework includes radiotherapy treatment data (i.e. reference plans) for 100 patients with head-and-neck cancer who were treated with intensity-modulated radiotherapy. That data also includes high-quality dose predictions from 19 KBP models that were developed by different research groups using out-of-sample data during the OpenKBP Grand Challenge. The dose predictions were input to four fluence-based dose mimicking models to form 76 unique KBP pipelines that generated 7600 plans (76 pipelines × 100 patients). The predictions and KBP-generated plans were compared to the reference plans via: the dose score, which is the average mean absolute voxel-by-voxel difference in dose; the deviation in dose-volume histogram (DVH) points; and the frequency of clinical planning criteria satisfaction. We also performed a theoretical investigation to justify our dose mimicking models. Main results. The range in rank order correlation of the dose score between predictions and their KBP pipelines was 0.50–0.62, which indicates that the quality of the predictions was generally positively correlated with the quality of the plans. Additionally, compared to the input predictions, the KBP-generated plans performed significantly better (P < 0.05; one-sided Wilcoxon test) on 18 of 23 DVH points. Similarly, each optimization model generated plans that satisfied a higher percentage of criteria than the reference plans, which satisfied 3.5% more criteria than the set of all dose predictions. Lastly, our theoretical investigation demonstrated that the dose mimicking models generated plans that are also optimal for an inverse planning model. Significance. This was the largest international effort to date for evaluating the combination of KBP prediction and optimization models. We found that the best performing models significantly outperformed the reference dose and dose predictions. In the interest of reproducibility, our data and code is freely available. |
37. | Robust direct aperture optimization for radiation therapy treatment planning Journal Article D. A. Ripsman, T. G. Purdie, T. C. Y. Chan, H. Mahmoudzadeh In: INFORMS Journal on Computing, vol. 34, no. 4, pp. 2017-2038, 2022. @article{ChanTCY.J093, Intensity-modulated radiation therapy (IMRT) allows for the design of customized, highly-conformal treatments for cancer patients. Creating IMRT treatment plans, however, is a mathematically complex process, which is often tackled in multiple, simpler stages. This sequential approach typically separates radiation dose requirements from mechanical deliverability considerations, which may result in suboptimal treatment quality. For patient health to be considered paramount, holistic models must address these plan elements concurrently, eliminating quality loss between stages. This combined direct aperture optimization (DAO) approach is rarely paired with uncertainty mitigation techniques, such as robust optimization, due to the inherent complexity of both parts. This paper outlines a robust DAO (RDAO) model and discusses novel methodologies for efficiently integrating salient constraints. Since the highly-complex RDAO model is difficult to solve, an original candidate plan generation (CPG) heuristic is proposed. The CPG produces rapid, high-quality, feasible plans, which are immediately clinically viable, and can also be used to generate a feasible incumbent solution for warm starting the RDAO model. Computational results obtained using clinical patient datasets with motion uncertainty show the benefit of incorporating the CPG, both in terms of first incumbent solution and final output plan quality. |
36. | Sparse flexible design: A machine learning approach Journal Article T. C. Y. Chan, D. Letourneau, B. Potter In: Flexible Services and Manufacturing Journal, vol. 34, pp. 1066-1116, 2022. @article{ChanTCY.J091, For a general production network, state-of-the-art methods for constructing sparse flexible designs are heuristic in nature, typically computing a proxy for the quality of unseen networks and using that estimate in a greedy manner to modify a current design. This paper develops two machine learning-based approaches to constructing sparse flexible designs that leverage a neural network to accurately and quickly predict the performance of large numbers of candidate designs. We demonstrate that our heuristics are competitive with existing approaches and produce high-quality solutions for both balanced and unbalanced networks. Finally, we introduce a novel application of process flexibility in healthcare operations to demonstrate the effectiveness of our approach in a large numerical case study. We study the flexibility of linear accelerators that deliver radiation to treat various types of cancer. We demonstrate how clinical constraints can be easily absorbed into the machine learning subroutine and how our sparse flexible treatment networks meet or beat the performance of those designed by state-of-the-art methods. |
35. | OpenKBP: The Open-Access Knowledge-Based Planning Grand Challenge and Dataset Journal Article A. Babier, B. Zhang, R. Mahmood, K. L. Moore, T. G. Purdie, A. L. McNiven, T. C. Y. Chan In: Medical Physics, vol. 48, no. 9, pp. 5549-5561, 2021. @article{ChanTCY.J078, Purpose: To advance fair and consistent comparisons of dose prediction methods for knowledge-based planning (KBP) in radiation therapy research. Methods: We hosted OpenKBP, a 2020 AAPM Grand Challenge, and challenged participants to develop the best method for predicting the dose of contoured computed tomography (CT) images. The models were evaluated according to two separate scores: (a) dose score, which evaluates the full three-dimensional (3D) dose distributions, and (b) dose-volume histogram (DVH) score, which evaluates a set DVH metrics. We used these scores to quantify the quality of the models based on their out-of-sample predictions. To develop and test their models, participants were given the data of 340 patients who were treated for head-and-neck cancer with radiation therapy. The data were partitioned into training (n = 200), validation (n = 40), and testing (n = 100) datasets. All participants performed training and validation with the corresponding datasets during the first (validation) phase of the Challenge. In the second (testing) phase, the participants used their model on the testing data to quantify the out-of-sample performance, which was hidden from participants and used to determine the final competition ranking. Participants also responded to a survey to summarize their models. Results: The Challenge attracted 195 participants from 28 countries, and 73 of those participants formed 44 teams in the validation phase, which received a total of 1750 submissions. The testing phase garnered submissions from 28 of those teams, which represents 28 unique prediction methods. On average, over the course of the validation phase, participants improved the dose and DVH scores of their models by a factor of 2.7 and 5.7, respectively. In the testing phase one model achieved the best dose score (2.429) and DVH score (1.478), which were both significantly better than the dose score (2.564) and the DVH score (1.529) that was achieved by the runner-up models. Lastly, many of the top performing teams reported that they used generalizable techniques (e.g., ensembles) to achieve higher performance than their competition. Conclusion: OpenKBP is the first competition for knowledge-based planning research. The Challenge helped launch the first platform that enables researchers to compare KBP prediction methods fairly and consistently using a large open-source dataset and standardized metrics. OpenKBP has also democratized KBP research by making it accessible to everyone, which should help accelerate the progress of KBP research. The OpenKBP datasets are available publicly to help benchmark future KBP research. |
34. | An ensemble learning framework for model fitting and evaluation in inverse linear optimization Journal Article A. Babier, T. C. Y. Chan, T. Lee, R. Mahmood, D. Terekhov In: INFORMS Journal on Optimization, vol. 3, no. 2, pp. 119-138, 2021. @article{ChanTCY.J075, We develop a generalized inverse optimization framework for fitting the cost vector of a single linear optimization problem given an ensemble of observed decisions. We unify multiple variants in the inverse optimization literature under a common template and derive assumption-free and exact solution methods for each variant. We extend a goodness-of-fit metric previously introduced for the problem with a single observed decision to this new setting, proving and numerically demonstrating several important properties. Finally, to illustrate our framework, we develop a novel inverse optimization-driven procedure for automated radiation therapy treatment planning. Here, the inverse optimization model leverages the combined power of an ensemble of dose predictions produced by different machine learning models to construct clinical treatment plans that better trade off between the competing clinical objectives that are used for plan evaluation in practice. |
33. | Sampling from the complement of a polyhedron: An MCMC algorithm for data augmentation Journal Article T. C. Y. Chan, A. Diamant, R. Mahmood In: Operations Research Letters, vol. 48, pp. 744–751, 2020. @article{ChanTCY.J072, We present an MCMC algorithm for sampling from the complement of a polyhedron. Our approach is based on the Shake-and-bake algorithm for sampling from the boundary of a set and provably covers the complement. We use this algorithm for data augmentation in a machine learning task of classifying a hidden feasible set in a data-driven optimization pipeline. Numerical results on simulated and MIPLIB instances demonstrate that our algorithm, along with a supervised learning technique, outperforms conventional unsupervised baselines. |
32. | The importance of evaluating the complete automated knowledge-based planning pipeline Journal Article A. Babier, R. Mahmood, A. L. McNiven, A. Diamant, T. C. Y. Chan In: Physica Medica, vol. 72, pp. 73-79, 2020. @article{ChanTCY.J064, We determine how prediction methods combine with optimization methods in two-stage knowledge-based planning (KBP) pipelines to produce radiation therapy treatment plans. We trained two dose prediction methods, a generative adversarial network (GAN) and a random forest (RF) with the same 130 treatment plans. The models were applied to 87 out-of-sample patients to create two sets of predicted dose distributions that were used as input to two optimization models. The first optimization model, inverse planning (IP), estimates weights for dose-objectives from a predicted dose distribution and generates new plans using conventional inverse planning. The second optimization model, dose mimicking (DM), minimizes the sum of one-sided quadratic penalties between the predictions and the generated plans using several dose-objectives. Altogether, four KBP pipelines (GAN-IP, GAN-DM, RF-IP, and RF-DM) were constructed and benchmarked against the corresponding clinical plans using clinical criteria; the error of both prediction methods was also evaluated. The best performing plans were GAN-IP plans, which satisfied the same criteria as their corresponding clinical plans (78%) more often than any other KBP pipeline. However, GAN did not necessarily provide the best prediction for the second-stage optimization models. Specifically, both the RF-IP and RF-DM plans satisfied the same criteria as the clinical plans 25% and 15% more often than GAN-DM plans (the worst performing plans), respectively. GAN predictions also had a higher mean absolute error (3.9 Gy) than those from RF (3.6 Gy). We find that state-of-the-art prediction methods when paired with different optimization algorithms, produce treatment plans with considerable variation in quality. |
31. | Knowledge-based automated planning with three-dimensional generative adversarial networks Journal Article A. Babier, R. Mahmood, A. L. McNiven, A. Diamant, T. C. Y. Chan In: Medical Physics, vol. 47, pp. 297-306, 2020. @article{ChanTCY.J059, Purpose: To develop a knowledge-based automated planning pipeline that generates treatment plans without feature engineering, using deep neural network architectures for predicting three-dimensional (3D) dose. Methods: Our knowledge-based automated planning (KBAP) pipeline consisted of a knowledge-based planning (KBP) method that predicts dose for a contoured computed tomography (CT) image followed by two optimization models that learn objective function weights and generate fluence-based plans, respectively. We developed a novel generative adversarial network (GAN)-based KBP approach, a 3D GAN model, which predicts dose for the full 3D CT image at once and accounts for correlations between adjacent CT slices. Baseline comparisons were made against two state-of-the-art deep learning–based KBP methods from the literature. We also developed an additional benchmark, a two-dimensional (2D) GAN model which predicts dose to each axial slice independently. For all models, we investigated the impact of multiplicatively scaling the predictions before optimization, such that the predicted dose distributions achieved all target clinical criteria. Each KBP model was trained on 130 previously delivered oropharyngeal treatment plans. Performance was tested on 87 out-of-sample previously delivered treatment plans. All KBAP plans were evaluated using clinical planning criteria and compared to their corresponding clinical plans. KBP prediction quality was assessed using dose-volume histogram (DVH) differences from the corresponding clinical plans. Results: The best performing KBAP plans were generated using predictions from the 3D GAN model that were multiplicatively scaled. These plans satisfied 77% of all clinical criteria, compared to the clinical plans, which satisfied 67% of all criteria. In general, multiplicatively scaling predictions prior to optimization increased the fraction of clinical criteria satisfaction by 11% relative to the plans generated with nonscaled predictions. Additionally, these KBAP plans satisfied the same criteria as the clinical plans 84% and 8% more frequently as compared to the two benchmark methods, respectively. Conclusions: We developed the first knowledge-based automated planning framework using a 3D generative adversarial network for prediction. Our results, based on 217 oropharyngeal cancer treatment plans, demonstrated superior performance in satisfying clinical criteria and generated more realistic plans as compared to the previous state-of-the-art approaches. |
30. | Inverse optimization: Closed-form solutions, geometry, and goodness of fit Journal Article T. C. Y. Chan, T. Lee, D. Terekhov In: Management Science, vol. 65, pp. 1115-1135, 2019. @article{ChanTCY.J050, In classical inverse linear optimization, one assumes that a given solution is a candidate to be optimal. Real data are imperfect and noisy, so there is no guarantee that this assumption is satisfied. Inspired by regression, this paper presents a unified framework for cost function estimation in linear optimization comprising a general inverse optimization model and a corresponding goodness-of-fit metric. Although our inverse optimization model is nonconvex, we derive a closed-form solution and present the geometric intuition. Our goodness-of-fit metric, ρ, the coefficient of complementarity, has similar properties to R^2 from regression and is quasi-convex in the input data, leading to an intuitive geometric interpretation. While ρ is computable in polynomial time, we derive a lower bound that possesses the same properties, is tight for several important model variations, and is even easier to compute. We demonstrate the application of our framework for model estimation and evaluation in production planning and cancer therapy. |
29. | The importance of evaluating the complete knowledge-based automated planning pipeline Proceedings Article A. Babier, R. Mahmood, A. Diamant, A. McNiven, T. C. Y. Chan In: Proceedings of the International Conference on the use of Computers in Radiation Therapy, 2019. @inproceedings{ChanTCY.Oth007c, |
28. | Robust radiotherapy planning Journal Article J. Unkelbach, M. Alber, M. Bangert, R. Bokrantz, T. C. Y. Chan, J. Deasy, A. Fredriksson, B. L. Gorissen, M. van Herk, W. Liu, H. Mahmoudzadeh, O. Nohadani, J. V. Siebers, M. Witte, H. Xu In: Physics in Medicine and Biology, vol. 63, no. Article No. 22TR02, 2018. @article{ChanTCY.J049, Motion and uncertainty in radiotherapy is traditionally handled via margins. The clinical target volume (CTV) is expanded to a larger planning target volume (PTV), which is irradiated to the prescribed dose. However, the PTV concept has several limitations, especially in proton therapy. Therefore, robust and probabilistic optimization methods have been developed that directly incorporate motion and uncertainty into treatment plan optimization for intensity modulated radiotherapy (IMRT) and intensity modulated proton therapy (IMPT). Thereby, the explicit definition of a PTV becomes obsolete and treatment plan optimization is directly based on the CTV. Initial work focused on random and systematic setup errors in IMRT. Later, inter-fraction prostate motion and intra-fraction lung motion became a research focus. Over the past ten years, IMPT has emerged as a new application for robust planning methods. In proton therapy, range or setup errors may lead to dose degradation and misalignment of dose contributions from different beams – a problem that cannot generally be addressed by margins. Therefore, IMPT has led to the first implementations of robust planning methods in commercial planning systems, making these methods available for clinical use. This paper first summarizes the limitations of the PTV concept. Subsequently, robust optimization methods are introduced and their applications in IMRT and IMPT planning are reviewed. |
27. | A small number of objective function weight vectors is sufficient for automated treatment planning in prostate cancer Journal Article A. Goli, J. J. Boutilier, M. B. Sharpe, T. Craig, T. C. Y. Chan In: Physics in Medicine and Biology, vol. 63, no. Article No. 195004, 2018. @article{ChanTCY.J045, Current practice for treatment planning optimization can be both inefficient and time consuming. In this paper, we propose an automated planning methodology that aims to combine both explorative and prescriptive approaches for improving the efficiency and the quality of the treatment planning process. Given a treatment plan, our explorative approach explores trade-offs between different objectives and finds an acceptable region for objective function weights via inverse optimization. Intuitively, the shape and size of these regions describe how 'sensitive' a patient is to perturbations in objective function weights. We then develop an integer programming-based prescriptive approach that exploits the information encoded by these regions to find a set of five representative objective function weight vectors such that for each patient there exists at least one representative weight vector that can produce a high quality treatment plan. Using 315 patients from Princess Margaret Cancer Centre, we show that the produced treatment plans are comparable and, for 96% of cases, improve upon the inversely optimized plans that are generated from the historical clinical treatment plans. |
26. | Inverse optimization of objective function weights for treatment planning using clinical dose-volume histograms Journal Article A. Babier, J. J. Boutilier, M. B. Sharpe, A. L. McNiven, T. C. Y. Chan In: Physics in Medicine and Biology, vol. 63, no. Article No. 105004, 2018. @article{ChanTCY.J044, We developed and evaluated a novel inverse optimization (IO) model to estimate objective function weights from clinical dose-volume histograms (DVHs). These weights were used to solve a treatment planning problem to generate 'inverse plans' that had similar DVHs to the original clinical DVHs. Our methodology was applied to 217 clinical head and neck cancer treatment plans that were previously delivered at Princess Margaret Cancer Centre in Canada. Inverse plan DVHs were compared to the clinical DVHs using objective function values, dose-volume differences, and frequency of clinical planning criteria satisfaction. Median differences between the clinical and inverse DVHs were within 1.1 Gy. For most structures, the difference in clinical planning criteria satisfaction between the clinical and inverse plans was at most 1.4%. For structures where the two plans differed by more than 1.4% in planning criteria satisfaction, the difference in average criterion violation was less than 0.5 Gy. Overall, the inverse plans were very similar to the clinical plans. Compared with a previous inverse optimization method from the literature, our new inverse plans typically satisfied the same or more clinical criteria, and had consistently lower fluence heterogeneity. Overall, this paper demonstrates that DVHs, which are essentially summary statistics, provide sufficient information to estimate objective function weights that result in high quality treatment plans. However, as with any summary statistic that compresses three-dimensional dose information, care must be taken to avoid generating plans with undesirable features such as hotspots; our computational results suggest that such undesirable spatial features were uncommon. Our IO-based approach can be integrated into the current clinical planning paradigm to better initialize the planning process and improve planning efficiency. It could also be embedded in a knowledge-based planning or adaptive radiation therapy framework to automatically generate a new plan given a predicted or updated target DVH, respectively. |
25. | Knowledge-based automated planning for oropharyngeal cancer Journal Article A. Babier, J. J. Boutilier, A. L. McNiven, T. C. Y. Chan In: Medical Physics, vol. 45, pp. 2875-2883, 2018. @article{ChanTCY.J043, Purpose: The purpose of this study was to automatically generate radiation therapy plans for oropharynx patients by combining knowledge-based planning (KBP) predictions with an inverse optimization (IO) pipeline. Methods: We developed two KBP approaches, the bagging query (BQ) method and the generalized principal component analysis-based (gPCA) method, to predict achievable dose–volume histograms (DVHs). These approaches generalize existing methods by predicting physically feasible organ-at-risk (OAR) and target DVHs in sites with multiple targets. Using leave-one-out cross validation, we applied both models to a large dataset of 217 oropharynx patients. The predicted DVHs were input into an IO pipeline that generated treatment plans (BQ and gPCA plans) via an intermediate step that estimated objective function weights for an inverse planning model. The KBP predictions were compared to the clinical DVHs for benchmarking. To assess the complete pipeline, we compared the BQ and gPCA plans to both the predictions and clinical plans. To isolate the effect of the KBP predictions, we put clinical DVHs through the IO pipeline to produce clinical inverse optimized (CIO) plans. This approach also allowed us to estimate the complexity of the clinical plans. The BQ and gPCA plans were benchmarked against the CIO plans using DVH differences and clinical planning criteria. Iso-complexity plans (relative to CIO) were also generated and evaluated. Results: The BQ method tended to predict that less dose is delivered than what was observed in the clinical plans while the gPCA predictions were more similar to clinical DVHs. Both populations of KBP predictions were reproduced with inverse plans to within a median DVH difference of 3 Gy. Clinical planning criteria for OARs were satisfied most frequently by the BQ plans (74.4%), by 6.3% points more than the clinical plans. Meanwhile, target criteria were satisfied most frequently by the gPCA plans (90.2%), and by 21.2% points more than clinical plans. However, once the complexity of the plans was constrained to that of the CIO plans, the performance of the BQ plans degraded significantly. In contrast, the gPCA plans still satisfied more clinical criteria than both the clinical and CIO plans, with the most notable improvement being in target criteria. Conclusion: Our automated pipeline can successfully use DVH predictions to generate high-quality plans without human intervention. Between the two KBP methods, gPCA plans tend to achieve comparable performance as clinical plans, even when controlling for plan complexity, whereas BQ plans tended to underperform. |
24. | A mixed-integer optimization approach for homogeneous magnet design Journal Article I. Dayarian, T. C. Y. Chan, D. Jaffray, T. Stanescu In: Technology, vol. 6, pp. 49-58, 2018. @article{ChanTCY.J041, Magnetic resonance imaging (MRI) is a powerful diagnostic tool that has become the imaging modality of choice for soft-tissue visualization in radiation therapy. Emerging technologies aim to integrate MRI with a medical linear accelerator to form novel cancer therapy systems (MR-linac), but the design of these systems to date relies on heuristic procedures. This paper develops an exact, optimization-based approach for magnet design that 1) incorporates the most accurate physics calculations to date, 2) determines precisely the relative spatial location, size, and current magnitude of the magnetic coils, 3) guarantees field homogeneity inside the imaging volume, 4) produces configurations that satisfy, for the first time, small-footprint feasibility constraints required for MR-linacs. Our approach leverages modern mixed-integer programming (MIP), enabling significant flexibility in magnet design generation, e.g., controlling the number of coils and enforcing symmetry between magnet poles. Our numerical results demonstrate the superiority of our method versus current mainstream methods. |
23. | Trade-off preservation in inverse multi-objective convex optimization Journal Article T. C. Y. Chan, T. Lee In: European Journal of Operational Research, vol. 270, pp. 25-39, 2018. @article{ChanTCY.J040, Given an input solution that may not be Pareto optimal, we present a new inverse optimization methodology for multi-objective convex optimization that determines a weight vector producing a weakly Pareto optimal solution that preserves the decision maker's trade-off intention encoded in the input solution. We introduce a notion of trade-off preservation, which we use as a measure of similarity for approximating the input solution, and show its connection with minimizing an optimality gap. We propose a linear approximation to the inverse model and a successive linear programming algorithm that balance between trade-off preservation and computational efficiency, and show that our model encompasses many of the existing inverse optimization models from the literature. We demonstrate the proposed method using clinical data from prostate cancer radiation therapy. |
22. | Automated treatment planning in radiation therapy using generative adversarial networks Proceedings Article R. Mahmood, A. Babier, A. McNiven, A. Diamant, T. C. Y. Chan In: Proceedings of the 3rd Machine Learning for Healthcare Conference, pp. 484-499, PMLR, 2018. @inproceedings{ChanTCY.Oth006c, Knowledge-based planning (KBP) is an automated approach to radiation therapy treatment planning that involves predicting desirable treatment plans before they are then corrected to deliverable ones. We propose a generative adversarial network (GAN) approach for predicting desirable 3D dose distributions that eschews the previous paradigms of site-specific feature engineering and predicting low-dimensional representations of the plan. Experiments on a dataset of oropharyngeal cancer patients show that our approach significantly outperforms previous methods on several clinical satisfaction criteria and similarity metrics. |
21. | Sample size requirements for knowledge-based treatment planning Journal Article J. J. Boutilier, T. Craig, M. B. Sharpe, T. C. Y. Chan In: Medical Physics, vol. 43, pp. 1212-1221, 2016. @article{ChanTCY.J029, Purpose: To determine how training set size affects the accuracy of knowledge-based treatment planning (KBP) models. Methods: The authors selected four models from three classes of KBP approaches, corresponding to three distinct quantities that KBP models may predict: dose–volume histogram (DVH) points, DVH curves, and objective function weights. DVH point prediction is done using the best plan from a database of similar clinical plans; DVH curve prediction employs principal component analysis and multiple linear regression; and objective function weights uses either logistic regression or K-nearest neighbors. The authors trained each KBP model using training sets of sizes n = 10, 20, 30, 50, 75, 100, 150, and 200. The authors set aside 100 randomly selected patients from their cohort of 315 prostate cancer patients from Princess Margaret Cancer Center to serve as a validation set for all experiments. For each value of n, the authors randomly selected 100 different training sets with replacement from the remaining 215 patients. Each of the 100 training sets was used to train a model for each value of n and for each KBT approach. To evaluate the models, the authors predicted the KBP endpoints for each of the 100 patients in the validation set. To estimate the minimum required sample size, the authors used statistical testing to determine if the median error for each sample size from 10 to 150 is equal to the median error for the maximum sample size of 200. Results: The minimum required sample size was different for each model. The DVH point prediction method predicts two dose metrics for the bladder and two for the rectum. The authors found that more than 200 samples were required to achieve consistent model predictions for all four metrics. For DVH curve prediction, the authors found that at least 75 samples were needed to accurately predict the bladder DVH, while only 20 samples were needed to predict the rectum DVH. Finally, for objective function weight prediction, at least 10 samples were needed to train the logistic regression model, while at least 150 samples were required to train the K-nearest neighbor methodology. Conclusions: In conclusion, the minimum required sample size needed to accurately train KBP models for prostate cancer depends on the specific model and endpoint to be predicted. The authors' results may provide a lower bound for more complicated tumor sites. |
20. | Constraint generation methods for robust optimization in radiation therapy Journal Article H. Mahmoudzadeh, T. G. Purdie, T. C. Y. Chan In: Operations Research for Health Care, vol. 8, pp. 85-90, 2016. @article{ChanTCY.J027, We develop a constraint generation solution method for robust optimization problems in radiation therapy in which the problems include a large number of robust constraints. Each robust constraint must hold for any realization of an uncertain parameter within a given uncertainty set. Because the problems are large scale, the robust counterpart is computationally challenging to solve. To address this challenge, we explore different strategies of adding constraints in a constraint generation solution approach. We motivate and demonstrate our approach using robust intensity-modulated radiation therapy treatment planning for breast cancer. We use clinical data to compare the computational efficiency of our constraint generation strategies with that of directly solving the robust counterpart. |
19. | The value of nodal information in predicting lung cancer relapse using 4DPET/4DCT Journal Article H. Li, N. Becker, S. Raman, T. C. Y. Chan, J.-P. Bissonnette In: Medical Physics, vol. 42, pp. 4727-4733, 2015. @article{ChanTCY.J026, Purpose: There is evidence that computed tomography (CT) and positron emission tomography (PET) imaging metrics are prognostic and predictive in nonsmall cell lung cancer (NSCLC) treatment outcomes. However, few studies have explored the use of standardized uptake value (SUV)-based image features of nodal regions as predictive features. The authors investigated and compared the use of tumor and node image features extracted from the radiotherapy target volumes to predict relapse in a cohort of NSCLC patients undergoing chemoradiation treatment. Methods: A prospective cohort of 25 patients with locally advanced NSCLC underwent 4DPET/4DCT imaging for radiation planning. Thirty-seven image features were derived from the CT-defined volumes and SUVs of the PET image from both the tumor and nodal target regions. The machine learning methods of logistic regression and repeated stratified five-fold cross-validation (CV) were used to predict local and overall relapses in 2 yr. The authors used well-known feature selection methods (Spearman's rank correlation, recursive feature elimination) within each fold of CV. Classifiers were ranked on their Matthew's correlation coefficient (MCC) after CV. Area under the curve, sensitivity, and specificity values are also presented. Results: For predicting local relapse, the best classifier found had a mean MCC of 0.07 and was composed of eight tumor features. For predicting overall relapse, the best classifier found had a mean MCC of 0.29 and was composed of a single feature: the volume greater than 0.5 times the maximum SUV (N). Conclusions: The best classifier for predicting local relapse had only tumor features. In contrast, the best classifier for predicting overall relapse included a node feature. Overall, the methods showed that nodes add value in predicting overall relapse but not local relapse. |
18. | Robust PET-guided intensity-modulated radiation therapy Journal Article H. Li, J. P. Bissonnette, T. Purdie, T. C. Y. Chan In: Medical Physics, vol. 42, pp. 4863-4871, 2015. @article{ChanTCY.J025, Purpose: Functional image guided intensity-modulated radiation therapy has the potential to improve cancer treatment quality by basing treatment parameters such as heterogeneous dose distributions information derived from imaging. However, such heterogeneous dose distributions are subject to imaging uncertainty. In this paper, the authors develop a robust optimization model to design plans that are desensitized to imaging uncertainty. Methods: Starting from the pretreatment fluorodeoxyglucose-positron emission tomography scans, the authors use the raw voxel standard uptake values (SUVs) as input into a series of intermediate functions to transform the SUV into a desired dose. The calculated desired doses were used as an input into a robust optimization model to generate beamlet intensities. For each voxel, the authors assume that the true SUV cannot be observed but instead resides in an interval centered on the nominal (i.e., observed) SUV. Then the authors evaluated the nominal and robust solutions through a simulation study. The simulation considered the effect of the true SUV being different from the nominal SUV on the quality of the treatment plan. Treatment plans were compared on the metrics of objective function value and tumor control probability (TCP). Results: Computational results demonstrate the potential for improvements in tumor control probability and deviation from the desired dose distribution compared to a nonrobust model while maintaining acceptable tissue dose. Conclusions: Robust optimization can help design treatment plans that are more stable in the presence of image value uncertainties. |
17. | The perils of adapting to dose errors in radiation therapy Journal Article V. V. Mišić, T. C. Y. Chan In: PLOS ONE, vol. 10, no. Article No. e0125335, 2015. @article{ChanTCY.J024, We consider adaptive robust methods for lung cancer that are also dose-reactive, wherein the treatment is modified after each treatment session to account for the dose delivered in prior treatment sessions. Such methods are of interest because they potentially allow for errors in the delivered dose to be corrected as the treatment progresses, thereby ensuring that the tumor receives a sufficient dose at the end of the treatment. We show through a computational study with real lung cancer patient data that while dose reaction is beneficial with respect to the final dose distribution, it may lead to exaggerated daily underdose and overdose relative to non-reactive methods that grows as the treatment progresses. However, by combining dose reaction with a mechanism for updating an estimate of the uncertainty, the magnitude of this growth can be mitigated substantially. The key finding of this paper is that reacting to dose errors – an adaptation strategy that is both simple and intuitively appealing – may backfire and lead to treatments that are clinically unacceptable. |
16. | Adaptive and robust radiation therapy in the presence of drift Journal Article P. A. Mar, T. C. Y. Chan In: Physics in Medicine and Biology, vol. 60, pp. 3599-3615, 2015. @article{ChanTCY.J023, Combining adaptive and robust optimization in radiation therapy has the potential to mitigate the negative effects of both intrafraction and interfraction uncertainty over a fractionated treatment course. A previously developed adaptive and robust radiation therapy (ARRT) method for lung cancer was demonstrated to be effective when the sequence of breathing patterns was well-behaved. In this paper, we examine the applicability of the ARRT method to less well-behaved breathing patterns. We develop a novel method to generate sequences of probability mass functions that represent different types of drift in the underlying breathing pattern. Computational results derived from applying the ARRT method to these sequences demonstrate that the ARRT method is effective for a much broader class of breathing patterns than previously demonstrated. |
15. | Robust optimization methods for cardiac sparing in tangential breast IMRT Journal Article H. Mahmoudzadeh, J. Lee, T. C. Y. Chan, T. G. Purdie In: Medical Physics, vol. 42, pp. 2212-2222, 2015. @article{ChanTCY.J022, Purpose: In left-sided tangential breast intensity modulated radiation therapy (IMRT), the heart may enter the radiation field and receive excessive radiation while the patient is breathing. The patient's breathing pattern is often irregular and unpredictable. We verify the clinical applicability of a heart-sparing robust optimization approach for breast IMRT. We compare robust optimized plans with clinical plans at free-breathing and clinical plans at deep inspiration breath-hold (DIBH) using active breathing control (ABC). Methods: Eight patients were included in the study with each patient simulated using 4D-CT. The 4D-CT image acquisition generated ten breathing phase datasets. An average scan was constructed using all the phase datasets. Two of the eight patients were also imaged at breath-hold using ABC. The 4D-CT datasets were used to calculate the accumulated dose for robust optimized and clinical plans based on deformable registration. We generated a set of simulated breathing probability mass functions, which represent the fraction of time patients spend in different breathing phases. The robust optimization method was applied to each patient using a set of dose-influence matrices extracted from the 4D-CT data and a model of the breathing motion uncertainty. The goal of the optimization models was to minimize the dose to the heart while ensuring dose constraints on the target were achieved under breathing motion uncertainty. Results: Robust optimized plans were improved or equivalent to the clinical plans in terms of heart sparing for all patients studied. The robust method reduced the accumulated heart dose (D10cc) by up to 801 cGy compared to the clinical method while also improving the coverage of the accumulated whole breast target volume. On average, the robust method reduced the heart dose (D10cc) by 364 cGy and improved the optBreast dose (D99%) by 477 cGy. In addition, the robust method had smaller deviations from the planned dose to the accumulated dose. The deviation of the accumulated dose from the planned dose for the optBreast (D99%) was 12 cGy for robust versus 445 cGy for clinical. The deviation for the heart (D10cc) was 41 cGy for robust and 320 cGy for clinical. Conclusions: The robust optimization approach can reduce heart dose compared to the clinical method at free-breathing and can potentially reduce the need for breath-hold techniques. |
14. | Models for predicting objective function weights in prostate cancer IMRT Journal Article J. J. Boutilier, T. Lee, T. Craig, M. B. Sharpe, T. C. Y. Chan In: Medical Physics, vol. 42, pp. 1586-1595, 2015. @article{ChanTCY.J021, Purpose: To develop and evaluate the clinical applicability of advanced machine learning models that simultaneously predict multiple optimization objective function weights from patient geometry for intensity-modulated radiation therapy of prostate cancer. Methods: A previously developed inverse optimization method was applied retrospectively to determine optimal objective function weights for 315 treated patients. The authors used an overlap volume ratio (OV) of bladder and rectum for different PTV expansions and overlap volume histogram slopes (OVSR and OVSB for the rectum and bladder, respectively) as explanatory variables that quantify patient geometry. Using the optimal weights as ground truth, the authors trained and applied three prediction models: logistic regression (LR), multinomial logistic regression (MLR), and weighted K-nearest neighbor (KNN). The population average of the optimal objective function weights was also calculated. Results: The OV at 0.4 cm and OVSR at 0.1 cm features were found to be the most predictive of the weights. The authors observed comparable performance (i.e., no statistically significant difference) between LR, MLR, and KNN methodologies, with LR appearing to perform the best. All three machine learning models outperformed the population average by a statistically significant amount over a range of clinical metrics including bladder/rectum V53Gy, bladder/rectum V70Gy, and dose to the bladder, rectum, CTV, and PTV. When comparing the weights directly, the LR model predicted bladder and rectum weights that had, on average, a 73% and 74% relative improvement over the population average weights, respectively. The treatment plans resulting from the LR weights had, on average, a rectum V70Gy that was 35% closer to the clinical plan and a bladder V70Gy that was 29% closer, compared to the population average weights. Similar results were observed for all other clinical metrics. Conclusions: The authors demonstrated that the KNN and MLR weight prediction methodologies perform comparably to the LR model and can produce clinical quality treatment plans by simultaneously predicting multiple weights that capture trade-offs associated with sparing multiple OARs. |
13. | A robust-CVaR optimization approach with application to breast cancer therapy Journal Article T. C. Y. Chan, H. Mahmoudzadeh, T. G. Purdie In: European Journal of Operational Research, vol. 238, pp. 876-885, 2014. @article{ChanTCY.J020, We present a framework to optimize the conditional value-at-risk (CVaR) of a loss distribution under uncertainty. Our model assumes that the loss distribution is dependent on the state of some system and the fraction of time spent in each state is uncertain. We develop and compare two robust-CVaR formulations that take into account this type of uncertainty. We motivate and demonstrate our approach using radiation therapy treatment planning of breast cancer, where the uncertainty is in the patient's breathing motion and the states of the system are the phases of the patient's breathing cycle. We use a CVaR representation of the tails of the dose distribution to the points in the body and account for uncertainty in the patient's breathing pattern that affects the overall dose distribution. |
12. | Generalized inverse multi-objective optimization with application to cancer therapy Journal Article T. C. Y. Chan, T. Craig, T. Lee, M. B. Sharpe In: Operations Research, vol. 62, pp. 680-695, 2014. @article{ChanTCY.J018, We generalize the standard method of solving inverse optimization problems to allow for the solution of inverse problems that would otherwise be ill posed or infeasible. In multiobjective linear optimization, given a solution that is not a weakly efficient solution to the forward problem, our method generates objective function weights that make the given solution a near-weakly efficient solution. Our generalized inverse optimization model specializes to the standard model when the given solution is weakly efficient and retains the complexity of the underlying forward problem. We provide a novel interpretation of our inverse formulation as the dual of the well-known Benson's method and by doing so develop a new connection between inverse optimization and Pareto surface approximation techniques. We apply our method to prostate cancer data obtained from Princess Margaret Cancer Centre in Toronto, Canada. We demonstrate that clinically acceptable treatments can be generated using a small number of objective functions and inversely optimized weights—current treatments are designed using a complex formulation with a large parameter space in a trial-and-error reoptimization process. We also show that our method can identify objective functions that are most influential in treatment plan optimization. |
11. | A stochastic model for tumor geometry evolution during radiation therapy in cervical cancer Journal Article Y. Liu, T. C. Y. Chan, C.-G. Lee, Y. B. Cho, M. K. Islam In: Medical Physics, vol. 41, no. Article No. 021705, 2014. @article{ChanTCY.J017, Purpose: To develop mathematical models to predict the evolution of tumor geometry in cervical cancer undergoing radiation therapy. Methods: The authors develop two mathematical models to estimate tumor geometry change: a Markov model and an isomorphic shrinkage model. The Markov model describes tumor evolution by investigating the change in state (either tumor or nontumor) of voxels on the tumor surface. It assumes that the evolution follows a Markov process. Transition probabilities are obtained using maximum likelihood estimation and depend on the states of neighboring voxels. The isomorphic shrinkage model describes tumor shrinkage or growth in terms of layers of voxels on the tumor surface, instead of modeling individual voxels. The two proposed models were applied to data from 29 cervical cancer patients treated at Princess Margaret Cancer Centre and then compared to a constant volume approach. Model performance was measured using sensitivity and specificity. Results: The Markov model outperformed both the isomorphic shrinkage and constant volume models in terms of the trade-off between sensitivity (target coverage) and specificity (normal tissue sparing). Generally, the Markov model achieved a few percentage points in improvement in either sensitivity or specificity compared to the other models. The isomorphic shrinkage model was comparable to the Markov approach under certain parameter settings. Convex tumor shapes were easier to predict. Conclusions: By modeling tumor geometry change at the voxel level using a probabilistic model, improvements in target coverage and normal tissue sparing are possible. Our Markov model is flexible and has tunable parameters to adjust model performance to meet a range of criteria. Such a model may support the development of an adaptive paradigm for radiation therapy of cervical cancer. |
10. | Predicting objective function weights from patient anatomy in prostate IMRT treatment planning Journal Article T. Lee, M. Hummad, T. C. Y. Chan, T. Craig, M. B. Sharpe In: Medical Physics, vol. 40, no. Article No. 121706, 2013. @article{ChanTCY.J015, Purpose: Intensity-modulated radiation therapy (IMRT) treatment planning typically combines multiple criteria into a single objective function by taking a weighted sum. The authors propose a statistical model that predicts objective function weights from patient anatomy for prostate IMRT treatment planning. This study provides a proof of concept for geometry-driven weight determination. Methods: A previously developed inverse optimization method (IOM) was used to generate optimal objective function weights for 24 patients using their historical treatment plans (i.e., dose distributions). These IOM weights were around 1% for each of the femoral heads, while bladder and rectum weights varied greatly between patients. A regression model was developed to predict a patient's rectum weight using the ratio of the overlap volume of the rectum and bladder with the planning target volume at a 1 cm expansion as the independent variable. The femoral head weights were fixed to 1% each and the bladder weight was calculated as one minus the rectum and femoral head weights. The model was validated using leave-one-out cross validation. Objective values and dose distributions generated through inverse planning using the predicted weights were compared to those generated using the original IOM weights, as well as an average of the IOM weights across all patients. Results: The IOM weight vectors were on average six times closer to the predicted weight vectors than to the average weight vector, using l_2 distance. Likewise, the bladder and rectum objective values achieved by the predicted weights were more similar to the objective values achieved by the IOM weights. The difference in objective value performance between the predicted and average weights was statistically significant according to a one-sided sign test. For all patients, the difference in rectum V54.3 Gy, rectum V70.0 Gy, bladder V54.3 Gy, and bladder V70.0 Gy values between the dose distributions generated by the predicted weights and IOM weights was less than 5 percentage points. Similarly, the difference in femoral head V54.3 Gy values between the two dose distributions was less than 5 percentage points for all but one patient. Conclusions: This study demonstrates a proof of concept that patient anatomy can be used to predict appropriate objective function weights for treatment planning. In the long term, such geometry-driven weights may serve as a starting point for iterative treatment plan design or may provide information about the most clinically relevant region of the Pareto surface to explore. |
9. | Adaptive and robust radiation therapy optimization for lung cancer Journal Article T. C. Y. Chan, V. V. Mišić In: European Journal of Operational Research, vol. 231, pp. 745-756, 2013. @article{ChanTCY.J012, A previous approach to robust intensity-modulated radiation therapy (IMRT) treatment planning for moving tumors in the lung involves solving a single planning problem before the start of treatment and using the resulting solution in all of the subsequent treatment sessions. In this paper, we develop an adaptive robust optimization approach to IMRT treatment planning for lung cancer, where information gathered in prior treatment sessions is used to update the uncertainty set and guide the reoptimization of the treatment for the next session. Such an approach allows for the estimate of the uncertain effect to improve as the treatment goes on and represents a generalization of existing robust optimization and adaptive radiation therapy methodologies. Our method is computationally tractable, as it involves solving a sequence of linear optimization problems. We present computational results for a lung cancer patient case and show that using our adaptive robust method, it is possible to attain an improvement over the traditional robust approach in both tumor coverage and organ sparing simultaneously. We also prove that under certain conditions our adaptive robust method is asymptotically optimal, which provides insight into the performance observed in our computational study. The essence of our method – solving a sequence of single-stage robust optimization problems, with the uncertainty set updated each time – can potentially be applied to other problems that involve multi-stage decisions to be made under uncertainty. |
8. | Motion-compensating intensity maps in intensity-modulated radiation therapy Journal Article T. C. Y. Chan In: IIE Transactions on Healthcare Systems Engineering, vol. 3, pp. 1-22, 2013. @article{ChanTCY.J008, Managing the effects of tumor motion during radiation therapy is critical to ensuring that a robust treatment is delivered to a cancer patient. Tumor motion due to patient breathing may result in the tumor moving in and out of the beam of radiation, causing the edge of the tumor to be underdosed. One approach to managing the effects of motion is to increase the intensity of the radiation delivered at the edge of the tumor—an edge-enhanced intensity map—which decreases the likelihood of underdosing that area. A second approach is to use a margin, which increases the volume of irradiation surrounding the tumor, also with the aim of reducing the risk of underdosage. In this paper, we characterize the structure of optimal solutions within these two classes of intensity maps. We prove that the ratio of the tumor size to the standard deviation of motion characterizes the structure of an optimal edge-enhanced intensity map. Similar results are derived for a three-dimensional margin case. Furthermore, we extend our analysis by considering a robust version of the problem where the parameters of the underlying motion distribution are not known with certainty, but lie in pre-specified intervals. We show that the robust counterpart of the uncertain 3D margin problem has a very similar structure to the nominal (no uncertainty) problem. |
7. | Optimal margin and edge-enhanced intensity maps in the presence of motion and uncertainty Journal Article T. C. Y. Chan, J. N. Tsitsiklis, T. Bortfeld In: Physics in Medicine and Biology, vol. 55, pp. 515-533, 2010. @article{ChanTCY.J006, In radiation therapy, intensity maps involving margins have long been used to counteract the effects of dose blurring arising from motion. More recently, intensity maps with increased intensity near the edge of the tumour (edge enhancements) have been studied to evaluate their ability to offset similar effects that affect tumour coverage. In this paper, we present a mathematical methodology to derive margin and edge-enhanced intensity maps that aim to provide tumour coverage while delivering minimum total dose. We show that if the tumour is at most about twice as large as the standard deviation of the blurring distribution, the optimal intensity map is a pure scaling increase of the static intensity map without any margins or edge enhancements. Otherwise, if the tumour size is roughly twice (or more) the standard deviation of motion, then margins and edge enhancements are preferred, and we present formulae to calculate the exact dimensions of these intensity maps. Furthermore, we extend our analysis to include scenarios where the parameters of the motion distribution are not known with certainty, but rather can take any value in some range. In these cases, we derive a similar threshold to determine the structure of an optimal margin intensity map. |
6. | Experimental evaluation of a robust optimization method for IMRT of moving targets Journal Article C. Vrančić, A. Trofimov, T. C. Y. Chan, G. C. Sharp, T. Bortfeld In: Physics in Medicine and Biology, vol. 54, pp. 2901-2914, 2009. @article{ChanTCY.J005, Internal organ motion during radiation therapy, if not considered appropriately in the planning process, has been shown to reduce target coverage and increase the dose to healthy tissues. Standard planning approaches, which use safety margins to handle intrafractional movement of the tumor, are typically designed based on the maximum amplitude of motion, and are often overly conservative. Comparable coverage and reduced dose to healthy organs appear achievable with robust motion-adaptive treatment planning, which considers the expected probability distribution of the average target position and the uncertainty of its realization during treatment delivery. A dosimetric test of a robust optimization method for IMRT was performed, using patient breathing data. External marker motion data acquired from respiratory-gated radiotherapy patients were used to build and test the framework for robust optimization. The motion trajectories recorded during radiation treatment itself are not strictly necessary to generate the initial version of a robust treatment plan, but can be used to adapt the plan during the course of treatment. Single-field IMRT plans were optimized to deliver a uniform dose to a rectangular area. During delivery on a linear accelerator, a computer-driven motion phantom reproduced the patients' breathing patterns and a two-dimensional ionization detector array measured the dose delivered. The dose distributions from robust-optimized plans were compared to those from standard plans, which used a margin expansion. Dosimetric tests confirmed the improved sparing of the non-target area with robust planning, which was achieved without compromising the target coverage. The maximum dose in robust plans did not exceed 110% of the prescription, while the minimum target doses were comparable in standard and robust plans. In test courses, optimized for a simplified target geometry, and delivered to a phantom that moved in one dimension with an average amplitude of 17 mm, the robust treatment design produced a reduction of more than 12% of the integral dose to non-target areas, compared to the standard plan using 10 mm margin expansion. |
5. | Robust management of motion uncertainty in intensity modulated radiation therapy Journal Article T. Bortfeld, T. C. Y. Chan, A. Trofimov, J. N. Tsitsiklis In: Operations Research, vol. 56, pp. 1461-1473, 2008. @article{ChanTCY.J004, Radiation therapy is subject to uncertainties that need to be accounted for when determining a suitable treatment plan for a cancer patient. For lung and liver tumors, the presence of breathing motion during treatment is a challenge to the effective and reliable delivery of the radiation. In this paper, we build a model of motion uncertainty using probability density functions that describe breathing motion, and provide a robust formulation of the problem of optimizing intensity-modulated radiation therapy. We populate our model with real patient data and measure the robustness of the resulting solutions on a clinical lung example. Our robust framework generalizes current mathematical programming formulations that account for motion, and gives insight into the trade-off between sparing the healthy tissues and ensuring that the tumor receives sufficient dose. For comparison, we also compute solutions to a nominal (no uncertainty) and margin (worst-case) formulation. In our experiments, we found that the nominal solution typically underdosed the tumor in the unacceptable range of 6% to 11%, whereas the robust solution underdosed by only 1% to 2% in the worst case. In addition, the robust solution reduced the total dose delivered to the main organ-at-risk (the left lung) by roughly 11% on average, as compared to the margin solution. |
4. | Tumor trailing strategy for intensity-modulated radiation therapy of moving targets Journal Article A. Trofimov, C. Vrancic, T. C. Y. Chan, G. C. Sharp, T. Bortfeld In: Medical Physics, vol. 35, pp. 1718-1733, 2008. @article{ChanTCY.J003, Internal organ motion during the course of radiation therapy of cancer affects the distribution of the delivered dose and, generally, reduces its conformality to the targeted volume. Previously proposed approaches aimed at mitigating the effect of internal motion in intensity-modulated radiation therapy (IMRT) included expansion of the target margins, motion-correlated delivery (e.g., respiratory gating, tumor tracking), and adaptive treatment plan optimization employing a probabilistic description of motion. We describe and test the tumor trailing strategy, which utilizes the synergy of motion-adaptive treatment planning and delivery methods. We regard the (rigid) target motion as a superposition of a relatively fast cyclic component (e.g., respiratory) and slow aperiodic trends (e.g., the drift of exhalation baseline). In the trailing approach, these two components of motion are decoupled and dealt with separately. Real-time motion monitoring is employed to identify the 'slow' shifts, which are then corrected by applying setup adjustments. The delivery does not track the target position exactly, but trails the systematic trend due to the delay between the time a shift occurs, is reliably detected, and, subsequently, corrected. The 'fast' cyclic motion is accounted for with a robust motion-adaptive treatment planning, which allows for variability in motion parameters (e.g., mean and extrema of the tidal volume, variable period of respiration, and expiratory duration). Motion-surrogate data from gated IMRT treatments were used to provide probability distribution data for motion-adaptive planning and to test algorithms that identified systematic trends in the character of motion. Sample IMRT fields were delivered on a clinical linear accelerator to a programmable moving phantom. Dose measurements were performed with a commercial two-dimensional ion-chamber array. The results indicate that by reducing intrafractional motion variability, the trailing strategy enhances relevance and applicability of motion-adaptive planning methods, and improves conformality of the delivered dose to the target in the presence of irregular motion. Trailing strategy can be applied to respiratory-gated treatments, in which the correction for the slow motion can increase the duty cycle, while robust probabilistic planning can improve management of the residual motion within the gate window. Similarly, trailing may improve the dose conformality in treatment of patients who exhibit detectable target motion of low amplitude, which is considered insufficient to provide a clinical indication for the use of respiratory-gated treatment (e.g., peak-to-peak motion of less than 10 mm). The mechanical limitations of implementing tumor trailing are less rigorous than those of real-time tracking, and the same technology could be used for both. |
3. | Optimization under uncertainty in radiation therapy PhD Thesis T. C. Y. Chan Sloan School of Management, MIT, 2007. @phdthesis{Chan2007, In the context of patient care for life-threatening illnesses, the presence of uncertainty may compromise the quality of a treatment. In this thesis, we investigate robust approaches to managing uncertainty in radiation therapy treatments for cancer. In the first part of the thesis, we study the effect of breathing motion uncertainty on intensity-modulated radiation therapy treatments of a lung tumor. We construct a robust framework that generalizes current mathematical programming formulations that account for motion. This framework gives insight into the trade-off between sparing the healthy tissues and ensuring that the tumor receives sufficient dose. With this trade-off in mind, we show that our robust solution outperforms a nominal (no uncertainty) solution and a margin (worst-case) solution on a clinical case. Next, we perform an in-depth study into the structure of different intensity maps that were witnessed in the first part of the thesis. We consider parameterized intensity maps and investigate their ability to deliver a sufficient dose to the tumor in the presence of motion that follows a Gaussian distribution. We characterize the structure of optimal intensity maps in terms of certain conditions on the problem parameters. Finally, in the last part of the thesis, we study intensity-modulated proton therapy under uncertainty in the location of maximum dose deposited by the beamlets of radiation. We provide a robust formulation for the optimization of proton-based treatments and show that it outperforms traditional formulations in the face of uncertainty. In our computational experiments, we see evidence that optimal robust solutions use the physical characteristics of the proton beam to create dose distributions that are far less sensitive to the underlying uncertainty. |
2. | Accounting for range uncertainties in the optimization of intensity modulated proton therapy Journal Article J. Unkelbach, T. C. Y. Chan, T. Bortfeld In: Physics in Medicine and Biology, vol. 52, pp. 2755-2773, 2007. @article{ChanTCY.J002, Treatment plans optimized for intensity modulated proton therapy (IMPT) may be sensitive to range variations. The dose distribution may deteriorate substantially when the actual range of a pencil beam does not match the assumed range. We present two treatment planning concepts for IMPT which incorporate range uncertainties into the optimization. The first method is a probabilistic approach. The range of a pencil beam is assumed to be a random variable, which makes the delivered dose and the value of the objective function a random variable too. We then propose to optimize the expectation value of the objective function. The second approach is a robust formulation that applies methods developed in the field of robust linear programming. This approach optimizes the worst case dose distribution that may occur, assuming that the ranges of the pencil beams may vary within some interval. Both methods yield treatment plans that are considerably less sensitive to range variations compared to conventional treatment plans optimized without accounting for range uncertainties. In addition, both approaches—although conceptually different—yield very similar results on a qualitative level. |
1. | A robust approach to IMRT optimization Journal Article T. C. Y. Chan, T. Bortfeld, J. N. Tsitsiklis In: Physics in Medicine and Biology, vol. 51, pp. 2567-2583, 2006. @article{ChanTCY.J001, Managing uncertainty is a major challenge in radiation therapy treatment planning, including uncertainty induced by intrafraction motion, which is particularly important for tumours in the thorax and abdomen. Common methods to account for motion are to introduce a margin or to convolve the static dose distribution with a motion probability density function. Unlike previous work in this area, our development does not assume that the patient breathes according to a fixed distribution, nor is the patient required to breathe the same way throughout the treatment. Despite this generality, we create a robust optimization framework starting from the convolution method that is robust to fluctuations in breathing motion, yet spares healthy tissue better than a margin solution. We describe how to generate the data for our model using breathing motion data and we test our model on a computer phantom using data from real patients. In our numerical results, the robust solution delivers approximately 38% less dose to the healthy tissue than the margin solution, while providing the same level of protection against breathing uncertainty. |
Cardiac Arrest and Public Access Defibrillator Location
The likelihood of survival from cardiac arrest is very time sensitive. One method to reset the heart’s normal rhythm is to deliver an electric shock using an automated external defibrillator (AED), which can be used by lay responders with little to no training. My research focuses on developing optimization methods for AED deployment, ranging from static deployment in public locations to delivery via drone. Accordingly, I am interested in approaches to estimate cardiac arrest risk and quantify accessibility.
RELEVANT PUBLICATIONS
33. | Cost-effectiveness of drone-delivered automated external defibrillators for out-of-hospital cardiac arrest Journal Article Forthcoming M. Maaz, K. H. B. Leung, J. J. Boutilier, S. Suen, P. Dorian, L. J. Morrison, D. Scales, S. Cheskes, T. C. Y. Chan In: Resuscitation, Forthcoming. @article{ChanTCY.J134, Background: Out-of-hospital cardiac arrest (OHCA) is a significant cause of mortality and morbidity in North America, for which timely defibrillation of shockable rhythms is essential. Drones have been proposed as an intervention to improve response time and are being implemented in practice. Aim: To determine the cost-effectiveness of drone-delivered automated external defibrillators (AEDs) for OHCAs. Methods: Using data from 22,017 OHCAs in Ontario, Canada over 10 years, we developed a comprehensive computational framework combining machine learning, optimization and a Markov microsimulation model to provide an economic evaluation of 964 different drone networks across a wide range of sizes and configurations. We simulated response times, survival outcomes, lifetime quality-adjusted life-years (QALYs), lifetime healthcare costs, and 10-year operational costs for each network. Results: All 964 drone networks were cost-effective. We identified 20 networks on the cost-QALY efficient frontier, each with shorter response times, more survivors across all categories, and higher costs per survivor. Historical ambulance response (i.e., standard care) had mean response time of 6 min 21 s. On the efficient frontier, average drone response times were 32% to 71% shorter than standard care. There were 1,855 (8.4%) survivors to hospital discharge in standard care, which increased by 21% to 46% across the 20 drone networks. The smallest non-dominated drone network, with 20 drones, cost $20,912 per QALY gained. All drone networks had higher net monetary benefit than standard care. Cost-effectiveness was even greater for shockable and witnessed populations. Extensive sensitivity analyses showed that our results were robust to changes in modelling assumptions. Conclusions: Drone-delivered AEDs were associated with reductions in response time, mortality and morbidity, and were found to be highly cost-effective relative to standard ambulance response with no drones. |
32. | Optimisation of the deployment of automated external defibrillators in public places in England Journal Article T. P. Brown, L. Andronis, A. El-Banna, B. K. H. Leung, T. N. Arvanitis, C. Deakin, A. N. Siriwardena, J. Long, G. Clegg, S. Brooks, T. C. Y. Chan, S. Irving, L. Walker, C. Mortimer, S. Igbodo, G. D. Perkins In: Health and Social Care Delivery Research, vol. 13, iss. 5, 2025. @article{ChanTCY.J129, Background: Ambulance services treat over 32,000 patients sustaining an out-of-hospital cardiac arrest annually, receiving over 90,000 calls. The definitive treatment for out-of-hospital cardiac arrest is defibrillation. Prompt treatment with an automated external defibrillator can improve survival significantly. However, their location in the community limits opportunity for their use. There is a requirement to identify the optimal location for an automated external defibrillator to improve out-of-hospital cardiac arrest coverage, to improve the chances of survival. Methods: This was a secondary analysis of data collected by the Out-of-Hospital Cardiac Arrest Outcomes registry on historical out-of-hospital cardiac arrests, data held on the location of automated external defibrillators registered with ambulance services, and locations of points of interest. Walking distance was calculated between out-of-hospital cardiac arrests, registered automated external defibrillators and points of interest designated as potential sites for an automated external defibrillator. An out-of-hospital cardiac arrest was deemed to be covered if it occurred within 500 m of a registered automated external defibrillator or points of interest. For the optimisation analysis, mathematical models focused on the maximal covering location problem were adapted. A de novo decision-analytic model was developed for the cost-effectiveness analysis and used as a vehicle for assessing the costs and benefits (in terms of quality-adjusted life-years) of deployment strategies. A meeting of stakeholders was held to discuss and review the results of the study. Results: Historical out-of-hospital cardiac arrests occurred in more deprived areas and automated external defibrillators were placed in more affluent areas. The median out-of-hospital cardiac arrest – automated external defibrillator distance was 638 m and 38.9% of out-of-hospital cardiac arrests occurred within 500 m of an automated external defibrillator. If an automated external defibrillator was placed in all points of interests, the proportion of out-of-hospital cardiac arrests covered varied greatly. The greatest coverage was achieved with cash machines. Coverage loss, assuming an automated external defibrillator was not available outside working hours, varied between points of interest and was greatest for schools. Dividing the country up into 1 km2 grids and placing an automated external defibrillator in the centre increased coverage significantly to 78.8%. The optimisation model showed that if automated external defibrillators were placed in each points-of-interest location out-of-hospital cardiac arrest coverage levels would improve above the current situation significantly, but it would not reach that of optimisation-based placement (based on grids). The coverage efficiency provided by the optimised grid points was unmatched by any points of interest in any region. An economic evaluation determined that all alternative placements were associated with higher quality-adjusted life-years and costs compared to current placement, resulting in incremental cost-effectiveness ratios over £30,000 per additional quality-adjusted life-year. The most appealing strategy was automated external defibrillator placement in halls and community centres, resulting in an additional 0.007 quality-adjusted life-year (non-parametric 95% confidence interval 0.004 to 0.011), an additional expected cost of £223 (non-parametric 95% confidence interval £148 to £330) and an incremental cost-effectiveness ratio of £32,418 per quality-adjusted life-year. The stakeholder meeting agreed that the current distribution of registered publicly accessible automated external defibrillators was suboptimal, and that there was a disparity in their location in respect of deprivation and other health inequalities. Conclusions: We have developed a data-driven framework to support decisions about public-access automated external defibrillator locations, using optimisation and statistical models. Optimising automated external defibrillator locations can result in substantial improvement in coverage. Comparison between placement based on points of interest and current placement showed that the former improves coverage but is associated with higher costs and incremental cost-effectiveness ratio values over £30,000 per additional quality-adjusted life-year. |
31. | Centre-specific variation in atrial fibrillation ablation rates in a universal single-payer health care system Journal Article C. Seo, S. Kushwaha, P. Angaran, P. Gozdyra, K. S. Allan, H. Abdel-Qadir, P. Dorian, T. C. Y. Chan In: CJC Open, vol. 6, no. 11, pp. 1355-1362, 2024. @article{ChanTCY.J0122, Background Disparities in atrial fibrillation ablation rates have been previously studied, with focuses on patient characteristics and systems factors rather than geographic factors. The impact of electrophysiology centre practice patterns on ablation rates have not been well studied. Methods This was a population-based cohort study using linked administrative datasets covering physician billing codes, hospitalizations, prescriptions, and census data. The study population consisted of patients visiting an emergency department with a new diagnosis of atrial fibrillation between 2007-2016, in Ontario, Canada. Patient characteristics, including age, sex, medical history, comorbidities, socioeconomic factors, closest electrophysiology centre within 20 km, and distance to nearest centre were used as predictors in multivariable logistic regression models to assess the relationship between living around specific electrophysiology centres and ablation rates. Results The cohort included 134,820 patients of whom 9,267 had an ablation during the study period. Patients undergoing ablation were younger, had a lower CHADS2 score, lived closer to electrophysiology centres, and had fewer comorbidities than people who did not receive ablation. There was wide variation in ablation rates, with adjacent census divisions having up to 2.6 times higher ablation rate. Multivariate regression revealed significant differences in ablation rates for patients living around certain electrophysiology centres. The odds ratios for living closest to specific centres ranged from 0.78 (95% CI: 0.68-0.89) to 1.60 (95% CI:1.34-1.90). Conclusions Living near specific electrophysiology centres may significantly affect a patient’s likelihood of ablation, regardless of factors such as age, gender, socioeconomic status, prior medical history, and distance to electrophysiology centres. |
30. | K. H. B. Leung, L. Hartley, L. Moncur, S. Gillon, S. Short, T. C. Y. Chan, G. R. Clegg In: Resuscitation, vol. 200, pp. 110256, 2024. @article{ChanTCY.J0119, Background: Extracorporeal cardiopulmonary resuscitation (ECPR) can improve survival for refractory out-of-hospital cardiac arrest (OHCA). We sought to assess the feasibility of a proposed ECPR programme in Scotland, considering both in-hospital and pre-hospital implementation scenarios. Methods: We included treated OHCAs in Scotland aged 16-70 between August 2018 and March 2022. We defined those clinically eligible for ECPR as patients where the initial rhythm was ventricular fibrillation, ventricular tachycardia, or pulseless electrical activity, and where pre-hospital return of spontaneous circulation was not achieved. We computed the call-to-ECPR access time interval as the amount of time from emergency medical service (EMS) call reception to either arrival at an ECPR-ready hospital or arrival of a pre-hospital ECPR crew. We determined the number of patients that had access to ECPR within 45 minutes, and estimated the number of additional survivors as a result. Results: A total of 6,639 OHCAs were included in the geospatial modelling, 1,406 of which were eligible for ECPR. Depending on the implementation scenario, 52.9-112.6 (13.8-29.4%) OHCAs per year had a call-to-ECPR access time within 45 minutes, with pre-hospital implementation scenarios having greater and earlier access to ECPR for OHCA patients. We further estimated that an ECPR programme in Scotland would yield 11.8-28.2 additional survivors per year, with the pre-hospital implementation scenarios yielding higher numbers. Conclusion: An ECPR programme for OHCA in Scotland could provide access to ECPR to a modest number of eligible OHCA patients, with pre-hospital ECPR implementation scenarios yielding higher access to ECPR and higher numbers of additional survivors. |
29. | Combinations of first responder and drone delivery to achieve 5-minute AED deployment in OHCA Journal Article M. A. Starks, J. Chu, K. H. B. Leung, A. L. Blewer, D. Simmons, C. Malta Hansen, A. Joiner, J. G. Cabañas, M. R. Harmody, R. D. Nelson, B. F. McNally, J. P. Ornato, C. B. Granger, T. C. Y. Chan, D. B. Mark In: JACC: Advances, vol. 3, no. 7, pp. 101033, 2024. @article{ChanTCY.J0118, BACKGROUND Defibrillation in the critical first minutes of out-of-hospital cardiac arrest (OHCA) can significantly improve survival. However, timely access to automated external defibrillators (AEDs) remains a barrier. OBJECTIVES The authors estimated the impact of a statewide program for drone-delivered AEDs in North Carolina integrated into emergency medical service and first responder (FR) response for OHCA. METHODS Using Cardiac Arrest Registry to Enhance Survival registry data, we included 28,292 OHCA patients $18 years of age between 1 January 2013 and 31 December 2019 in 48 North Carolina counties. We estimated the improvement in response times (time from 9-1-1 call to AED arrival) achieved by 2 sequential interventions: 1) AEDs for all FRs; and 2) optimized placement of drones to maximize 5-minute AED arrival within each county. Interventions were evaluated with logistic regression models to estimate changes in initial shockable rhythm and survival. RESULTS Historical county-level median response times were 8.0 minutes (IQR: 7.0-9.0 minutes) with 16.5% of OHCAs having AED arrival times of <5 minutes (IQR: 11.2%-24.3%). Providing all FRs with AEDs improved median response to 7.0 minutes (IQR: 6.2-7.8 minutes) and increased OHCAs with <5-minute AED arrival to 22.3% (IQR: 16.4%-30.9%). Further incorporating optimized drone networks (326 drones across all 48 counties) improved median response to 4.8 minutes (IQR: 4.3-5.2 minutes) and OHCAs with <5-minute AED arrival to 56.3% (IQR: 46.9%-64.2%). Survival rates were estimated to increase by 34% for witnessed OHCAs with estimated drone arrival <5 minutes and ahead of FR and emergency medical service. CONCLUSIONS Deployment of AEDs by FRs and optimized drone delivery can improve AED arrival times which may lead to improved clinical outcomes. Implementation studies are needed. |
28. | M. Heidet, K. H. B. Leung, W. Bougouin, R. Alam, B. Frattini, D. Liang, D. Jost, V. Canon, J. Deakin, H. Hubert, J. Christenson, B. Vivien, T. Chan, A. Cariou, F. Dumas, X. Jouven, E. Marijon, S. Bennington, S. Travers, S. Souihi, B. Grunau In: Resuscitation, vol. 193, pp. 109995, 2023. @article{ChanTCY.J0110, |
27. | L. D. Liang, K. H. B. Leung, T. C. Y. Chan, J. Deakin, M. Heidet, G. Meckler, F. Scheuermeyer, S. Sanatani, J. Christenson, B. Grunau In: Resuscitation, vol. 181, pp. 20-25, 2022. @article{ChanTCY.J102, Background: Systematic automated external defibrillator (AED) placement in schools may improve pediatric out-of-hospital cardiac arrest (OHCA) survival. To estimate their utility, we identified school-located pediatric and adult OHCAs to estimate the potential utilization of school-located AEDs. Further, we identified all OHCAs within an AED-retrievable distance of the school by walking, biking, and driving. Methods: We used prospectively collected data from the British Columbia(BC) Cardiac Arrest Registry (2013–2020), and geo-plotted all OHCAs and schools (n = 824) in BC. We identified adult and pediatric (age < 18 years) OHCAs occurring in schools, as well as nearby OHCAs for which a school-based externally-placed AED could be retrieved by a bystander prior to emergency medical system (EMS) arrival. Results: Of 16,409 OHCAs overall in the study period, 28.6 % occurred during school hours. There were 301 pediatric OHCAs. 5 (1.7 %) occurred in schools, of whom 2 (40 %) survived to hospital discharge. Among both children and adults, 28 (0.17 %) occurred in schools(0.0042/school/year), of whom 21 (75 %) received bystander resuscitation, 4 (14 %) had a bystander AED applied, and 14(50 %) survived to hospital discharge. For each AED, an average of 0.29 OHCAs/year (95 % CI 0.21–0.37), 0.93 OHCAs/year (95 % CI 0.69–1.56) and 1.69 OHCAs/year (95 % CI 1.21–2.89) would be within the potential retrieval distance of a school-located AED by pedestrian, cyclist and automobile retrieval, respectively, using the median EMS response times. Conclusion: While school-located OHCAs were uncommon, outcomes were favourable. 11.1% to 60.9% of all OHCAs occur within an AED-retrievable distance to a school, depending on retrieval method. Accessible external school-located AEDs may improve OHCA outcomes of school children and in the surrounding community. |
26. | Optimizing defibrillator deployment Book Chapter T. C. Y. Chan, C. L. F. Sun In: Suen, S.; Scheinker, D.; Enns, E. (Ed.): Artificial Intelligence for Healthcare: Interdisciplinary Partnerships for Analytics-driven Improvements in a Post-COVID World, Chapter 6, pp. 110-140, Cambridge University Press, Cambridge, 2022, ISBN: 978-1108872188. @inbook{ChanTCY.Oth011, In this chapter, we explore how data-driven modeling can improve the understanding of OHCA risk, help identify the limitations of current AED placement strategies, and guide the development of optimal AED networks to increase the chance of AED use and OHCA survival. More specifically, we frame AED network design and related response efforts as a facility location problem, focusing on the maximum coverage location and p-median problems. We also highlight how novel tools that combine techniques from areas including information theory and machine learning with optimization models can shape the future of OHCA response efforts and AED placement strategies. |
25. | Incremental gains in response time with varying base location types for drone-delivered automated external defibrillators Journal Article K. H. B. Leung, B. Grunau, R. Al Assil, M. Heidet, L. D. Liang, J. Deakin, J. Christenson, S. Cheskes, T. C. Y. Chan In: Resuscitation, vol. 174, pp. 24-30, 2022. @article{ChanTCY.J097, Introduction: Drone-delivered automated external defibrillators (AEDs) may reduce delays to defibrillation for out-of-hospital cardiac arrests (OHCAs). We sought to determine how integration of drones and selection of drone bases between emergency service stations (i.e., paramedic, fire, police) would affect 9-1-1 call-to-arrival intervals. Methods: We identified all treated OHCAs in southern Vancouver Island, British Columbia, Canada from Jan. 2014 to Dec. 2020. We developed mathematical models to select 1–5 optimal drone base locations from each of: paramedic stations, fire stations, police stations, or an unrestricted grid-based set of points to minimize drone travel time to OHCAs. We evaluated models on the estimated first response interval assuming that drones were integrated with existing OHCA response. We compared median response intervals with historical response, as well as across drone base locations. Results: A total of 1610 OHCAs were included in the study with a historical median response interval of 6.4 minutes (IQR 5.0–8.6). All drone- integrated response systems significantly reduced the median response interval to 4.2–5.4 minutes (all P < 0.001), with grid-based stations using 5 drones resulting in the lowest response interval (4.2 minutes). Median response times between drone base location types differed by 6–16 seconds, all comparisons of which were statistically significant (all P < 0.02). Conclusion: Integrating drone-delivered AEDs into OHCA response may reduce first response intervals, even with a small quantity of drones. Implementing drone response with only one emergency service resulted in similar response metrics regardless of the emergency service hosting the drone base and was competitive with unrestricted drone base locations. |
24. | Drone network design for cardiac arrest response Journal Article J. J. Boutilier, T. C. Y. Chan In: Manufacturing and Service Operations Management, vol. 24, no. 5, pp. 2407-2424, 2022. @article{ChanTCY.J096, Problem definition: Our objective is to design a defibrillator-enabled drone network that augments the existing emergency medical services (EMS) system to rapidly respond to out-of-hospital cardiac arrest (OHCA). Academic/practical relevance: OHCA claims more than 400,000 lives each year in North America and is one of the most time-sensitive medical emergencies. Drone-delivered automated external defibrillators (AEDs) have the potential to be a transformative innovation in the provision of emergency care for OHCA. Methodology: We develop an integrated location-queuing model that incorporates existing EMS response times and is based on the p-median architecture, where each base constitutes an explicit M/M/d/d queue (i.e., Erlang loss). We then develop a reformulation technique that exploits the existing EMS response times, allowing us to solve real-world instances to optimality using an off-the-shelf solver. We evaluate our solutions using a tactical simulation model that accounts for the effects of congestion and dispatching, and we use a machine-learning model to translate our response-time reductions into survival estimates. Results:Using real data from an area covering 26,000 square kilometers around Tor-onto, Canada, we find that a modest number of drones are required to significantly reduce response times in all regions. An objective function that minimizes average response time results in drone resources concentrated in cities, with little impact on the tail of the distribution. In contrast, optimizing for the tail of the response-time distribution produces larger and more geographically dispersed drone networks that improve response-time equity across the regions. We estimate that the response-time reductions achieved by the drone network are associated with between a 42% and 76% higher survival rate and up to 144 additional lives saved each year across the geographical region we consider. Managerial implications: Overall, this paper provides a realistic framework that can be leveraged by system designers and/or EMS personnel seeking to investigate design questions associated with a drone network. An objective function focused on improving the tail of the response-time distribution is well-suited for use in practice because the model provides equitable solutions that reduce the entire response-time distribution and corresponds to the real-world metrics, on which EMS systems are most commonly evaluated. |
23. | Utilization and cost-effectiveness of school and community center AED deployment models in Canadian cities Journal Article L. D. Liang, T. C. Y. Chan, K. H. B. Leung, F. Scheuermeyer, S. Chakrabarti, L. Andelius, J. Deakin, M. Heidet, C. B. Fordyce, J. Helmer, J. Christenson, R. Al Assil, B. Grunau In: Resuscitation, vol. 172, pp. 194-200, 2022. @article{ChanTCY.J094, Background: The optimal locations and cost-effectiveness of placing automated external defibrillators (AEDs) for out-of-hospital cardiac arrest (OHCAs) in urban residential neighbourhoods are unclear. Methods: We used prospectively collected data from 2016 to 2018 from the British Columbia OHCA Registry to examine the utilization and cost- effectiveness of hypothetical AED deployment in municipalities with a population of over 100 000. We geo-plotted OHCA events using seven hypothetical deployment models where AEDs were placed at the exteriors of public schools and community centers and fetched by bystanders. We calculated the “radius of effectiveness” around each AED within which it could be retrieved and applied to an individual prior to EMS arrival, comparing automobile and pedestrian-based retrieval modes. For each deployment model, we estimated the number of OHCAs within the “radius of effectiveness”. Results: We included 4017 OHCAs from ten urban municipalities. The estimated radius of effectiveness around each AED was 625 m for auto- mobile and 240 m for pedestrian retrieval. With AEDs placed outside each school and community center, 2567 (64%) and 605 (15%) of OHCAs fell within the radii of effectiveness for automobile and pedestrian retrieval, respectively. For each AED, there was an average of 1.20–2.66 and 0.25–0.61 in-range OHCAs per year for automobile retrieval and pedestrian retrieval, respectively, depending on the deployment model. All of our proposed surpassed the cost-effectiveness threshold of 0.125 OHCA/AED/year provided > 5.3–11.6% in-range AEDs were brought-to-scene. Conclusions: The systematic deployment of AEDs at schools and community centers in urban neighbourhoods may result in increased application and be a cost-effective public health intervention. |
22. | Moderating effects of out-of-hospital cardiac arrest characteristics on the association between EMS response time and survival Journal Article C. E. Stoesser, J. J. Boutilier, C. L. F. Sun, S. C. Brooks, S. Cheskes, K. N. Dainty, M. Feldman, D. T. Ko, S. Lin, L. J. Morrison, D. C. Scales, T. C. Y. Chan In: Resuscitation, vol. 169, pp. 31-38, 2021. @article{ChanTCY.J087, Background Although several Utstein variables are known to independently improve survival, how they moderate the effect of emergency medical service (EMS) response times on survival is unknown. Objectives To quantify how public location, witnessed status, bystander CPR, and bystander AED shock individually and jointly moderate the effect of EMS response time delays on OHCA survival. Methods This retrospective cohort study was a secondary analysis of the Resuscitation Outcomes Consortium Epistry-Cardiac Arrest database (December 2005 to June 2015). We included all adult, non-traumatic, non-EMS witnessed, and EMS-treated OHCAs from eleven sites across the US and Canada. We trained a logistic regression model with standard Utstein control variables and interaction terms between EMS response time and the four aforementioned OHCA characteristics. Results 102,216 patients were included. Three of the four characteristics – witnessed OHCAs (OR=0.962), bystander CPR (OR=0.968) and public location (OR=0.980) – increased the negative effect of a one-minute delay on the odds of survival. In contrast, a bystander AED shock decreased the negative effect of a one-minute response time delay on the odds of survival (OR=1.064). The magnitude of the effect of a one-minute delay in EMS response time on the odds of survival ranged from 1.3% to 9.8% (average: 5.3%), depending on the underlying OHCA characteristics. Conclusions Delays in EMS response time had the largest reduction in survival odds for OHCAs that did not receive a bystander AED shock but were witnessed, occurred in public, and/or received bystander CPR. A bystander AED shock appears to be protective against a delay in EMS response time. |
21. | Implications for cardiac arrest coverage using straight-line versus route distance to nearest automated external defibrillator Journal Article L. Karlsson, C. L. F. Sun, C. Torp-Pedersen, K. Wodschow, A. K. Ersbøll, M. Wissenberg, C. M. Hansen, L. J. Morrison, T. C. Y. Chan, F. Folke In: Resuscitation, vol. 167, pp. 326–335, 2021. @article{ChanTCY.J086, Aim Quantifying the ratio describing the difference between “true route” and “straight-line” distances from out-of-hospital cardiac arrests (OHCAs) to the closest accessible automated external defibrillator (AED) can help correct likely overestimations in AED coverage. Furthermore, we aimed to examine to what extent the closest AED based on true route distance differed from the closest AED using “straight-line”. Methods OHCAs (1994-2016) and AEDs (2016) in Copenhagen, Denmark and in Toronto, Canada (2007-2015 and 2015, respectively) were identified. Three distances were calculated between OHCA and target AED: 1) the straight-line distance (“straight-line”) to the closest AED, 2) the corresponding true route distance to the same AED (“true route”), and 3) the closest AED based only on true route distance (“shortest true route”). The ratio between “true route” and “straight-line” distance was calculated and differences in AED coverage (an OHCA ≤100m of an accessible AED) were examined. Results The “straight-line” AED coverage of 100m was 24.2% (n=2008/8295) in Copenhagen and 6.9% (n=964/13916) in Toronto. The corresponding “true route” distance reduced coverage to 9.5% (n=786) and 3.8% (n=529), respectively. The median ratio between “true route” and “straight-line” distance was 1.6 in Copenhagen and 1.4 in Toronto. In 26.1% (n=2167) and 22.9% (n=3181) of all Copenhagen and Toronto OHCAs respectively, the closest AED in “shortest true route” was different than the closest AED initially found by ”straight-line”. Conclusions Straight-line distance is not an accurate measure of distance and overestimates the actual AED coverage compared to a more realistic true route distance by a factor 1.4-1.6. |
20. | Socioeconomically equitable public defibrillator placement using mathematical optimization Journal Article K. H. B. Leung, S. C. Brooks, G. R. Clegg, T. C. Y. Chan In: Resuscitation, vol. 166, pp. 14-20, 2021. @article{ChanTCY.J085, Background: Mathematical optimization can be used to place automated external defibrillators (AEDs) in locations that maximize coverage of out-of-hospital cardiac arrests (OHCAs). We sought to determine whether optimization can improve alignment between AED locations and OHCA counts across levels of socioeconomic deprivation. Methods: All suspected OHCAs and registered AEDs in Scotland between Jan. 2011 and Sept. 2017 were included and mapped to a corresponding socioeconomic deprivation level using the Scottish Index of Multiple Deprivation (SIMD). We used mathematical optimization to determine optimal locations for placing 10%, 25%, 50%, and 100% additional AEDs, as well as locations for relocating existing AEDs. For each AED placement policy, we examined the impact on AED distribution and OHCA “coverage” (suspected OHCA occurring within 100 m of AED) with respect to SIMD quintiles. Results: We identified 49,432 suspected OHCAs and 1532 AEDs. The distribution of existing AED locations across SIMD quintiles significantly diered from the distribution of suspected OHCAs (P < 0.001). Optimization-guided AED placement increased coverage of suspected OHCAs compared to existing AED locations (all P < 0.001). Optimization resulted in more AED placements and increased OHCA coverage in areas of greater socioeconomic deprivation, such that resulting distributions across SIMD quintiles matched the shape of the OHCA count distribution. Optimally relocating existing AEDs achieved similar OHCA coverage levels to that of doubling the number of total AEDs. Conclusions: Mathematical optimization results in AED locations and suspected OHCA coverage that more closely resembles the suspected OHCA distribution and results in more equitable coverage across levels of socioeconomic deprivation. |
19. | Machine learning-based dispatch of drone-delivered defibrillators for out-of-hospital cardiac arrest Journal Article J. Chu, K. H. B. Leung, P. Snobelen, G. Nevils, I. R. Drennan, S. Cheskes, T. C. Y. Chan In: Resuscitation, vol. 162, pp. 120-127, 2021. @article{ChanTCY.J083, Background: Drone-delivered defibrillators have the potential to significantly reduce response time for out-of-hospital cardiac arrest (OHCA). However, optimal policies for the dispatch of such drones are not yet known. We sought to develop dispatch rules for a network of defibrillator-carrying drones. Methods: We identified all suspected OHCAs in Peel Region, Ontario, Canada from Jan. 2015 to Dec. 2019. We developed drone dispatch rules based on the difference between a predicted ambulance response time to a calculated drone response time for each OHCA. Ambulance response times were predicted using linear regression and neural network models, while drone response times were calculated using drone specifications from recent pilot studies and the literature. We evaluated the dispatch rules based on response time performance and dispatch decisions, comparing them to two baseline policies of never dispatching and always dispatching drones. Results: A total of 3573 suspected OHCAs were included in the study with median and mean historical ambulance response times of 5.8 and 6.2 min. All machine learning-based dispatch rules significantly reduced the median response time to 3.9min and mean response time to 4.14.2min (all P < 0.001) and were non-inferior to universally dispatching drones (all P < 0.001) while reducing the number of drone flights by up to 30%. Dispatch rules with more drone flights achieved higher sensitivity but lower specificity and accuracy. Conclusion: Machine learning-based dispatch rules for drone-delivered defibrillators can achieve similar response time reductions as universal drone dispatch while substantially reducing the number of trips. |
18. | Public defibrillator accessibility and mobility trends during the COVID-19 pandemic in Canada Journal Article K. H. B. Leung, R. Alam, S. C. Brooks, T. C. Y. Chan In: Resuscitation, vol. 162, pp. 329-333, 2021. @article{ChanTCY.J079, Introduction: The COVID-19 pandemic has led to closures of non-essential businesses and buildings. The impact of such closures on automated external defibrillator (AED) accessibility compared to changes in foot traffic levels is unknown. Methods: We identified all publicly available online AED registries in Canada last updated May 1, 2019 or later. We mapped AED locations to location types and classified each location type as completely inaccessible, partially inaccessible, or unaffected based on government-issued closure orders as of May 1, 2020. Using location and mobility data from Google’s COVID-19 Community Mobility Reports, we identified the change in foot traffic levels between February 15–May 1, 2020 (excluding April 10–12) compared to the baseline of January 3–February 1, 2020, and determined the discrepancy between foot traffic levels and AED accessibility. Results: We identified four provincial and two municipal AED registries containing a total of 5848 AEDs. Of those, we estimated that 69.9% were completely inaccessible, 18.8% were partially inaccessible, and 11.3% were unaffected. Parks, retail and recreation locations, and workplaces experienced the greatest reduction in AED accessibility. The greatest discrepancies between foot traffic levels and AED accessibility occurred in parks, retail and recreation locations, and transit stations. Conclusion: A majority of AEDs became inaccessible during the COVID-19 pandemic due to government-mandated closures. In a substantial number of locations across Canada, the reduction in AED accessibility was far greater than the reduction in foot traffic. |
17. | C. L. F. Sun, L. Karlsson, L. J. Morrison, S. C. Brooks, F. Folke, T. C. Y. Chan In: Journal of the American Heart Association, vol. 9 (Article No. e016701), 2020. @article{ChanTCY.J069, BACKGROUND: Mathematical optimization of automated external defibrillator (AED) placement may improve AED accessibil- ity and out-of-hospital cardiac arrest (OHCA) outcomes compared with American Heart Association (AHA) and European Resuscitation Council (ERC) placement guidelines. We conducted an in silico trial (simulated prospective cohort study) com- paring mathematically optimized placements with placements derived from current AHA and ERC guidelines, which recommend placement in locations where OHCAs are usually witnessed. METHODS AND RESULTS: We identified all public OHCAs of presumed cardiac cause from 2008 to 2016 in Copenhagen, Denmark. For the control, we computationally simulated placing 24/7-accessible AEDs at every unique, public, witnessed OHCA location at monthly intervals over the study period. The intervention consisted of an equal number of simulated AEDs placements, deployed monthly, at mathematically optimized locations, using a model that analyzed historical OHCAs before that month. For each approach, we calculated the number of OHCAs in the study period that occurred within a 100-m route distance based on Copenhagen’s road network of an available AED after it was placed (“OHCA coverage”). Estimated impact on bystander defibrillation and 30-day survival was calculated by multivariate logistic regression. The control scenario involved 393 AEDs at historical, public, witnessed OHCA locations, covering 15.8% of the 653 public OHCAs from 2008 to 2016. The optimized locations provided significantly higher coverage (24.2%; P<0.001). Estimated bystander defibrillation and 30-day survival rates increased from 15.6% to 18.2% (P<0.05) and from 32.6% to 34.0% (P<0.05), respectively. As a baseline, the 1573 real AEDs in Copenhagen covered 14.4% of the OHCAs. CONCLUSIONS: Mathematical optimization can significantly improve OHCA coverage and estimated clinical outcomes compared with a guidelines-based approach to AED placement. |
16. | Improving access to automated external defibrillators in rural and remote settings: A drone delivery feasibility study Journal Article S. Cheskes, S. L. McLeod, M. Nolan, P. Snobelen, C. Vaillancourt, S. C. Brooks, K. N. Dainty, T. C. Y. Chan, I. R. Drennan In: Journal of the American Heart Association, vol. 9 (Article No. e016687), 2020. @article{ChanTCY.J066, BACKGROUND: Time to treatment is critical for survival from sudden cardiac arrest. Every minute delay in defibrillation results in a 7% to 10% reduction in survival. This is particularly problematic in rural and remote regions, where emergency medical service response is prolonged and automated external defibrillators (AEDs) are often not available. Our primary objective was to ex- amine the feasibility of a novel AED drone delivery method for rural and remote sudden cardiac arrest. A secondary objective was to compare response times between AED drone delivery and ambulance to mock sudden cardiac arrest resuscitations. METHODS AND RESULTS: We conducted 6 simulations in 2 rural communities in southern Ontario, Canada. In the first 2 simu- lations, the drone and ambulance were dispatched from the same paramedic base. In simulations 3 and 4, the drone and ambulance were dispatched from separate paramedic bases; and in simulations 5 and 6, the drone was dispatched from an optimized location. During each simulation, a “mock” call was placed to 911 and a single AED drone and an ambulance were simultaneously dispatched to a predetermined destination. On scene, trained first responders retrieved the AED from the drone and initiated resuscitative efforts on a mannequin until paramedics arrived. No difficulties were encountered during drone activation by dispatch, ascent, landing, or bystander retrieval of the AED from the drone. During simulations 1 and 2, the distance to the scene was 6.6 km. For simulations 3 and 4, the ambulance response distance increased to 8.8 km while drone remained at 6.6 km; and in simulations 5 and 6, the ambulance response distance was 20 km compared with 9 km for the drone. During each flight, the AED drone arrived on scene before the ambulance, between 1.8 and 8.0 minutes faster. CONCLUSIONS: This study suggests AED drone delivery is feasible, with the potential for improvements in response time during simulated sudden cardiac arrest scenarios. Further research is required to determine the appropriate system configuration for AED drone delivery in an integrated emergency medical service system as well as optimal strategies to simplify bystander application of a drone-delivered AED. |
15. | Optimal in-hospital defibrillator placement Journal Article K. H. B. Leung, C. L. F. Sun, M. Yang, K. S. Allan, N. Wong, T. C. Y. Chan In: Resuscitation, vol. 151, pp. 91-98, 2020. @article{ChanTCY.J065, Aims: To determine if mathematical optimization of in-hospital defibrillator placements can reduce in-hospital cardiac arrest-to-defibrillator distance compared to existing defibrillators in a single hospital. Methods: We identified treated IHCAs and defibrillator placements in St. Michael's Hospital in Toronto, Canada from Jan. 2013 to Jun. 2017 and mapped them to a 3-D computer model of the hospital. An optimization model identified an equal number of optimal defibrillator locations that minimized the average distance between IHCAs and the closest defibrillator using a 10-fold cross-validation approach. The optimized and existing defibrillator locations were compared in terms of average distance to the out-of-sample IHCAs. We repeated the analysis excluding intensive care units (ICUs), operating theatres (OTs), and the emergency department (ED). We also re-solved the model using fewer defibrillators to determine when the average distance matched the performance of existing defibrillators. Results: We identified 433 treated IHCAs and 53 defibrillators. Of these, 167 IHCAs and 31 defibrillators were outside of ICUs, OTs, and the ED. Optimal defibrillator placements reduced the average IHCA-to-defibrillator distance from 16.1 m to 2.7 m (relative decrease of 83.0%; P = 0.002) compared to existing defibrillator placements. For non-ICU/OT/ED IHCAs, the average distance was reduced from 24.4 m to 11.9 m (relative decrease of 51.3%; P = 0.002). 8‒9 optimized defibrillator locations were sufficient to match the average IHCA-to-defibrillator distance of existing defibrillator placements. Conclusions: Optimization-guided placement of in-hospital defibrillators can reduce the distance from an IHCA to the closest defibrillator. Equivalently, optimization can match existing defibrillator performance using far fewer defibrillators. |
14. | Improving bystander defibrillation in out-of-hospital cardiac arrests at home Journal Article L. Karlsson, C. M. Hansen, C. Vourakis, C. L. F. Sun, S. Rajan, K. B. Sondergaard, L. Andelius, F. Lippert, G. H. Gislason, T. C. Y. Chan, C. Torp-Pedersen, F. Folke In: European Heart Journal: Acute Cardiovascular Care, vol. 9, no. S4, pp. S74-S81, 2020. @article{ChanTCY.J063, Aims: Most out-of-hospital cardiac arrests occur at home with dismal bystander defibrillation rates. We investigated automated external defibrillator coverage of home arrests, and the proportion potentially reachable with an automated external defibrillator before emergency medical service arrival according to different bystander activation strategies. Methods and results: Cardiac arrests in homes (private/nursing/senior homes) in Copenhagen, Denmark (2008–2016) and registered automated external defibrillators (2007–2016), were identified. Automated external defibrillator coverage (distance from arrest to automated external defibrillator) and accessibility at the time of arrest were examined according to route distance to nearest automated external defibrillator and emergency medical service response time. The proportion of arrests reachable with an automated external defibrillator by bystander was calculated using two-way (from patient to automated external defibrillator and back) and one-way (from automated external defibrillator to patient) potential activation strategies. Of 1879 home arrests, automated external defibrillator coverage ≤100 m was low (6.3%) and a two-way bystander could potentially only retrieve an accessible automated external defibrillator before emergency medical service in 31.1% (n=37) of cases. If a bystander only needed to travel one-way to bring an automated external defibrillator (≤100 m, ≤250 m and ≤500 m), 45.4% (n=54/119), 37.1% (n=196/529) and 29.8% (n=350/1174) could potentially be reached before the emergency medical service based on current automated external defibrillator accessibility. Conclusions: Few home arrests were reachable with an automated external defibrillator before emergency medical service if bystanders needed to travel from patient to automated external defibrillator and back. However, nearly one-third of arrests ≤500 m of an automated external defibrillator could be reached before emergency medical service arrival if the bystander only needed to travel one-way from the automated external defibrillator to the patient. |
13. | In silico trial of optimized versus real public defibrillator locations Journal Article C. L. F. Sun, L. Karlsson, C. Torp-Pedersen, L. J. Morrison, S. C. Brooks, F. Folke, T. C. Y. Chan In: Journal of the American College of Cardiology, vol. 74, pp. 1557-1567, 2019. @article{ChanTCY.J055, Background: Automated external defibrillators (AEDs) are often placed in areas of low risk and limited temporal availability. Mathematical optimization can improve AED accessibility but has not been compared with current practices. Objectives: This study sought to determine whether, compared with real AED locations, optimized AED locations improve coverage of out-of-hospital cardiac arrests (OHCAs). Methods: The authors conducted the first retrospective in silico trial of an AED placement intervention. This study identified all public OHCAs of presumed cardiac cause and real AED deployed (control group) from 2007 to 2016 in Copenhagen, Denmark. Optimization models trained on historical OHCAs (1994 to 2007) were used to optimize an equal number of AEDs to the control group in locations with availabilities based on building hours (intervention #1) or 24/7 access (intervention #2). The 2 interventions and control scenario were compared using the number of OHCAs that occurred within 100 m of an accessible AED ("OHCA coverage") during the 2007 to 2016 period. Change in bystander defibrillation and 30-day survival were estimated using multivariate logistic regression. Results: There were 673 public OHCAs and 1,573 registered AEDs from 2007 to 2016. OHCA coverage of real AED placements was 22.0%. OHCA coverage of interventions #1 and #2 was significantly higher at 33.4% and 43.1%, respectively; relative gains of 52.0% to 95.9% (p < 0.001). Bystander defibrillation increased from 14.6% (control group) to 22.5% to 26.9% (intervention #1 to intervention #2); relative increase of 52.9% to 83.5% (p < 0.001). The 30-day survival rates increased from 31.3% (control group) to 34.7% to 35.4%, which is a relative increase of 11.0% to 13.3% (p < 0.001). Conclusions: Optimized AED placements increased OHCA coverage by approximately 50% to 100% over real AED placements, leading to significant predicted increases in bystander defibrillation and 30-day survival. |
12. | Health care utilization prior to out-of-hospital cardiac arrest: A population-based study Journal Article M. Shuvy, M. Koh, F. Qiu, S. C. Brooks, T. C. Y. Chan, S. Cheskes, P. Dorian, G. Geri, S. Lin, D. C. Scales, D. T. Ko In: Resuscitation, vol. 141, pp. 158-165, 2019. @article{ChanTCY.J053, Introduction: Although out-of-hospital cardiac arrest (OHCA) is thought of as a sudden event, recent studies suggest that many patients have symptoms or have sought medical attention prior to their arrest. Our objective was to evaluate patterns of healthcare utilization before OHCA. Methods: We conducted a population-based cohort study in Ontario, Canada, which included all patients ≥20 years, who suffered out-of-hospital cardiac arrest and transferred to an emergency department (ED) from 2007 to 2018. Measurements included emergency room assessments, hospitalizations and physician visits prior to arrest. Results: The cohort comprised 38,906 patients, their mean age was 66.5 years, and 32.7% were women. Rates of ED assessments and hospital admissions were relatively constant until 90 days prior to arrest where they markedly increased to the time before arrest. Within 90 days, rates of ED assessment, hospitalization, and primary care physician visit were 29.5%, 16.4%, and 70.1%, respectively. Cardiovascular conditions were diagnosed in 14.4% of ED visits, and 33.7% of hospitalizations in this time period. The largest age-difference was the mental and behavioural disorders within 90 days of OHCA in the ED, where rates were 12.2% among patients <65 years vs. 1.9% for patients ≥65 years. Conclusions: In contrast to the conventional wisdom that OHCA occurs without prior contacts to the health care system, we found that more than 1 in 4 patients were assessed in the ED prior within 90 days of their arrest. Identification of warning signs of OHCA may allow future development of prevention strategies. |
11. | Spatiotemporal AED optimization is generalizable Journal Article C. L. F. Sun, L. Karlsson, C. Torp-Pedersen, L. J. Morrison, F. Folke, T. C. Y. Chan In: Resuscitation, vol. 131, pp. 101-107, 2018. @article{ChanTCY.J047, Aims: Mathematical optimization of automated external defibrillator (AED) placements has the potential to improve out-of-hospital cardiac arrest (OHCA) coverage and reverse the negative effects of limited AED accessibility. However, the generalizability of optimization approaches has not yet been investigated. Our goal is to examine the performance and generalizability of a spatiotemporal AED placement optimization methodology, initially developed for Toronto, Canada, to the new study setting of Copenhagen, Denmark. Methods: We identified all public OHCAs (1994–2016) and all registered AEDs (2016) in Copenhagen, Denmark. We calculated the coverage loss associated with limited temporal accessibility of registered AEDs, and used a spatiotemporal optimization model to quantify the potential coverage gain of optimized AED deployment. Coverage gain of spatiotemporal deployment over a spatial-only solution was quantified through 10-fold cross-validation. Statistical testing was performed using χ^2 and McNemar's tests. Results: We found 2149 public OHCAs and 1573 registered AED locations. Coverage loss was found to be 24.4% (1104 OHCAs covered under assumed 24/7 coverage, and 835 OHCAs under actual coverage). The coverage gain from using the spatiotemporal model over a spatial-only approach was 15.3%. Temporal and geographical trends in coverage gain were similar to Toronto. Conclusions: Without modification, a previously developed spatiotemporal AED optimization approach was applied to Copenhagen, resulting in similar OHCA coverage findings as Toronto, despite large geographic and cultural differences between the two cities. In addition to reinforcing the importance of temporal accessibility of AEDs, these similarities demonstrate the generalizability of optimization approaches to improve AED placement and accessibility. |
10. | Robust defibrillator deployment under cardiac arrest location uncertainty via row-and-column generation Journal Article T. C. Y. Chan, Z.-J. Shen, A. Siddiq In: Operations Research, vol. 66, pp. 358-379, 2018. @article{ChanTCY.J038, Sudden cardiac arrest is a significant public health concern. Successful treatment of cardiac arrest is extremely time sensitive, and use of an automated external defibrillator (AED) where possible significantly increases the probability of survival. Placement of AEDs in public locations can improve survival by enabling bystanders to treat victims of cardiac arrest prior to the arrival of emergency medical responders, thus shortening the time between collapse and treatment. However, since the exact locations of future cardiac arrests cannot be known a priori, AEDs must be placed strategically in public locations to ensure their accessibility in the event of an out-of-hospital cardiac arrest emergency. In this paper, we propose a data-driven optimization model for deploying AEDs in public spaces while accounting for uncertainty in future cardiac arrest locations. Our approach involves discretizing a continuous service area into a large set of scenarios, where the probability of cardiac arrest at each location is itself uncertain. We model uncertainty in the spatial risk of cardiac arrest using a polyhedral uncertainty set that we calibrate using historical cardiac arrest data. We propose a solution technique based on row-and-column generation that exploits the structure of the uncertainty set, allowing the algorithm to scale gracefully with the total number of scenarios. Using real cardiac arrest data from the City of Toronto, we conduct an extensive numerical study on AED deployment public locations. We find that hedging against cardiac arrest location uncertainty can produce AED deployments that outperform an intuitive sample average approximation by 9%–15% and cuts the performance gap with respect to an ex post model by half. Our findings suggest that accounting for cardiac arrest location uncertainty can lead to improved accessibility of AEDs during cardiac arrest emergencies and the potential for improved survival outcomes. |
9. | Increased cardiac arrest survival and bystander intervention in enclosed pedestrian walkway systems Journal Article M. Lee, D. Demirtas, J. E. Buick, M. J. Feldman, S. Cheskes, L. J. Morrison, T. C. Y. Chan In: Resuscitation, vol. 118, pp. 1-7, 2017. @article{ChanTCY.J037, Background: Cities worldwide have underground or above-ground enclosed walkway systems for pedestrian travel, representing unique environments for studying out-of-hospital cardiac arrests (OHCAs). The characteristics and outcomes of OHCAs that occur in such systems are unknown. Objective: To determine whether OHCAs occurring in enclosed pedestrian walkway systems have differing demographics, prehospital intervention, and survival outcomes compared to the encompassing city, by examining the PATH walkway system in Toronto. Methods: We identified all atraumatic, public-location OHCAs in Toronto from April 2006 to March 2016. Exclusion criteria were obvious death, existing DNR, and EMS-witnessed OHCAs. OHCAs were classified into mutually exclusive location groups: Toronto, Downtown, and PATH-accessible. PATH-accessible OHCAs were those that occurred within the PATH system between the first basement and third floor. We analyzed demographic, prehospital intervention, and survival data using t-tests and chi-squared tests. Results: We identified 2172 OHCAs: 1752 Toronto, 371 Downtown, and 49 PATH-accessible. Compared to Toronto, a significantly higher proportion of PATH-accessible OHCAs was bystander-witnessed (62.6% vs 83.7%, p = 0.003), had bystander CPR (56.6% vs 73.5%, p = 0.019), bystander AED use (11.0% vs 42.6%, p<0.001), shockable initial rhythm (45.5% vs 72.9%, p<0.001), and overall survival (18.5% vs 33.3%, p = 0.009). Similar significant differences were observed when compared to Downtown. Conclusions: This study suggests that OHCAs in enclosed pedestrian walkway systems are uniquely different from other public settings. Bystander resuscitation efforts are significantly more frequent and survival rates are significantly higher. Urban planners in similar infrastructure systems worldwide should consider these findings when determining AED placement and public engagement strategies. |
8. | Optimizing a drone network to deliver automated external defibrillators Journal Article J. J. Boutilier, S. C. Brooks, A. Janmohamed, A. Byers, J. E. Buick, C. Zhan, A. P. Schoellig, S. Cheskes, L. J. Morrison, T. C. Y. Chan In: Circulation, vol. 135, pp. 2454-2465, 2017. @article{ChanTCY.J034, Background: Public access defibrillation programs can improve survival after out-of-hospital cardiac arrest, but automated external defibrillators (AEDs) are rarely available for bystander use at the scene. Drones are an emerging technology that can deliver an AED to the scene of an out-of-hospital cardiac arrest for bystander use. We hypothesize that a drone network designed with the aid of a mathematical model combining both optimization and queuing can reduce the time to AED arrival. Methods: We applied our model to 53 702 out-of-hospital cardiac arrests that occurred in the 8 regions of the Toronto Regional RescuNET between January 1, 2006, and December 31, 2014. Our primary analysis quantified the drone network size required to deliver an AED 1, 2, or 3 minutes faster than historical median 911 response times for each region independently. A secondary analysis quantified the reduction in drone resources required if RescuNET was treated as a large coordinated region. Results: The region-specific analysis determined that 81 bases and 100 drones would be required to deliver an AED ahead of median 911 response times by 3 minutes. In the most urban region, the 90th percentile of the AED arrival time was reduced by 6 minutes and 43 seconds relative to historical 911 response times in the region. In the most rural region, the 90th percentile was reduced by 10 minutes and 34 seconds. A single coordinated drone network across all regions required 39.5% fewer bases and 30.0% fewer drones to achieve similar AED delivery times. Conclusions: An optimized drone network designed with the aid of a novel mathematical model can substantially reduce the AED delivery time to an out-of-hospital cardiac arrest event. |
7. | Ranking businesses and municipal locations by spatiotemporal cardiac arrest risk to guide public defibrillator placement Journal Article C. L. F. Sun, S. C. Brooks, L. J. Morrison, T. C. Y. Chan In: Circulation, vol. 135, pp. 1104-1119, 2017. @article{ChanTCY.J033, Background: Efforts to guide automated external defibrillator placement for out-of-hospital cardiac arrest (OHCA) treatment have focused on identifying broadly defined location categories without considering hours of operation. Broad location categories may be composed of many businesses with varying accessibility. Identifying specific locations for automated external defibrillator deployment incorporating operating hours and time of OHCA occurrence may improve automated external defibrillator accessibility. We aim to identify specific businesses and municipal locations that maximize OHCA coverage on the basis of spatiotemporal assessment of OHCA risk in the immediate vicinity of franchise locations. Methods: This study was a retrospective population-based cohort study using data from the Toronto Regional RescuNET Epistry cardiac arrest database. We identified all nontraumatic public OHCAs occurring in Toronto, ON, Canada, from January 2007 through December 2015. We identified 41 unique businesses and municipal location types with ≥20 locations in Toronto from the YellowPages, Canadian Franchise Association, and the City of Toronto Open Data Portal. We obtained their geographic coordinates and hours of operation from Web sites, by phone, or in person. We determined the number of OHCAs that occurred within 100 m of each location when it was open (spatiotemporal coverage) for Toronto overall and downtown. The businesses and municipal locations were then ranked by spatiotemporal OHCA coverage. To evaluate temporal stability of the rankings, we calculated intraclass correlation of the annual coverage values. Results: There were 2654 nontraumatic public OHCAs. Tim Hortons ranked first in Toronto, covering 286 OHCAs. Starbucks ranked first in downtown, covering 110 OHCAs. Coffee shops and bank machines from the 5 largest Canadian banks occupied 8 of the top 10 spots in both Toronto and downtown. The rankings exhibited high temporal stability with intraclass correlation values of 0.88 (95% confidence interval, 0.83–0.93) in Toronto and 0.79 (95% confidence interval, 0.71–0.86) in downtown. Conclusions: We identified and ranked businesses and municipal locations by spatiotemporal OHCA risk in their immediate vicinity. This approach may help policy makers and funders to identify and prioritize potential partnerships for automated external defibrillator deployment in public-access defibrillator programs. |
6. | Rise and shock: Optimal defibrillator placement in a high-rise building Journal Article T. C. Y. Chan In: Prehospital Emergency Care, vol. 21, pp. 309-314, 2017. @article{ChanTCY.J032, Objective: Out-of-hospital cardiac arrests (OHCA) in high-rise buildings experience lower survival and longer delays until paramedic arrival. Use of publicly accessible automated external defibrillators (AED) can improve survival, but "vertical" placement has not been studied. We aim to determine whether elevator-based or lobby-based AED placement results in shorter vertical distance travelled ("response distance") to OHCAs in a high-rise building. Methods: We developed a model of a single-elevator, n-floor high-rise building. We calculated and compared the average distance from AED to floor of arrest for the two AED locations. We modeled OHCA occurrences using floor-specific Poisson processes, the risk of OHCA on the ground floor (λ_1) and the risk on any above-ground floor (λ). The elevator was modeled with an override function enabling direct travel to the target floor. The elevator location upon override was modeled as a discrete uniform random variable. Calculations used the laws of probability. Results: Elevator-based AED placement had shorter average response distance if the number of floors (n) in the building exceeded three quarters of the ratio of ground-floor OHCA risk to above-ground floor risk (λ_1/λ) plus one half (n ≥ 3λ_1/4λ + 0.5). Otherwise, a lobby-based AED had shorter average response distance. If OHCA risk on each floor was equal, an elevator-based AED had shorter average response distance. Conclusions: Elevator-based AEDs travel less vertical distance to OHCAs in tall buildings or those with uniform vertical risk, while lobby-based AEDs travel less vertical distance in buildings with substantial lobby, underground, and nearby street-level traffic and OHCA risk. |
5. | Overcoming spatial and temporal barriers to public access defibrillators via optimization Journal Article C. L. F. Sun, D. Demirtas, S. C. Brooks, L. J. Morrison, T. C. Y. Chan In: Journal of the American College of Cardiology, vol. 68, pp. 836-845, 2016. @article{ChanTCY.J030, BACKGROUND: Immediate access to an automated external defibrillator (AED) increases the chance of survival for out-of-hospital cardiac arrest (OHCA). Current deployment usually considers spatial AED access, assuming AEDs are available 24 h a day. OBJECTIVES: The goal of this study was to develop an optimization model for AED deployment, accounting for spatial and temporal accessibility, to evaluate if OHCA coverage would improve compared with deployment based on spatial accessibility alone. METHODS: This study was a retrospective population-based cohort trial using data from the Toronto Regional RescuNET Epistry cardiac arrest database. We identified all nontraumatic public location OHCAs in Toronto, Ontario, Canada (January 2006 through August 2014) and obtained a list of registered AEDs (March 2015) from Toronto Paramedic Services. Coverage loss due to limited temporal access was quantified by comparing the number of OHCAs that occurred within 100 meters of a registered AED (assumed coverage 24 h per day, 7 days per week) with the number that occurred both within 100 meters of a registered AED and when the AED was available (actual coverage). A spatiotemporal optimization model was then developed that determined AED locations to maximize OHCA actual coverage and overcome the reported coverage loss. The coverage gain between the spatiotemporal model and a spatial-only model was computed by using 10-fold cross-validation. RESULTS: A total of 2,440 nontraumatic public OHCAs and 737 registered AED locations were identified. A total of 451 OHCAs were covered by registered AEDs under assumed coverage 24 h per day, 7 days per week, and 354 OHCAs under actual coverage, representing a coverage loss of 21.5% (p < 0.001). Using the spatiotemporal model to optimize AED deployment, a 25.3% relative increase in actual coverage was achieved compared with the spatial-only approach (p < 0.001). CONCLUSIONS: One in 5 OHCAs occurred near an inaccessible AED at the time of the OHCA. Potential AED use was significantly improved with a spatiotemporal optimization model guiding deployment. |
4. | Optimizing the deployment of public access defibrillators Journal Article T. C. Y. Chan, D. Demirtas, R. H. Kwon In: Management Science, vol. 62, pp. 3617-3635, 2016. @article{ChanTCY.J028, Out-of-hospital cardiac arrest is a significant public health issue, and treatment, namely, cardiopulmonary resuscitation and defibrillation, is very time sensitive. Public access defibrillation programs, which deploy automated external defibrillators (AEDs) for bystander use in an emergency, reduce the time to defibrillation and improve survival rates. In this paper, we develop models to guide the deployment of public AEDs. Our models generalize existing location models and incorporate differences in bystander behavior. We formulate three mixed integer nonlinear models and derive equivalent integer linear reformulations or easily computable bounds. We use kernel density estimation to derive a spatial probability distribution of cardiac arrests that is used for optimization and model evaluation. Using data from Toronto, Canada, we show that optimizing AED deployment outperforms the existing approach by 40% in coverage, and substantial gains can be achieved through relocating existing AEDs. Our results suggest that improvements in survival and cost-effectiveness are possible with optimization. |
3. | Modeling the impact of public access defibrillator range on public location cardiac arrest coverage Journal Article A. A. Siddiq, S. C. Brooks, T. C. Y. Chan In: Resuscitation, vol. 84, pp. 904-909, 2013. @article{ChanTCY.J011, Background: Public access defibrillation with automated external defibrillators (AEDs) can improve survival from out-of-hospital cardiac arrests (OHCA) occurring in public. Increasing the effective range of AEDs may improve coverage for public location OHCAs. Objective: To quantify the relationship between AED effective range and public location cardiac arrest coverage. Methods: This was a retrospective cohort study using the Resuscitation Outcomes Consortium Epistry database. We included all public-location, atraumatic, EMS-attended OHCAs in Toronto, Canada between December 16, 2005 and July 15, 2010. We ran a mathematical model for AED placement that maximizes coverage of historical public OHCAs given pre-specified values of AED effective range and the number of locations to place AEDs. Locations of all non-residential buildings were obtained from the City of Toronto and used as candidate sites for AED placement. Coverage was evaluated for range values from 10 to 300 m and number of AED locations from 10 to 200, both in increments of 10, for a total of 600 unique scenarios. Coverage from placing AEDs in all public buildings was also measured. Results: There were 1310 public location OHCAs during the study period, with 25,851 non-residential buildings identified as candidate sites for AED placement. Cardiac arrest coverage increased with AED effective range, with improvements in coverage diminishing at higher ranges. For example, for a deployment of 200 AED locations, increasing effective range from 100 m to 200 m covered an additional 15% of cardiac arrests, whereas increasing range further from 200 m to 300 m covered an additional 10%. Placing an AED in each of the 25,851 public buildings resulted in coverage of 50% and 95% under assumed effective ranges of 50 m and 300 m, respectively. Conclusion: Increasing AED effective range can improve cardiac arrest coverage. Mathematical models can help evaluate the potential impact of initiatives which increase AED range. |
2. | Identifying locations for public access defibrillators using mathematical optimization Journal Article T. C. Y. Chan, H. Li, G. Lebovic, S. K. Tang, J. Y. T. Chan, H. C. K. Cheng, L. J. Morrison, S. C. Brooks In: Circulation, vol. 127, pp. 1801-1809, 2013. @article{ChanTCY.J010, Background—Geospatial methods using mathematical optimization to identify clusters of cardiac arrests and prioritize public locations for defibrillator deployment have not been studied. Our objective was to develop such a method and test its performance against a population-guided approach. Methods and Results—All public location cardiac arrests in Toronto, Ontario, Canada, from December 16, 2005, to July 15, 2010, and all automated external defibrillator (AED) locations registered with Toronto Emergency Medical Services as of September 2009 were plotted geographically. Current AED coverage was quantified by determining the number of cardiac arrests occurring within 100 m of a registered AED. Clusters of cardiac arrests without a registered AED within 100 m were identified. With the use of mathematical optimization techniques, cardiac arrest coverage improvements were computed and shown to be superior to results from a population-guided deployment method. There were 1310 eligible public location cardiac arrests and 1669 registered AEDs. Of the eligible cardiac arrests, 304 were within 100 m of at least 1 registered AED (23% coverage). The average distance from a cardiac arrest to the closest AED was 281 m. With AEDs deployed in the top 30 locations, an additional 112 historical cardiac arrests would be covered (32% total coverage), and the average distance to the closest AED would be 262 m. Conclusions—Geographic clusters of cardiac arrests can be easily identified and prioritized with the use of mathematical modeling. Optimized AED deployment can increase cardiac arrest coverage and decrease the distance to the closest AED. Mathematical modeling can augment public AED deployment programs. |
1. | S. C. Brooks, J. H. Hsu, S. K. Tang, R. Jeyakumar, T. C. Y. Chan In: Annals of Emergency Medicine, vol. 61, pp. 530-538, 2013. @article{ChanTCY.J009, Study objective: Automated external defibrillator use by lay bystanders during out-of-hospital cardiac arrest rarely occurs but can improve survival. We seek to estimate risk for out-of-hospital cardiac arrest by location type and evaluate current automated external defibrillator deployment in a Canadian urban setting to guide future automated external defibrillator deployment. Methods: This was a retrospective analysis of a population-based out-of-hospital cardiac arrest database. We included consecutive public location, nontraumatic, out-of-hospital cardiac arrests occurring in Toronto from January 1, 2006, to June 30, 2010, captured in the Resuscitation Outcomes Consortium Epistry database. Two investigators independently categorized each out-of-hospital cardiac arrest and automated external defibrillator location into one of 38 categories. Total site counts in each location category were used to estimate average annual per-site cardiac arrest incidence and determine the relative automated external defibrillator coverage for each location type. Results: There were 608 eligible out-of-hospital cardiac arrest cases. The top 5 location categories by average annual out-of-hospital cardiac arrests per site were race track/casino (0.67; 95% confidence interval [CI] 0 to 1.63), jail (0.62; 95% CI 0.3 to 1.06), hotel/motel (0.15; 95% CI 0.12 to 0.18), hostel/shelter (0.14; 95% CI 0.067 to 0.19), and convention center (0.11; 95% CI 0 to 0.43). Although schools were relatively lower risk for cardiac arrest, they represented 72.5% of automated external defibrillator–covered locations in the study region. Some higher-risk location types such as hotel/motel, hostel/shelter, and rail station were severely underrepresented with respect to automated external defibrillator coverage. Conclusion: We have identified types of locations with higher per-site risk for cardiac arrest relative to others. We have also identified potential mismatches between cardiac arrest risk by location type and registered automated external defibrillator distribution in a Canadian urban setting. |
Global Health
I am interested in bringing operations research methods to bear on important problems in global health. My main research project in this domain focuses on developing optimization models to guide ambulance deployment and routing in developing country settings, which are subject to major uncertainties in demand and travel time. Click here for a two-minute introduction to the project.
RELEVANT PUBLICATIONS
6. | Predicting protein and fat content in human donor milk using machine learning Journal Article R. K. Wong, M. A. Pitino, R. Mahmood, I. Y. Zhu, D. Stone, D. L. O'Connor, S. Unger, T. C. Y. Chan In: Journal of Nutrition, vol. 151, pp. 2075-2083, 2021. @article{ChanTCY.J084, Background: Donor milk is the standard of care for hospitalized very low birth weight (VLBW) infants when mother’s milk is unavailable; however, growth of donor milk–fed infants is frequently suboptimal. Variability in nutrient composition of donated milk complicates the production of a uniform pooled product and, subsequently, the provision of adequate nutrition to promote optimal growth and development of VLBW infants. We reasoned a machine learning approach to construct batches using characteristics of the milk donation might be an effective strategy in reducing the variability in donor milk product composition. Objective: The objective of this study was to identify whether machine learning models can accurately predict donor milk macronutrient content. We focused on predicting fat and protein, given their well-established importance in VLBW infant growth outcomes. Methods: Samples of donor milk, consisting of 272 individual donations and 61 pool samples, were collected from the Rogers Hixon Ontario Human Milk Bank and analyzed for macronutrient content. Four different machine learning models were constructed using independent variable groups associated with donations, donors, and donor-pumping practices. A baseline model was established using lactation stage and infant gestational status. Predictions were made for individual donations and resultant pools. Results: Machine learning models predicted protein of individual donations and pools with a mean absolute error (MAE) of 0.16 g/dL and 0.10 g/dL, respectively. Individual donation and pooled fat predictions had an MAE of 0.91 g/dL and 0.42 g/dL, respectively. At both the individual donation and pool levels, protein predictions were significantly more accurate than baseline, whereas fat predictions were competitive with baseline. Conclusions: Machine learning models can provide accurate predictions of macronutrient content in donor milk. The macronutrient content of pooled milk had a lower prediction error, reinforcing the value of pooling practices. Future research should examine how macronutrient content predictions can be used to facilitate milk bank pooling strategies. |
5. | Risk stratification for early detection of diabetes and hypertension in resource-limited settings: Machine learning analysis Journal Article J. J. Boutilier, T. C. Y. Chan, M. Ranjan, S. Deo In: Journal of Medical Internet Research, vol. 23, no. Article No. e20123, 2021. @article{ChanTCY.J077, Background: The impending scale up of noncommunicable disease screening programs in low- and middle-income countries coupled with limited health resources require that such programs be as accurate as possible at identifying patients at high risk. Objective: The aim of this study was to develop machine learning–based risk stratification algorithms for diabetes and hypertension that are tailored for the at-risk population served by community-based screening programs in low-resource settings. Methods: We trained and tested our models by using data from 2278 patients collected by community health workers through door-to-door and camp-based screenings in the urban slums of Hyderabad, India between July 14, 2015 and April 21, 2018. We determined the best models for predicting short-term (2-month) risk of diabetes and hypertension (a model for diabetes and a model for hypertension) and compared these models to previously developed risk scores from the United States and the United Kingdom by using prediction accuracy as characterized by the area under the receiver operating characteristic curve (AUC) and the number of false negatives. Results: We found that models based on random forest had the highest prediction accuracy for both diseases and were able to outperform the US and UK risk scores in terms of AUC by 35.5% for diabetes (improvement of 0.239 from 0.671 to 0.910) and 13.5% for hypertension (improvement of 0.094 from 0.698 to 0.792). For a fixed screening specificity of 0.9, the random forest model was able to reduce the expected number of false negatives by 620 patients per 1000 screenings for diabetes and 220 patients per 1000 screenings for hypertension. This improvement reduces the cost of incorrect risk stratification by US $1.99 (or 35%) per screening for diabetes and US $1.60 (or 21%) per screening for hypertension. Conclusions: In the next decade, health systems in many countries are planning to spend significant resources on noncommunicable disease screening programs and our study demonstrates that machine learning models can be leveraged by these programs to effectively utilize limited resources by improving risk stratification. |
4. | A drone delivery network for antiepileptic drugs: A framework and modeling case study in a lowest-income country Journal Article F. J. Mateen, K. H. B. Leung, A. C. Vogel, A. Fode Cissé, T. C. Y. Chan In: Transactions of the Royal Society of Tropical Medicine and Hygiene, vol. 114, pp. 308-314, 2020. @article{ChanTCY.J061, Background: In urbanized, low-income cities with high rates of congestion, delivery of antiepileptic drugs (AEDs) by unmanned aerial vehicles (drones) to people with epilepsy for both emergency and non-urgent distribution may prove beneficial. Methods: Conakry is the capital of the Republic of Guinea, a low-income sub-Saharan African country (2018 per capita gross national income US$830). We computed the number of drones and delivery times to distribute AEDs from a main urban hospital to 27 pre-identified gas stations, mosques and pharmacies and compared these to the delivery times of a personal vehicle. Results: We predict that a single drone could serve all pre-identified delivery locations in Conakry within a 20.4-h period. In an emergency case of status epilepticus, 8, 20 and 24 of the 27 pre-identified destinations can be reached from the hub within 5, 10 and 15 min, respectively. Compared with the use of a personal vehicle, the response time for a drone is reduced by an average of 78.8% across all times of the day. Conclusions: Drones can dramatically reduce the response time for both emergency and routine delivery of lifesaving medicines. We discuss the advantages and disadvantages of such a drone delivery model with relevance to epilepsy. However, the commissioning of a trial of drones for drug delivery in related diseases and geographies is justified. |
3. | Ambulance Emergency Response Optimization in developing countries Journal Article J. J. Boutilier, T. C. Y. Chan In: Operations Research, vol. 68, pp. 1315-1334, 2020. @article{ChanTCY.J060, The lack of emergency medical transportation is viewed as the main barrier to the access and availability of emergency medical care in low- and middle-income countries (LMICs). In this paper, we present a robust optimization approach to optimize both the location and routing of emergency response vehicles, accounting for uncertainty in travel times and spatial demand characteristic of LMICs. We traveled to Dhaka, Bangladesh, the sixth largest and third most densely populated city in the world, to conduct field research resulting in the collection of two unique data sets that inform our approach. These data are leveraged to estimate demand for emergency medical services in an LMIC setting and to predict the travel time between any two locations in the road network for different times of day and days of the week. We combine our prediction-optimization framework with a simulation model and real data to provide an in-depth investigation into three policy- related questions. First, we demonstrate that outpost locations optimized for weekday rush hour lead to good performance for all times of day and days of the week. Second, we find that the performance of the current system could be replicated using one third of the current outpost locations and one half of the current number of ambulances. Finally, we show that a fleet of small ambulances has the potential to significantly outperform tra- ditional ambulance vans. In particular, they are able to capture approximately three times more demand while reducing the median average response time by roughly 10%–18% over the entire week and 24%–35% during rush hour because of increased routing flexibility offered by more nimble vehicles on a larger road network. Our results provide practical insights for emergency response optimization that can be leveraged by hospital-based and private ambulance providers in Dhaka and other urban centers in developing countries. |
2. | Operations Research in Global Health: A scoping review with a focus on the themes of health equity and impact Journal Article B. D. Bradley, T. Jung, A. Tandon-Verma, B. Khoury, T. C. Y. Chan, Y.-L. Cheng In: Health Research Policy and Systems, vol. 15, no. Article No. 32, 2017. @article{ChanTCY.J035, Background: Operations research (OR) is a discipline that uses advanced analytical methods (e.g. simulation, optimisation, decision analysis) to better understand complex systems and aid in decision-making. Summary: Herein, we present a scoping review of the use of OR to analyse issues in global health, with an emphasis on health equity and research impact. A systematic search of five databases was designed to identify relevant published literature. A global overview of 1099 studies highlights the geographic distribution of OR and common OR methods used. From this collection of literature, a narrative description of the use of OR across four main application areas of global health – health systems and operations, clinical medicine, public health and health innovation – is also presented. The theme of health equity is then explored in detail through a subset of 44 studies. Health equity is a critical element of global health that cuts across all four application areas, and is an issue particularly amenable to analysis through OR. Finally, we present seven select cases of OR analyses that have been implemented or have influenced decision-making in global health policy or practice. Based on these cases, we identify three key drivers for success in bridging the gap between OR and global health policy, namely international collaboration with stakeholders, use of contextually appropriate data, and varied communication outlets for research findings. Such cases, however, represent a very small proportion of the literature found. Conclusion: Poor availability of representative and quality data, and a lack of collaboration between those who develop OR models and stakeholders in the contexts where OR analyses are intended to serve, were found to be common challenges for effective OR modelling in global health. |
1. | Estimating oxygen needs for childhood pneumonia in developing country health systems: A new model for expecting the unexpected Journal Article B. D. Bradley, S. R. C. Howie, T. C. Y. Chan, Y.-L. Cheng In: PLOS ONE, vol. 9, no. Article No. e89872, 2014. @article{ChanTCY.J019, Background: Planning for the reliable and cost-effective supply of a health service commodity such as medical oxygen requires an understanding of the dynamic need or 'demand' for the commodity over time. In developing country health systems, however, collecting longitudinal clinical data for forecasting purposes is very difficult. Furthermore, approaches to estimating demand for supplies based on annual averages can underestimate demand some of the time by missing temporal variability. Methods: A discrete event simulation model was developed to estimate variable demand for a health service commodity using the important example of medical oxygen for childhood pneumonia. The model is based on five key factors affecting oxygen demand: annual pneumonia admission rate, hypoxaemia prevalence, degree of seasonality, treatment duration, and oxygen flow rate. These parameters were varied over a wide range of values to generate simulation results for different settings. Total oxygen volume, peak patient load, and hours spent above average-based demand estimates were computed for both low and high seasons. Findings: Oxygen demand estimates based on annual average values of demand factors can often severely underestimate actual demand. For scenarios with high hypoxaemia prevalence and degree of seasonality, demand can exceed average levels up to 68% of the time. Even for typical scenarios, demand may exceed three times the average level for several hours per day. Peak patient load is sensitive to hypoxaemia prevalence, whereas time spent at such peak loads is strongly influenced by degree of seasonality. Conclusion: A theoretical study is presented whereby a simulation approach to estimating oxygen demand is used to better capture temporal variability compared to standard average-based approaches. This approach provides better grounds for health service planning, including decision-making around technologies for oxygen delivery. Beyond oxygen, this approach is widely applicable to other areas of resource and technology planning in developing country health systems. |
Sports Analytics
I am a passionate sports fan and enjoy analyzing interesting (decision) problems in sports. I have worked on topics in hockey, baseball, tennis, golf, football, and curling. Click here for a video of a talk I gave on sports analytics. Here is my TEDxUofT talk on baseball flexibility. A team of students and I developed an interactive NHL Expansion Draft optimization tool, which allows users to optimize protection and selection decisions in real time.
RELEVANT PUBLICATIONS
21. | Altered inter-segmental coordination in athletes with a history of concussion Journal Article K. Liu, T. C. Y. Chan, T. A. Burkhart, M. G. Hutchison In: Journal of Sports Sciences, vol. 42, no. 21, pp. 2061-2069, 2024. @article{ChanTCY.J125, Concussion-recovered athletes have a higher risk of injury following return to sport. This study investigated the effect of history of concussion on the pattern and variability of inter-segmental coordination in athletes during squat jumps and timed squat and hinge tasks. A human pose estimation algorithm was applied to videos of 111 athletes (72 with no history of concussion (NOHX), 9 within 1 year of concussion (CONC1), 30 more than one-year post-concussion (CONC2) performing a series of movement tasks. Continuous relative phase metrics, calculated from phase angles of two contiguous segments, were used to evaluate inter-segmental coordination. Linear models were used to evaluate the causal effect of concussion group on hip, knee, and ankle coordination and repetition duration for each task. CONC1 affected repetition duration and knee and hip coordination and variability, while CONC2 influenced knee coordination. The findings suggest that concussion may have long-term persisting effects on lower-limb inter-segmental coordination in athletes. |
20. | Learning risk preferences in Markov Decision Processes: An application to the fourth down decision in the National Football League Journal Article N. Sandholtz, L. Wu, M. Puterman, T. C. Y. Chan In: The Annals of Applied Statistics, vol. 18, no. 4, pp. 3205-3228, 2024. @article{ChanTCY.J0120, For decades, National Football League (NFL) coaches' observed fourth down decisions have been largely inconsistent with prescriptions based on statistical models. In this paper, we develop a framework to explain this discrepancy using a novel inverse optimization approach. We model the fourth down decision and the subsequent sequence of plays in a game as a Markov decision process (MDP), the dynamics of which we estimate from NFL play-by-play data from the 2014 through 2022 seasons. We assume that coaches' observed decisions are optimal but that the risk preferences governing their decisions are unknown. This yields a novel inverse decision problem for which the optimality criterion, or risk measure, of the MDP is the estimand. Using the quantile function to parameterize risk, we estimate which quantile-optimal policy yields the coaches' observed decisions as minimally suboptimal. In general, we find that coaches' fourth-down behavior is consistent with optimizing low quantiles of the next-state value distribution, which corresponds to conservative risk preferences. We also find that coaches exhibit higher risk tolerances when making decisions in the opponent's half of the field than in their own, and that league average fourth down risk tolerances have increased over the seasons in our data. |
19. | Evaluating space creation in the National Hockey League using puck and player tracking data Proceedings Article H. Inayatali, T. Chan In: Proceedings of the Linköping Hockey Analytics Conference LINHAC 2024 Research Track (Linköping Electronic Conference Proceedings 209), pp. 13-25, 2024. @inproceedings{ChanTCY.Oth010c, |
18. | No more throwing darts at the wall: Developing fair handicaps for darts using a Markov Decision Process Proceedings Article T. C. Y. Chan, C. Fernandes, R. Walker In: Proceedings of the 18th Annual MIT Sloan Sports Analytics Conference, 2024. @inproceedings{ChanTCY.Oth009c, |
17. | Equity, diversity, and inclusion in sports analytics Journal Article C. Fernandes, J. D. Vescovi, R. Norman, C. L. Bradish, N. Taback, T. C. Y. Chan In: Journal of Quantitative Analysis in Sports, vol. 20, no. 2, pp. 87-111, 2024. @article{ChanTCY.J0114, This paper presents a landmark study of equity, diversity and inclusion (EDI) in the field of sports analytics. We developed a survey that examined personal and job-related demographics, as well as individual perceptions and experiences about EDI in the workplace. We sent the survey to individuals in the five major North American professional leagues, representatives from the Olympic and Paralympic Committees in Canada and the U.S., the NCAA Division I programs, companies in sports tech/analytics, and university research groups. Our findings indicate the presence of a clear dominant group in sports analytics identifying as: young (72.0%), White (69.5%), heterosexual (89.7%) and male (82.0%). Within professional sports, males in management positions earned roughly 30,000(27equallyalarmingpaygapof17,000 (14%) was found between White and non-White management personnel. Of concern, females were nearly five times as likely to experience discrimination and twice as likely to have considered leaving their job due to isolation or feeling unwelcome. While they had similar levels of agreement regarding fair processes for rewards and compensation, females "strongly agreed" less often than males regarding equitable support, equitable workload, having a voice, and being taken seriously. Over one third (36.3%) of females indicated that they "strongly agreed" that they must work harder than others to be valued equally, compared to 9.8% of males. We conclude the paper with concrete recommendations that could be considered to create a more equitable, diverse and inclusive environment for individuals working within the sports analytics sector. |
16. | Miss it like Messi: Extracting value from off-target shots in soccer Journal Article E. Baron, N. Sandholtz, D. Pleuler, T. C. Y. Chan In: Journal of Quantitative Analysis in Sports, vol. 20, no. 1, pp. 37-50, 2024. @article{ChanTCY.J0113, Measuring soccer shooting skill is a challenging analytics problem due to the scarcity and highly contextual nature of scoring events. The introduction of more advanced data surrounding soccer shots has given rise to model-based metrics which better cope with these challenges. Specifically, metrics such as expected goals added, goals above expectation, and post-shot expected goals all use advanced data to offer an improvement over the classical conversion rate. However, all metrics developed to date assign a value of zero to off-target shots, which account for almost two-thirds of all shots, since these shots have no probability of scoring. We posit that there is non-negligible shooting skill signal contained in the trajectories of off- target shots and propose two shooting skill metrics that incorporate the signal contained in off-target shots. Specifically, we develop a player-specific generative model for shot trajectories based on a mixture of truncated bivariate Gaussian distributions. We use this generative model to compute metrics that allow us to attach non-zero value to off-target shots. We demonstrate that our proposed metrics are more stable than current state-of-the-art metrics and have increased predictive power. |
15. | Case ― Moneyball for Murderball: Using analytics to construct lineups in wheelchair rugby Journal Article T. C. Y. Chan, C. Fernandes, A. Loa, N. Sandholtz In: INFORMS Transactions on Education, vol. 24, no. 2, pp. 182-183, 2024. @article{ChanTCY.J112, |
14. | Case Article ― Moneyball for Murderball: Using analytics to construct lineups in wheelchair rugby Journal Article T. C. Y. Chan, C. Fernandes, A. Loa, N. Sandholtz In: INFORMS Transactions on Education, vol. 24, no. 2, pp. 175-181, 2024. @article{ChanTCY.J111, Motivated by the problem of lineup optimization in wheelchair rugby (WCR), this case study covers descriptive, predictive, and prescriptive analytics. The case is presented from the perspective of a new assistant coach of Canada’s national WCR team, who has been tasked by the head coach to use various analytics techniques to improve their lineups. Whereas the data and actors are fictitious, they are based on real data and discussions with the national team coach and sport scientists. To solve the case, students must conduct data analysis, regression modeling, and optimization modeling. These three steps are tightly linked, as the data analysis is needed to prepare the data for regression, and the regression outputs are used as parameters in the optimization. As such, students build proficiency in developing an end-to-end solution approach for a complex real-world problem. The primary learning objectives for the students are to understand the differences between descriptive, predictive, and prescriptive analytics, to build proficiency in implementing the models using appropriate software, and to identify how these techniques can be applied to solve problems in other sports or other application areas. |
13. | How to get away with murderball - An end-to-end analytics case study to construct lineups in wheelchair rugby Masters Thesis A. Loa, C. Fernandes, N. Sandholtz, T. C. Y. Chan OR/MS Today 50(2): 30-34, 2023. @mastersthesis{ChanTCY.M02, |
12. | A Markov process approach to untangling intention versus execution in tennis Journal Article T. C. Y. Chan, D. S. Fearing, C. Fernandes, S. Kovalchik In: Journal of Quantitative Analysis of Sports , vol. 18, no. 2, pp. 127-145, 2022. @article{ChanTCY.J101, Value functions are used in sports to determine the optimal action players should employ. However, most literature implicitly assumes that players can perform the prescribed action with known and fixed probability of success. The effect of varying this probability or, equivalently, “execution error” in implementing an action (e.g., hitting a tennis ball to a specific location on the court) on the design of optimal strategies, has received limited attention. In this paper, we develop a novel modeling framework based on Markov reward processes and Markov decision processes to investigate how execution error impacts a player’s value function and strategy in tennis. We power our models with hundreds of millions of simulated tennis shots with 3D ball and 2D player tracking data. We find that optimal shot selection strategies in tennis become more conservative as execution error grows, and that having perfect execution with the empirical shot selection strategy is roughly equivalent to choosing one or two optimal shots with average execution error. We find that execution error on backhand shots is more costly than on forehand shots, and that optimal shot selection on a serve return is more valuable than on any other shot, over all values of execution error. |
11. | Optimizer for the 2021 NHL expansion draft Masters Thesis M. Shin, Y. Shalaby, A. Loa, B. Potter, T. C. Y. Chan, R. Mahmood OR/MS Today 48(5): 52-54, 2021. @mastersthesis{ChanTCY.M01, |
10. | Points gained in football: Using Markov process-based value functions to assess team performance Journal Article T. C. Y. Chan, C. Fernandes, M. L. Puterman In: Operations Research, vol. 69, no. 3, pp. 877-894, 2021. @article{ChanTCY.J074, To develop a novel approach for performance assessment, this paper considers the problem of computing value functions in professional American football. We provide a theoretical justification for using a dynamic programming approach to estimating value functions in sports by formulating the problem as a Markov chain for two asymmetric teams. We show that the Bellman equation has a unique solution equal to the bias of the underlying infinite horizon Markov reward process. This result provides, for the first time in the sports analytics literature, a precise interpretation of the value function as the expected number of points gained or lost over and above the steady state points gained or lost. We derive a martingale representation for the value function that provides justification, in addition to the analysis of our empirical transition probabilities, for using an infinite horizon approximation of a finite horizon game. Using more than 160,000 plays from the 2013–2016 National Football League seasons, we derive an empirical value function that forms our points gained performance assessment metric, which quantifies the value created or destroyed on any play relative to expected performance. We show how this metric provides new insight into factors that distinguish performance. For example, passing plays generate the most points gained, whereas running plays tend to generate negative value. Short passes account for the majority of the top teams’ success and the worst teams’ poor performance. Other insights include how teams differ by down, quarter, and field position. The paper concludes with a case study of the 2019 Super Bowl and suggests how the key concepts might apply outside of sports. |
9. | Predicting plays in the National Football League Journal Article C. Fernandes, R. Yakubov, Y. Li, A. Prasad, T. C. Y. Chan In: Journal of Sports Analytics, vol. 6, pp. 35-43, 2020. @article{ChanTCY.J058, This paper aims to develop an interpretable machine learning model to predict plays (pass versus rush) in the National Football League that will be useful for players and coaches in real time. Using data from the 2013–2014 to 2016– 2017 NFL regular seasons, which included 1034 games and 130,344 pass/rush plays, we first develop and compare several machine learning models to determine the maximum possible prediction accuracy. The best performing model, a neural network, achieves a prediction accuracy of 75.3%, which is competitive with the state-of-the-art methods applied to other datasets. Then, we search over a family of simple decision tree models to identify one that captures 86% of the prediction accuracy of the neural network yet can be easily memorized and implemented in an actual game. We extend the analysis to building decision tree models tailored for each of the 32 NFL teams, obtaining accuracies ranging from 64.7% to 82.5%. Overall, our decision tree models can be a useful tool for coaches and players to improve their chances of stopping an offensive play. |
8. | A mathematical optimization framework for expansion draft decision making and analysis Journal Article K. E. C. Booth, T. C. Y. Chan, Y. Shalaby In: Journal of Quantitative Analysis in Sports, vol. 15, pp. 27-40, 2019. @article{ChanTCY.J052, In this paper, we present and analyze a mathematical programming approach to expansion draft optimization in the context of the 2017 NHL expansion draft involving the Vegas Golden Knights, noting that this approach can be generalized to future NHL expansions and to those in other sports leagues. In particular, we present a novel mathematical optimization approach, consisting of two models, to optimize expansion draft protection and selection decisions made by the various teams. We use this approach to investigate a number of expansion draft scenarios, including the impact of 'collaboration' between existing teams, the trade-off between team performance and salary cap flexibility, as well as opportunities for Vegas to take advantage of side agreements in a 'leverage' experiment. Finally, we compare the output of our approach to what actually happened in the expansion draft, noting both similarities and discrepancies between our solutions and the actual outcomes. Overall, we believe our framework serves as a promising foundation for future expansion draft research and decision-making in hockey and in other sports. |
7. | Process flexibility in baseball: The value of positional flexibility Journal Article T. C. Y. Chan, D. Fearing In: Management Science, vol. 65, pp. 1642-1666, 2019. @article{ChanTCY.J052, This paper introduces the formal study of process flexibility to the novel domain of sports analytics. In baseball, positional flexibility is the analogous concept to process flexibility from manufacturing. We study the flexibility of players (plants) on a baseball team who produce innings-played at different positions (products). We develop models and metrics to evaluate expected and worst-case performance under injury risk (capacity uncertainty) with continuous player-position capabilities. Using Major League Baseball data, we quantify the impact of flexibility on team and individual performance and explore the player chains that arise when injuries occur. We discover that top teams can attribute at least one to two wins per season to flexibility alone, generally as a result of long subchains in the infield or outfield. The least robust teams to worst-case injury, those whose performance is driven by one or two star players, are over four times as fragile as the most robust teams. We evaluate several aspects of individual flexibility, such as how much value individual players bring to their team in terms of average and worst-case performance. Finally, we demonstrate the generalizability of our framework for player evaluation by quantifying the value of potential free agent additions and uncovering the true 'MVP' of a team. |
6. | A Bayesian regression approach to handicapping tennis players based on a rating system Journal Article T. C. Y. Chan, R. Singal In: Journal of Quantitative Analysis in Sports, vol. 14, pp. 131-141, 2018. @article{ChanTCY.J046, This paper builds on a recently developed Markov Decision Process-based (MDP) handicap system for tennis, which aims to make amateur matches more competitive. The system gives points to the weaker player based on skill difference, which is measured by the point-win probability. However, estimating point-win probabilities at the amateur level is challenging since point-level data is generally only available at the professional level. On the other hand, tennis rating systems are widely used and provide an estimate of the difference in ability between players, but a rigorous determination of handicap using rating systems is lacking. Therefore, our goal is to develop a mapping between the Universal Tennis Rating (UTR) system and the MDP-based handicaps, so that two amateur players can determine an appropriate handicap for their match based only on their UTRs. We first develop and validate an approach to extract server-independent point-win probabilities from match scores. Then, we show how to map server-independent point-win probabilities to server-specific point-win probabilities. Finally, we use the estimated probabilities to produce handicaps via the MDP model, which are regressed against UTR differences between pairs of players. We conclude with thoughts on how a handicap system could be implemented in practice. |
5. | Improving fairness in match play golf through enhanced handicap allocation Journal Article T. C. Y. Chan, D. Madras, M. L. Puterman In: Journal of Sports Analytics, vol. 4, pp. 251-262, 2018. @article{ChanTCY.J039, In amateur golf, lower handicap players "give strokes" to higher handicap players based on their handicap differential to make head-to-head matches fairer. In match play, the standard way to allocate handicap strokes uses the "course-defined hole ranking". Using a bootstrapped simulation of over 70,000 matches based on 392 rounds of golf, we first show that the standard stroke allocation method and course-defined hole ranking favor the better player in 53% of matches. Then, we investigate the impact of three potential changes to stroke allocation: modifying the hole ranking; giving both players their full handicaps instead of using handicap differential; awarding extra strokes to the weaker player. Our two primary findings are: 1) fair matches can be achieved by giving the weaker player 0.5 extra strokes, which corresponds to a tie-breaker on a single hole; 2) giving both players their full handicap makes the fairness results robust to different hole rankings. Together, these simple changes can improve fairness in match play golf and improve generalizability to other courses. |
4. | A Markov Decision Process-based handicap system for tennis Journal Article T. C. Y. Chan, R. Singal In: Journal of Quantitative Analysis in Sports, vol. 12, pp. 179-189, 2016. @article{ChanTCY.J031, Handicap systems are used in many sports to improve competitive balance and equalize the match-win probability between opponents of differing ability. Recognizing the absence of such a system in tennis, we develop a novel optimization-based handicap system for tennis using a Markov Decision Process (MDP) model. In our handicap system, the weaker player is given β "free points" or "credits" at the start of the match, which he can use before the start of any point during the match to win the point outright. The MDP model determines two key features of the handicap system: (1) Fairness: the minimum value of β required to equalize the match-win probability, and (2) Achievability: the optimal policy governing usage of the β credits to achieve the desired match-win probability. We test the sensitivity of the handicap values to the model's input parameters. Finally, we apply the model to real match data to estimate professional handicaps. |
3. | The value of flexibility in baseball roster construction Proceedings Article T. C. Y. Chan, D. S. Fearing In: Proceedings of the 7th Annual MIT Sloan Sports Analytics Conference, 2013. @inproceedings{ChanTCY.Oth005c, Drawing inspiration from the theory of production flexibility in manufacturing networks, we provide the first optimization-based analysis of the value of positional flexibility (the ability of a player to play multiple positions) for a major league baseball team in the presence of injury risk. First, we develop novel statistical models to estimate (1) the likelihood and duration of player injuries during the regular season, and (2) fielding abilities at secondary fielding positions. Next, we develop a robust optimization model to calculate the degradation in team performance due to injuries. Finally, we apply this model to measure the difference in performance between a team with players who have positional flexibility and a team that does not. We find that using 2012 rosters, flexibility was expected to create from 3% (White Sox) to 15% (Cubs) in value for each team, measured in runs above replacement. In analyzing the results, we find that platoon advantages (e.g., having left-handed batters face right-handed pitchers) form an important component of flexibility. As a secondary finding, based on our statistical analysis of injuries, we find that the likelihood of injury increases with age, but the duration of injury does not. |
2. | Split personalities of NHL players: Using clustering, projection and regression to measure individual point shares Proceedings Article T. C. Y. Chan, D. C. Novati In: Proceedings of the 6th Annual MIT Sloan Sports Analytics Conference, 2012. @inproceedings{ChanTCY.Oth003c, Recent literature in hockey analytics has considered the use of clustering to determine specific categories or types of NHL players. Regression analysis has then been used to measure the contribution of each of these player types to team performance. This paper uses a combination of clustering, projection and regression methods to individualize the classification of NHL players. Instead of assigning each player to only one type, the overall "personality" of the player is split into fractional components representing different player types. The result is a unique make-up for each player, which is used to quantify his individual contributions to his team's performance, a metric known as "point shares". Top ranked players in terms of point shares tend to be winners of major NHL awards, are leaders in scoring, and have the highest salaries. High point shares in a contract year may also factor into salary increases. Overall, a better understanding of individual NHL player characteristics may provide a foundation for deeper, data-driven player analysis. |
1. | Quantifying the contribution of NHL player types to team performance Journal Article T. C. Y. Chan, J. A. Cho, D. C. Novati In: Interfaces, vol. 42, pp. 131-145, 2012. @article{ChanTCY.J007, In this paper, we use k-means clustering to define distinct player types for each of the three positions on a National Hockey League (NHL) team and then use regression to determine a quantitative relationship between team performance and the player types identified in the clustering. Using NHL regular-season data from 2005–2010, we identify four forward types, four defensemen types, and three goalie types. Goalies tend to contribute the most to team performance, followed by forwards and then defensemen. We also show that once we account for salary cap and playing-time information, the value of different player types may become similar. Lastly, we illustrate how to use the regression results to analyze trades and their impact on team performance. |
Healthcare Operations
I am interested in a variety of healthcare operations problems including scheduling and process flexibility.
RELEVANT PUBLICATIONS
24. | Optimizing locations for public naloxone kits for opioid poisoning using geospatial analytics: A modelling study Journal Article Forthcoming K. H. B. Leung, B. E. Grunau, M. K. Lee, J. Buxton, J. Helmer, S. van Diepen, J. Christenson, T. C. Y. Chan In: CMAJ, Forthcoming. @article{ChanTCY.J133, |
23. | Dynamic control of service systems with returns: Application to design of post-discharge hospital readmission prevention programs Journal Article Forthcoming T. C. Y. Chan, S. Y. Huang, V. Sarhangian In: Operations Research, Forthcoming. @article{ChanTCY.J0130, We study a control problem for queueing systems where customers may return for additional episodes of service after their initial service completion. At each service completion epoch, the decision maker can choose to reduce the probability of return for the departing customer but at a cost that is convex increasing in the amount of reduction in the return probability. Other costs are incurred as customers wait in the queue and every time they return for service. Our primary motivation comes from post-discharge Quality Improvement (QI) interventions (e.g., follow up phone-calls, appointments) frequently used in a variety of healthcare settings to reduce unplanned hospital readmissions. Our objective is to understand how the cost of interventions should be balanced with the reductions in congestion and service costs. To this end, we consider a fluid approximation of the queueing system and characterize the structure of optimal long-run average and bias-optimal transient control policies for the fluid model. Our structural results motivate the design of intuitive surge protocols whereby different intensities of interventions (corresponding to different levels of reduction in the return probability) are provided based on the congestion in the system. Through extensive simulation experiments, we study the performance of the fluid policy for the stochastic system and identify parameter regimes where it leads to significant cost savings compared to a fixed long-run average optimal policy that ignores holding costs and a simple policy that uses the highest level of intervention whenever the queue is non-empty. In particular, we find that in a parameter regime relevant to our motivating application, dynamically adjusting the intensity of interventions could result in up to 25.4% reduction in long-run average cost and 33.7% in finite-horizon costs compared to the simple aggressive policy. |
22. | Exact sensitivity analysis of Markov reward processes via algebraic geometry Miscellaneous T. C. Y. Chan, M. Maaz 2024. @misc{ChanTCY.Pre021, We introduce a new approach for deterministic sensitivity analysis of Markov reward processes, commonly used in cost-effectiveness analyses, via reformulation into a polynomial system. Our approach leverages cylindrical algebraic decomposition (CAD), a technique arising from algebraic geometry that provides an exact description of all solutions to a polynomial system. While it is typically intractable to build a CAD for systems with more than a few variables, we show that a special class of polynomial systems, which includes the polynomials arising from Markov reward processes, can be analyzed much more tractably. We establish several theoretical results about such systems and develop a specialized algorithm to construct their CAD, which allows us to perform exact, multi-way sensitivity analysis for common health economic analyses. We develop an open-source software package that implements our algorithm. Finally, we apply it to two case studies, one with synthetic data and one that re-analyzes a previous cost-effectiveness analysis from the literature, demonstrating advantages of our approach over standard techniques. Our software and code are available at: https://github.com/mmaaz-git/markovag |
21. | M. Naghshbandi, S. Y. Huang, H. Shah, C. Yim, E. Lee, M. Caesar, A. Hope, T. C. Y. Chan, V. Sarhangian In: Canadian Liver Journal, vol. 7, no. 4, pp. 427-438, 2024. @article{ChanTCY.J0123, Background: We evaluated an outpatient, Nurse Practitioner (NP)-led transitional care program with respect to its efficacy in reducing unplanned readmission rates for liver disease patients. Methods: We conducted a retrospective cohort study using data from an academic health system in Toronto, Ontario. The study included 803 admissions associated with an ICD10 code of R18 (ascites), I85.0 or I98.3 (variceal bleeding), or K70 K77 range (diseases of liver including hepatic encephalopathy). Patients were selected to receive the transitional care (intervention group) or not (no-intervention group) by discretion of the hepatologists. We used the competing risk proportional hazard model to estimate the associations between receiving the intervention, and marginal probability of 30-, 60-, and 90-day readmission in presence of death as a competing risk. We conducted sensitivity analyses to examine the robustness of our estimates to various sources of bias, including adjusting for propensity of receiving the intervention estimated using a logistic regression model. Results: The intervention was associated with 49% reduction in risk of 30-day readmission (HR: 0.51, 95% CI, 0.30-0.85), 40% reduction in risk of 60-day readmission (HR:0.60, 95% CI, 0.40- 0.91), and 45% reduction in risk of 90-day readmission (HR:0.55, 95% CI, 0.37-0.83). The negative associations remained statistically significant under the sensitivity analyses, except for the propensity adjusted estimate for the 60-day outcome. Conclusions: A NP-led transitional-care program could be effective in reducing the risk of readmission for liver disease patients. Future studies are needed to standardize the referral process and prospectively measure the effectiveness and financial value of the program. |
20. | Centre-specific variation in atrial fibrillation ablation rates in a universal single-payer health care system Journal Article C. Seo, S. Kushwaha, P. Angaran, P. Gozdyra, K. S. Allan, H. Abdel-Qadir, P. Dorian, T. C. Y. Chan In: CJC Open, vol. 6, no. 11, pp. 1355-1362, 2024. @article{ChanTCY.J0122, Background Disparities in atrial fibrillation ablation rates have been previously studied, with focuses on patient characteristics and systems factors rather than geographic factors. The impact of electrophysiology centre practice patterns on ablation rates have not been well studied. Methods This was a population-based cohort study using linked administrative datasets covering physician billing codes, hospitalizations, prescriptions, and census data. The study population consisted of patients visiting an emergency department with a new diagnosis of atrial fibrillation between 2007-2016, in Ontario, Canada. Patient characteristics, including age, sex, medical history, comorbidities, socioeconomic factors, closest electrophysiology centre within 20 km, and distance to nearest centre were used as predictors in multivariable logistic regression models to assess the relationship between living around specific electrophysiology centres and ablation rates. Results The cohort included 134,820 patients of whom 9,267 had an ablation during the study period. Patients undergoing ablation were younger, had a lower CHADS2 score, lived closer to electrophysiology centres, and had fewer comorbidities than people who did not receive ablation. There was wide variation in ablation rates, with adjacent census divisions having up to 2.6 times higher ablation rate. Multivariate regression revealed significant differences in ablation rates for patients living around certain electrophysiology centres. The odds ratios for living closest to specific centres ranged from 0.78 (95% CI: 0.68-0.89) to 1.60 (95% CI:1.34-1.90). Conclusions Living near specific electrophysiology centres may significantly affect a patient’s likelihood of ablation, regardless of factors such as age, gender, socioeconomic status, prior medical history, and distance to electrophysiology centres. |
19. | Synergizing radiation oncology and operations research Book Chapter S. Kim, T. C. Y. Chan In: Fox, C. J.; Munbodh, R. (Ed.): Workflow Optimization in Radiation Oncology: From Theory to Clinical Implementation, no. Medical Physics Monograph 41, Chapter 7, Medical Physics Publishing, Madison, 2024, ISBN: 978-1-951134-31-0. @inbook{ChanTCY.Oth012, |
18. | Disparities in surgery rates during the COVID-19 pandemic: Retrospective study Journal Article A. Sankar, T. A. Stukel, N. N. Baxter, D. N. Wijeysundera, S. W. Hwang, A. S. Wilton, T. C. Y. Chan, V. Sarhangian, A. N. Simpson, C. de Mestral, D. Pincus, R. J. Campbell, D. R. Urbach, J. Irish, D. Gomez In: BJS Open, vol. 8, no. 4, pp. zrae088, 2024. @article{ChanTCY.J0121, |
17. | Dynamic transfer policies for parallel queues Miscellaneous T. C. Y. Chan, J. Park, V. Sarhangian 2024. @misc{ChanTCY.Pre020, We consider the problem of load balancing in parallel queues by transferring customers between them at discrete points in time. Holding costs accrue as customers wait in the queue, while transfer decisions incur both fixed (setup) and variable costs proportional to the number and direction of transfers. Our work is primarily motivated by inter-facility patient transfers between hospitals during a surge in demand for hospitalization (e.g., during a pandemic). By analyzing an associated fluid control problem, we show that under fairly general assumptions including time-varying arrivals and convex increasing holding costs, the optimal policy in each period partitions the state-space into a well-defined no-transfer region and its complement, such that transferring is optimal if and only if the system is sufficiently imbalanced. In the absence of fixed transfer costs, an optimal policy moves the state to the no-transfer region's boundary; in contrast, with fixed costs, the state is moved to the no-transfer region's relative interior. We further leverage the fluid control problem to provide insights on the trade-off between holding and transfer costs, emphasizing the importance of preventing excessive idleness when transfers are not feasible in continuous-time. Using simulation experiments, we investigate the performance and robustness of the fluid policy for the stochastic system. In particular, our case study calibrated using data during the pandemic in the Greater Toronto Area demonstrates that transferring patients between hospitals could result in up to 27.7% reduction in total cost with relatively few transfers. |
16. | Evolution of the surgical procedure gap during and after the COVID-19 pandemic in Ontario, Canada: Cross-sectional and modeling study Journal Article R. Stephenson, V. Sarhangian, J. Park, A. Sankar, N. N. Baxter, T. A. Stukel, A. N. Simpson, D. N. Wijeysundera, A. S. Wilton, C. de Mestral, S. W. Hwang, D. Pincus, R. J. Campbell, D. R. Urbach, J. Irish, D. Gomez, T. C. Y. Chan In: British Journal of Surgery, vol. 110, no. 12, pp. 1887-1889, 2023. @article{ChanTCY.J0109, During the COVID-19 pandemic, many countries faced significant reductions in surgical capacity, leading to unprecedented surgical backlogs, or procedure gaps. This study developed a framework to estimate procedure gaps and project their future evolution. The framework was applied to data from Ontario, Canada to provide policy insights for a fair and effective surgical recovery. This study updates previous work that estimated the impacts of COVID on surgery rates and future surgical recovery by providing a more recent estimate, and adding modeling of major ongoing COVID impacts. In reality, COVID and its downstream effects have continued to impact healthcare systems into 2023, and can be reasonably expected to continue into the future. Population-based weekly surgery count data was obtained for all scheduled adult surgical procedures in Ontario between January 1, 2017 and June 25, 2022, grouped by inpatient/outpatient and body system. Negative Binomial regression was used to estimate the expected size of the procedure gaps as of June 25, 2022, and Monte Carlo simulation was used to estimate their evolution over 10 years under future COVID and surgical capacity-increase scenarios (Figure S1). As of June 25, 2022, the total outpatient and inpatient procedure gaps were estimated to be 214 925 (95% CI 207 281-222 569) and 99 232 (95% CI 96 856-101 609), respectively (Table 1). Assuming no future impacts of COVID and 10% or 20% increases in surgical capacity, all procedure gaps were estimated to clear within 10 years. However, under scenarios in which COVID impacts persist, with a 0% or 10% increase in surgical capacity, no procedure gaps were expected to clear within 10 years. With a 20% increase, only three procedure gaps were expected to clear; several other gaps were expected to grow. Our results highlight the heterogeneous impact of the pandemic on different procedure groups. These differences are apparent in the growth of procedure gaps over the pandemic (Figure S4), and in their forecast evolution. For example, the two largest outpatient procedure gaps (Eye and Musculoskeletal), which have been the subject of pre-pandemic prioritization through added capacity and volume-based funding models, make up almost half of the total outpatient procedure gap. However, even if COVID impacts persist, their forecasted gaps are expected to drop significantly with a 10% increase in surgical capacity (Table 1). In contrast, the estimated inpatient Gynecology gap is currently much smaller, but even with a 20% increase in capacity, the gap is expected to more than double by 2032 (Table 1). On February 2, 2023, the Ontario government released a plan to significantly increase cataract surgeries and hip and knee replacements through the use of for-profit centres, but without a clear plan to increase overall surgical capacity in hospitals. Our results suggest that other procedure groups (e.g., Gynecology and Otolaryngology) require targeted increases in surgical capacity, especially if those groups are predominantly funded through global hospital budgets. To avoid unfair patient experiences like extensive wait times, targeted investments considering both the current procedure gaps and their future evolution are necessary for surgical recovery plans that strike a balance between efficiency and equity of clearing the procedure gaps. This study has two key takeaways. First, small increases in overall surgical capacity will have little impact on clearing the surgical procedure gap in the near term. Second, capacity increases should be targeted by considering not only current procedure gaps, but also their forecasted evolution. Indeed, procedure groups with the largest gaps currently may not be the ones most in need of increased capacity investments. The developed framework can be applied to other jurisdictions to provide insights for design of robust surgical recovery plans. |
15. | Constrained optimization for decision making in healthcare using Python: A tutorial Journal Article K. H. B. Leung, N. Yousefi, T. C. Y. Chan, A. M. Bayoumi In: Medical Decision Making, vol. 43, no. 7-8, pp. 760-773, 2023. @article{ChanTCY.J0108, Constrained optimization can be used to make decisions aimed at maximizing some quantity in the face of fixed limits, such as resource allocation problems in health where tradeoffs between alternatives are inherent, and has been applied in a variety of health-related applications. This tutorial guides the reader through the process of mathematially formulating a constrained optimization problem, providing intuitive explanations for each component within the problem. We discuss how constrained optimization problems can be implemented using software and provide instructions on how to set up a solution environment using Python and the Gurobi solver engine. We present 2 examples from the existing literature that illustrate different constrained optimization problems in health and provide the reader with Python code used to solve these problems as well as a discussion of results and sensitivity analyses. This tutorial can be used to help readers formulate constrained optimization problems in their own application domains. |
14. | Priorities for artificial intelligence in primary care: A Canadian deliberative dialogue with patients, providers, and health system leaders Journal Article T. L. Upshaw, A. Craig-Neil, J. Macklin, C. Steele Gray, T. C. Y. Chan, J. Gibson, A. D. Pinto In: Journal of the American Board of Family Medicine, vol. 36, no. 2, pp. 210-220, 2023. @article{ChanTCY.J104, BACKGROUND: Artificial intelligence (AI) implementation in primary care is limited. Those set to be most impacted by AI technology in this setting should guide the application. We organized a national deliberative dialogue with primary care stakeholders from across Canada to explore how they thought AI should be applied in primary care. METHODS: We conducted 12 virtual deliberative dialogues with 22 patients, 21 interprofessional primary care providers, and 5 health system leaders from eight Canadian provinces to identify shared priorities for applying AI in primary care. Dialogue data were thematically analyzed using interpretive description approaches. RESULTS: Participants thought that AI should be first applied in primary care to documentation, practice operations, and triage tasks, in hopes of improving efficiency while maintaining person-centred delivery, relationships, and access. They viewed complex AI-driven clinical decision support and proactive care tools as impactful but recognized potential risks to patient safety, given numerous external limitations. Appropriate training and implementation support were the most important external enablers of safe, effective, and patient-centred use of AI in primary care settings. INTERPRETATION: Our findings offer an agenda for the future application of AI in primary care that is grounded in the shared values of patients and providers. We propose that, from conception, AI developers should work with primary care stakeholders as co-design partners, developing tools that respond to their shared priorities. |
13. | Strategies for lung- and diaphragm-protective ventilation in acute hypoxemic respiratory failure: A physiological trial Journal Article J. Dianti, S. Fard, J. Wong, T. C. Y. Chan, L. Del Sorbo, E. Fan, M. B. Passos Amato, J. Granton, L. Burry, W. D. Reid, B. Zhang, D. Ratano, S. Keshavjee, A. S. Slutsky, L. J. Brochard, N. D. Ferguson, E. C. Goligher In: Critical Care, vol. 26, no. Article No. 259, 2022. @article{ChanTCY.J100, Background: Insufficient or excessive respiratory effort during acute hypoxemic respiratory failure (AHRF) increases the risk of lung and diaphragm injury. We sought to establish whether respiratory effort can be optimized to achieve lung- and diaphragm-protective (LDP) targets (esophageal pressure swing − 3 to − 8 cm H2O; dynamic transpulmonary driving pressure ≤ 15 cm H2O) during AHRF. Methods: In patients with early AHRF, spontaneous breathing was initiated as soon as passive ventilation was not deemed mandatory. Inspiratory pressure, sedation, positive end-expiratory pressure (PEEP), and sweep gas flow (in patients receiving veno-venous extracorporeal membrane oxygenation (VV-ECMO)) were systematically titrated to achieve LDP targets. Additionally, partial neuromuscular blockade (pNMBA) was administered in patients with refrac- tory excessive respiratory effort. Results: Of 30 patients enrolled, most had severe AHRF; 16 required VV-ECMO. Respiratory effort was absent in all at enrolment. After initiating spontaneous breathing, most exhibited high respiratory effort and only 6/30 met LDP targets. After titrating ventilation, sedation, and sweep gas flow, LDP targets were achieved in 20/30. LDP targets were more likely to be achieved in patients on VV-ECMO (median OR 10, 95% CrI 2, 81) and at the PEEP level associated with improved dynamic compliance (median OR 33, 95% CrI 5, 898). Administration of pNMBA to patients with refractory excessive effort was well-tolerated and effectively achieved LDP targets. Conclusion: Respiratory effort is frequently absent under deep sedation but becomes excessive when spontaneous breathing is permitted in patients with moderate or severe AHRF. Systematically titrating ventilation and sedation can optimize respiratory effort for lung and diaphragm protection in most patients. VV-ECMO can greatly facilitate the delivery of a LDP strategy. |
12. | Inverse optimization on hierarchical networks: An application to breast cancer clinical pathways Journal Article T. C. Y. Chan, K. Forster, S. Habbous, C. Holloway, L. Ieraci, Y. Shalaby, N. Yousefi In: Health Care Management Science, vol. 25, pp. 590-622, 2022. @article{ChanTCY.J099, Clinical pathways are standardized processes that outline the steps required for managing a specific disease. However, patient pathways often deviate from clinical pathways. Measuring the concordance of patient pathways to clinical pathways is important for health system monitoring and informing quality improvement initiatives. In this paper, we develop an inverse optimization-based approach to measuring pathway concordance in breast cancer, a complex disease. We capture this complexity in a hierarchical network that models the patient’s journey through the health system. A novel inverse shortest path model is formulated and solved on this hierarchical network to estimate arc costs, which are used to form a concordance metric to measure the distance between patient pathways and shortest paths (i.e., clinical pathways). Using real breast cancer patient data from Ontario, Canada, we demonstrate that our concordance metric has a statistically significant association with survival for all breast cancer patient subgroups. We also use it to quantify the extent of patient pathway discordances across all subgroups, finding that patients undertaking additional clinical activities constitute the primary driver of discordance in the population. |
11. | An anesthesia block room is financially net positive for a hospital performing arthroplasty Journal Article J. Toor, I. Saleh, A. Abbas, J. Abouali, P. Wong, T. C. Y. Chan, V. Sarhangian In: Journal of the American Academy of Orthopaedic Surgeons, vol. 30, no. 15, pp. e1058-e1065, 2022. @article{ChanTCY.J098, Introduction: Regional anesthesia is increasingly used in total joint arthroplasty (TJA). It has shown efficiency benefits as it allows parallel processing of patients in a dedicated block room (BR). However, granular quantification of these benefits to hospital operations is lacking. The goal of this study was to determine the financial effect of establishing a BR using comprehensive operational modeling. Methods: A discrete-event simulation model of daily operating room (OR) patient flow for TJA procedures at a mid-sized hospital was developed. Two scenarios were tested: (1) without and (2) with a BR. Scenarios were compared according to staffing requirements, hours/day, and labor costs. The number of ORs and cases varied from 2 to 6 ORs performing 3 to 5 cases. These results were used as the inputs of a discounted cash flow (CF) model. Discounted CF model outputs were CF, net present value, internal rate of return, and return on investment. Results: Mean time savings of incorporating a BR were 68 min/d (range: 30 to 80 min/d), reducing the OR closing time by 1 hour. Incremental labor costs/day from nurse overtime pay ranged from $2,025 to $10,125 with no BR and $1,595 to $9,045 with a BR, which resulted in an increase in profit/day from $360 to $1,605. The CF/annum was $54,363, the net present value was $213,082, the internal rate of return was 12%, and the return on investment was 43.61%. Discussion: This study demonstrates that under all scenarios, a BR is more profitable than no BR to a hospital performing TJA via a bundled care or private payer remuneration model. A BR was shown to be financially net positive even when considering the necessary financial investment to establish it. In addition, this study demonstrates the potential of combining discrete-event simulation with financial analyses to assess various operational models of care to improve hospital efficiency, such as dedicated trauma rooms and swing rooms. Level of evidence: Level III |
10. | Drone network design for cardiac arrest response Journal Article J. J. Boutilier, T. C. Y. Chan In: Manufacturing and Service Operations Management, vol. 24, no. 5, pp. 2407-2424, 2022. @article{ChanTCY.J096, Problem definition: Our objective is to design a defibrillator-enabled drone network that augments the existing emergency medical services (EMS) system to rapidly respond to out-of-hospital cardiac arrest (OHCA). Academic/practical relevance: OHCA claims more than 400,000 lives each year in North America and is one of the most time-sensitive medical emergencies. Drone-delivered automated external defibrillators (AEDs) have the potential to be a transformative innovation in the provision of emergency care for OHCA. Methodology: We develop an integrated location-queuing model that incorporates existing EMS response times and is based on the p-median architecture, where each base constitutes an explicit M/M/d/d queue (i.e., Erlang loss). We then develop a reformulation technique that exploits the existing EMS response times, allowing us to solve real-world instances to optimality using an off-the-shelf solver. We evaluate our solutions using a tactical simulation model that accounts for the effects of congestion and dispatching, and we use a machine-learning model to translate our response-time reductions into survival estimates. Results:Using real data from an area covering 26,000 square kilometers around Tor-onto, Canada, we find that a modest number of drones are required to significantly reduce response times in all regions. An objective function that minimizes average response time results in drone resources concentrated in cities, with little impact on the tail of the distribution. In contrast, optimizing for the tail of the response-time distribution produces larger and more geographically dispersed drone networks that improve response-time equity across the regions. We estimate that the response-time reductions achieved by the drone network are associated with between a 42% and 76% higher survival rate and up to 144 additional lives saved each year across the geographical region we consider. Managerial implications: Overall, this paper provides a realistic framework that can be leveraged by system designers and/or EMS personnel seeking to investigate design questions associated with a drone network. An objective function focused on improving the tail of the response-time distribution is well-suited for use in practice because the model provides equitable solutions that reduce the entire response-time distribution and corresponds to the real-world metrics, on which EMS systems are most commonly evaluated. |
9. | Outcomes in patients with and without disability admitted to hospital with COVID-19: A retrospective cohort study Journal Article H. K. Brown, S. Saha, T. C. Y. Chan, A. M. Cheung, M. Fralick, M. Ghassemi, M. Herridge, J. Kwan, S. Rawal, L. Rosella, T. Tang, A. Weinerman, Y. Lunsky, F. Razak, A. A. Verma In: Canadian Medical Association Journal, vol. 194, pp. E112-121, 2022. @article{ChanTCY.J092, Background: Disability-related considerations have largely been absent from the COVID-19 response, despite evidence that people with disabilities are at elevated risk for acquiring COVID-19. We evaluated clinical outcomes in patients who were admittedto hospital with COVID-19 with a disability compared with patients without a disability. Methods: We conducted a retrospective cohort study that included adults with COVID-19 who were admitted to hospital and discharged between Jan. 1, 2020, and Nov. 30, 2020, at 7 hospitals in Ontario, Canada. We compared in-hospital death, admission to the intensive care unit (ICU), hospital length of stay and unplanned 30-day readmission among patients with and without a physical disability, hearing or vision impairment, traumatic brain injury, or intellectual or developmental disability, overall and stratified by age (≤ 64 and ≥ 65 yr) using multivariable regression, controlling for sex, residence in a long-term care facility and comorbidity. Results: Among 1279 admissions to hospital for COVID-19, 22.3% had a disability. We found that patients with a disability were more likely to die than those without a disability (28.1% v. 17.6%), had longer hospital stays (median 13.9 v. 7.8 d) and more readmissions (17.6% v. 7.9%), but had lower ICU admission rates (22.5% v. 28.3%). After adjustment, there were no statistically significant differences between those with and without disabilities for in-hospital death or admission to ICU. After adjustment, patients with a disability had longer hospital stays (rate ratio 1.36, 95% confidence interval [CI] 1.19–1.56) and greater risk of readmission (relative risk 1.77, 95% CI 1.14–2.75). In age-stratified analyses, we observed longer hospital stays among patients with a disability than in those without, in both younger and older subgroups; readmission risk was driven by younger patients with a disability. Interpretation: Patients with a disability who were admitted to hospital with COVID-19 had longer stays and elevated readmission risk than those without disabilities. Disability-related needs should be addressed to support these patients in hospital and after discharge. |
8. | Sparse flexible design: A machine learning approach Journal Article T. C. Y. Chan, D. Letourneau, B. Potter In: Flexible Services and Manufacturing Journal, vol. 34, pp. 1066-1116, 2022. @article{ChanTCY.J091, For a general production network, state-of-the-art methods for constructing sparse flexible designs are heuristic in nature, typically computing a proxy for the quality of unseen networks and using that estimate in a greedy manner to modify a current design. This paper develops two machine learning-based approaches to constructing sparse flexible designs that leverage a neural network to accurately and quickly predict the performance of large numbers of candidate designs. We demonstrate that our heuristics are competitive with existing approaches and produce high-quality solutions for both balanced and unbalanced networks. Finally, we introduce a novel application of process flexibility in healthcare operations to demonstrate the effectiveness of our approach in a large numerical case study. We study the flexibility of linear accelerators that deliver radiation to treat various types of cancer. We demonstrate how clinical constraints can be easily absorbed into the machine learning subroutine and how our sparse flexible treatment networks meet or beat the performance of those designed by state-of-the-art methods. |
7. | An inverse optimization approach to measuring clinical pathway concordance Journal Article T. C. Y. Chan, M. Eberg, K. Forster, C. Holloway, L. Ieraci, Y. Shalaby, N. Yousefi In: Management Science, vol. 68, pp. 1882-1903, 2022. @article{ChanTCY.J088, Clinical pathways outline standardized processes in the delivery of care for a specific disease. Patient journeys through the healthcare system, however, can deviate substantially from these pathways. Given the positive benefits of clinical pathways, it is important to measure the concordance of patient pathways so that variations in health system performance or bottlenecks in the delivery of care can be detected, monitored, and acted upon. This paper proposes the first data-driven inverse optimization approach to measuring pathway concordance in any problem context. Our specific application considers clinical pathway concordance for stage III colon cancer. We develop a novel concordance metric and demonstrate using real patient data from Ontario, Canada that it has a statistically significant association with survival. Our methodological approach considers a patient’s journey as a walk in a directed graph, where the costs on the arcs are derived by solving an inverse shortest path problem. The inverse optimization model uses two sources of information to find the arc costs: reference pathways developed by a provincial cancer agency (primary) and data from real-world patient-related activity from patients with both positive and negative clinical outcomes (secondary). Thus, our inverse optimization framework extends existing models by including data points of both varying “primacy” and “alignment.” Data primacy is addressed through a two-stage approach to imputing the cost vector, whereas data alignment is addressed by a hybrid objective function that aims to minimize and maximize suboptimality error for different subsets of input data. |
6. | Optimizing inter-hospital patient transfer decisions during a pandemic: A queueing network approach Miscellaneous T. C. Y. Chan, F. Pogacar, V. Sarhangian, E. Hellsten, F. Razak, A. Verma 2021. @misc{ChanTCY.Pre014, Problem definition: Geographical mismatch between demand for care and availability of healthcare resources has been a major challenge during the COVID-19 pandemic. As such, inter-hospital patient transfers have emerged as a key aspect of the pandemic response in many countries. We propose and investigate inter-facility patient transfer policies with the goal of alleviating hospital congestion and reducing inequities in the distribution of COVID patients across the health system. Methodology/Results: We propose a queueing network model that captures the salient features of patient flow from acute wards to ICUs within each hospital, and between wards and ICUs of different hospitals in a network. We formulate the problem of determining optimal patient transfer policies between the hospitals as a stochastic control problem and develop a solution method by leveraging a deterministic fluid approximation of the queueing network. Using real data during the pandemic from a network of 21 hospitals in the Greater Toronto Area of Ontario, Canada, we validate our queueing model and conduct a comprehensive case study to examine the value of guiding patient transfers using our proposed approach. Compared to the no-transfer policy, the expected reduction in the number of patient days above a 95% occupancy threshold under our policy can be up to 43.6% in wards and 30.9% in ICUs, and the expected reduction in COVID load inequity can be up to 53.2%. In addition, we find that our optimized transfers outperform the actual transfer decisions made during the periods considered in the case study. Managerial implications: Guiding patient transfers using our proposed approach can be effective in alleviating congestion imbalances and reducing inequities during pandemics or other episodes of surge in hospitalizations (e.g., flu season). These benefits could be realized using a relatively small number of daily transfers. |
5. | Characteristics and outcomes of hospital admissions for COVID-19 and influenza in the Toronto area Journal Article A. A. Verma, T. Hora, H. Y. Jung, M. Fralick, S. L. Malecki, L. Lapointe-Shaw, A. Weinerman, T. Tang, J. L. Kwan, J. J. Liu, S. Rawal, T. C. Y. Chan, A. M. Cheung, L. C. Rosella, M. Ghassemi, M. Herridge, M. Mamdani, F. Razak In: Canadian Medical Association Journal, vol. 193, no. 22, pp. E410-E418, 2021. @article{ChanTCY.J081, BACKGROUND: Patient characteristics, clinical care, resource use and out- comes associated with admission to hospital for coronavirus disease 2019 (COVID-19) in Canada are not well described. METHODS: We described all adults with COVID-19 or influenza discharged from inpatient medical services and medical–surgical intensive care units (ICUs) between Nov. 1, 2019, and June 30, 2020, at 7 hospitals in Toronto and Mississauga, Ontario. We compared patient outcomes using multivariable regression models, controlling for patient sociodemographic factors and comorbidity level. We validated the accuracy of 7 externally developed risk scores to predict mortality among patients with COVID-19. RESULTS: There were 1027 hospital admissions with COVID-19 (median age 65 yr, 59.1% male) and 783 with influenza (median age 68 yr, 50.8% male). Patients younger than 50 years accounted for 21.2% of all admissions for COVID-19 and 24.0% of ICU admissions. Compared with influenza, patients with COVID-19 had significantly greater in-hospital mortality (unadjusted 19.9% v. 6.1%, adjusted relative risk [RR] 3.46, 95% confidence interval [CI] 2.56–4.68), ICU use (unadjusted 26.4% v. 18.0%, adjusted RR 1.50, 95% CI 1.25–1.80) and hospital length of stay (unadjusted median 8.7 d v. 4.8 d, adjusted rate ratio 1.45, 95% CI 1.25– 1.69). Thirty-day readmission was not significantly different (unadjusted 9.3% v. 9.6%, adjusted RR 0.98, 95% CI 0.70– 1.39). Three points-based risk scores for predicting in-hospital mortality showed good discrimination (area under the receiver operating characteristic curve [AUC] ranging from 0.72 to 0.81) and calibration. INTERPRETATION: During the first wave of the pandemic, admission to hospital for COVID-19 was associated with significantly greater mortality, ICU use and hospital length of stay than influenza. Simple risk scores can predict in- hospital mortality in patients with COVID-19 with good accuracy. |
4. | F. Razak, S. Shin, F. Pogacar, H. Y. Jung, L. Pus, A. Moser, L. Lapointe-Shaw, T. Tang, J. L. Kwan, A. Weinerman, S. Rawal, V. Kushnir, D. Mak, D. Martin, K. G. Shojania, S. Bhatia, P. Agarwal, G. Mukerji, M. Fralick, M. K. Kapral, M. Morgan, B. Wong, T. C. Y. Chan, A. A. Verma In: CMAJ Open, vol. 8, pp. E514-E521, 2020. @article{ChanTCY.J071, Background: The coronavirus disease 2019 (COVID-19) outbreak increases the importance of strategies to enhance urgent medical care delivery in long-term care (LTC) facilities that could potentially reduce transfers to emergency departments. The study objective was to model resource requirements to deliver virtual urgent medical care in LTC facilities. Methods: We used data from all general medicine inpatient admissions at 7 hospitals in the Greater Toronto Area, Ontario, Canada, over a 7.5-year period (Apr. 1, 2010, to Oct. 31, 2017) to estimate historical patterns of hospital resource use by LTC residents. We estimated an upper bound of potentially avoidable transfers by combining data on short admissions (≤ 72 h) with historical data on the proportion of transfers from LTC facilities for which patients were discharged from the emergency department without admission. Regression models were used to extrapolate future resource requirements, and queuing models were used to estimate physician staffing requirements to perform virtual assessments. Results: There were 235 375 admissions to general medicine wards, and residents of LTC facilities (age 16 yr or older) accounted for 9.3% (n = 21 948) of these admissions. Among the admissions of residents of LTC facilities, short admissions constituted 24.1% (n = 5297), and for 99.8% (n = 5284) of these admissions, the patient received laboratory testing, for 86.9% (n = 4604) the patient received plain radiography, for 41.5% (n = 2197) the patient received computed tomography and for 81.2% (n = 4300) the patient received intravenous medications. If all patients who have short admissions and are transferred from the emergency department were diverted to outpatient care, the average weekly demand for outpatient imaging per hospital would be 2.6 ultrasounds, 11.9 computed tomographic scans and 23.9 radiographs per week. The average daily volume of urgent medical virtual assessments would range from 2.0 to 5.8 per hospital. A single centralized virtual assessment centre staffed by 2 or 3 physicians would provide services similar in efficiency (measured by waiting time for physician assessment) to 7 separate centres staffed by 1 physician each. Interpretation: The provision of acute medical care to LTC residents at their facility would probably require rapid access to outpatient diagnostic imaging, within-facility access to laboratory services and intravenous medication and virtual consultations with physicians. The results of this study can inform efforts to deliver urgent medical care in LTC facilities in light of a potential surge in COVID-19 cases. |
3. | Negative spillover on service level across priority classes: Evidence from a radiology workflow platform Unpublished T. C. Y. Chan, N. Howard, S. Lagzi, B. F. Quiroga, G. Romero under review for Journal of Operations Management, 2020. @unpublished{ChanTCY.Pre007, Problem Definition: We study a radiology workflow platform that connects off-site radiologists with hospitals. These radiologists select tasks from a common pool, and the service level is characterized by meeting priority-specific turnaround time targets. However, imbalances between pay and workload of different tasks could result in higher priority tasks with low pay relative to workload receiving poorer service than low priority tasks. Using a large dataset, we empirically investigate this phenomenon. Academic/Practical Relevance: Piece-rate compensation schemes, where workers are paid for each completed task regardless of the time spent on it, are common in practice. Detecting a potential negative impact on firm performance associated with their use adds to the literature on challenges of piece-rate compensation schemes. Methodology: We employ an instrumental variable methodology to investigate whether low priority tasks with a high pay-to-workload ratio have a shorter turnaround time. Then, using the same approach, we investigate whether having many low priority tasks with high pay-to-workload increases the turnaround time and probability of delay of higher priority tasks. Results: We show that turnaround time is decreasing in pay-to-workload for lower priority tasks, whereas it is increasing in workload for high priority tasks. More importantly, we find evidence of a spillover effect: Having many economically attractive tasks with low priority can lead to longer turnaround times for higher priority tasks, increasing the likelihood that those tasks are delayed. Implications: Our results suggest that organizations where workers have task discretion from a common pool need to carefully align their piece-rate compensation scheme with the workload of each task. Imbalances may lead to a degradation in the system service level provided to time-sensitive customers. |
2. | Ambulance Emergency Response Optimization in developing countries Journal Article J. J. Boutilier, T. C. Y. Chan In: Operations Research, vol. 68, pp. 1315-1334, 2020. @article{ChanTCY.J060, The lack of emergency medical transportation is viewed as the main barrier to the access and availability of emergency medical care in low- and middle-income countries (LMICs). In this paper, we present a robust optimization approach to optimize both the location and routing of emergency response vehicles, accounting for uncertainty in travel times and spatial demand characteristic of LMICs. We traveled to Dhaka, Bangladesh, the sixth largest and third most densely populated city in the world, to conduct field research resulting in the collection of two unique data sets that inform our approach. These data are leveraged to estimate demand for emergency medical services in an LMIC setting and to predict the travel time between any two locations in the road network for different times of day and days of the week. We combine our prediction-optimization framework with a simulation model and real data to provide an in-depth investigation into three policy- related questions. First, we demonstrate that outpost locations optimized for weekday rush hour lead to good performance for all times of day and days of the week. Second, we find that the performance of the current system could be replicated using one third of the current outpost locations and one half of the current number of ambulances. Finally, we show that a fleet of small ambulances has the potential to significantly outperform tra- ditional ambulance vans. In particular, they are able to capture approximately three times more demand while reducing the median average response time by roughly 10%–18% over the entire week and 24%–35% during rush hour because of increased routing flexibility offered by more nimble vehicles on a larger road network. Our results provide practical insights for emergency response optimization that can be leveraged by hospital-based and private ambulance providers in Dhaka and other urban centers in developing countries. |
1. | Women's College Hospital uses Operations Research to create an ambulatory clinic schedule Journal Article B. K. Eagen, T. C. Y. Chan, M. W. Carter In: Service Science, vol. 10, pp. 230-240, 2018. @article{ChanTCY.J042, Women's College Hospital (WCH) in Toronto, Canada, offers roughly 300 outpatient clinics every week. In April 2011, we started working with WCH to design a new schedule for their clinics, to accommodate a move to a new hospital building that was completed in May 2013. We developed an integer programming model to optimize the assignment of clinics to timeslots and locations, based on the desire to minimize changes from the historical schedule. In cooperation with senior leadership, we tested multiple scenarios that explored changes to space utilization policies at WCH and ultimately generated a new clinic schedule, which they implemented in May 2013. In this paper, we highlight the value our work has created for WCH and present the lessons we learned in development of the model and through our collaboration with the WCH team. |
Sustainability
Although I am not currently active in this area, I have previous experience applying optimization methods to problems in green building design and wind farm layout.
RELEVANT PUBLICATIONS
8. | Income pools for superstar markets Journal Article Forthcoming T. C. Y. Chan, N. Chen, C. Fernandes In: Management Science, Forthcoming. @article{ChanTCY.J132, “Superstar” markets, characterized by a small portion of individuals earning disproportionately large salaries compared to their peers, have been identified in industries such as entrepreneurship, sports, music and entertainment. Individuals entering these markets face extreme income uncertainty which may deter some of them from entering altogether or force them to exit early. To address this difficulty, we propose income pools. An income pool involves individuals agreeing that if any one of them reaches a particular salary milestone, a portion of their future earnings will be shared amongst all members in their pool. Despite growing interest and implementation of income pools in practice, they have not been studied yet in the academic literature. In response, we develop the first mathematical model to analyze income pools, focusing on stability (i.e., pools where no agents leave or join). We concentrate on risk-averse agents and show that they prefer to join income pools, but no finite-sized stable pool exists. There are two remedies to this instability. First, by introducing an upper bound on pool size, we show that a bounded stable pool always exists and is easily identifiable. In general, these pools require more “weaker” (i.e., lower chances of success) agents than strong ones to remain stable. Interestingly, we prove a “Pareto dominance” result, whereby all agents in a given bounded stable pool will simultaneously prefer a unique bounded stable pool. Second, by introducing a cost ϵ to leave/join a pool, we show that finite-sized ϵ-stable pools always exist and provide a sufficient condition to identify them. We analyze two extensions, individualized contracts and single-winner-take-all markets, and show that the instability result persists in both scenarios. We conclude with a case study using real professional baseball player data that demonstrates a 20%-30% increase in social welfare if players join income pools, under varying income pool contract parameters. This increase is highest for players with the lowest probability of reaching superstardom. We present practical implications of our theoretical and numerical results in terms of creating finite-sized pools. |
7. | Exploring the geographical equity-efficiency tradeoff in cycling infrastructure planning Journal Article M. Bonsma-Fisher, B. Lin, T. C. Y. Chan, S. Saxe In: Journal of Transport Geography, vol. 121, pp. 104010, 2024. @article{ChanTCY.J124, Cycling is affordable, healthy, and sustainable, but access to destinations on low-stress safe cycling routes in most cities is both limited and unevenly distributed. Many cities are expanding cycling networks to improve safety, increase cycling mode share, and increase diversity in access to cycling, however resources remain limited which requires prioritization of infrastructure. When proposed infrastructure locations are optimized to provide the highest average access to opportunities using a utilitarian definition of accessibility, marginalized groups and locations may be further left behind. This occurs since the greatest gains to network connectivity, using a utility definition, come from expansions inside or directly adjacent to the densest network areas. We compare utilitarian and equity-driven planning strategies for cycling network expansion and explore tradeoffs in spatial coverage, equity, and efficiency, using Toronto, Canada as a case study. We find that optimizing accessibility in several small regions instead of city-wide leads to an infrastructure plan that is more spatially distributed. Further, we show that a model targeting low-access areas produces an infrastructure plan with more regions meeting a minimum threshold of accessibility but with lower average accessibility gains, indicating the presence of an equity-efficiency tradeoff. We also find that infrastructure projects that maximize a region's accessibility to destinations are often located outside that region, challenging political perceptions of `local' infrastructure and benefits. These results inform planning, advocacy, design, and policy, and shed light on spatial and socio-demographic equity tradeoffs in deciding where to add cycling infrastructure. |
6. | A. A. Imrit, J. Fischer, T. C. Y. Chan, S. Saxe, M. Bonsma-Fisher In: Findings, vol. 2024, no. doi.org/10.32866/001c.92109, 2024. @article{ChanTCY.J0115, This study uses Strava bicycling data to investigate network level patterns of bicycle ridership in Toronto, Canada based on Level of Traffic Stress (LTS). We found that most bicycling occurred on a small fraction of the network, with just 10% of all roads and paths accounting for 75% of all bicycle kilometres travelled in 2022. Low-stress routes (LTS 1 and LTS 2) were more popular than high-stress routes for the top 80% most popular streets. The majority of bicycle kilometres travelled (84%) in LTS 2 occurred on routes with no bicycle infrastructure, highlighting the importance of quiet residential streets in forming a low-stress bike network. Despite high-stress conditions, some LTS 3 and LTS 4 streets were heavily used, suggesting infrastructure gaps in Toronto’s bicycle network. |
5. | The impact of COVID-19 cycling infrastructure on low-stress cycling accessibility: A case study in the city of Toronto Journal Article B. Lin, T. C. Y. Chan, S. Saxe In: Findings, vol. 2021, no. doi.org/10.32866/001c.19069, 2021. @article{ChanTCY.J082, This paper investigates the impact of COVID-19 cycling infrastructure on Toronto’s low-stress cycling accessibility. We calculated the level of traffic stress (LTS) for the road network with and without the cycling infrastructure built in response to the COVID-19 pandemic. We then computed the accessibility to populations, jobs, food stores, and parks for each dissemination area at each LTS. We find that the COVID-19 cycling infrastructure increased low-stress population and job accessibility by 10.4% and 22.3% respectively, and granted food and park access to 54,023 and 3,178 people. Accessibility gains were largest in areas where new infrastructure linked with preexisting networks. |
4. | Robust wind farm layout optimization Book Chapter P. Y. Zhang, J. Y. J. Kuo, D. Romero, T. C. Y. Chan, C. H. Amon In: Terlaky, T.; Anjos, M.; Ahmed, S. (Ed.): Advances and Trends in Optimization with Engineering Applications, Chapter 28, pp. 367-374, SIAM, Philadelphia, 2017, ISBN: 978-1-61197-468-3. @inbook{ChanTCY.Oth009, The number of commercial-scale wind farms and total installed capacity have grown dramatically over the past two decades [1958]. Relying on an essentially free but intermittent energy source and spanning large areas of land or water, wind farms face unique challenges in their design, installation, and operations. Two types of optimization problems arise naturally from this context: (a) optimization of aggregated energy output by strategic turbine placement and (b) optimization of electrical dispatch policies to reduce the economic impact of wind intermittency. In this chapter, we apply robust optimization to the former and discuss the potential benefits on both total energy output and variability in energy output, which can impact dispatch policies. |
3. | A new mathematical programming approach to optimize wind farm layouts Journal Article S. D. O. Turner, D. A. Romero, P. Y. Zhang, C. H. Amon, T. C. Y. Chan In: Renewable Energy, vol. 63, pp. 674-680, 2014. @article{ChanTCY.J016, The optimal placement of turbines in a wind farm is critical to the maximization of power production. In this paper, we develop a new mathematical programming approach for wind farm layout optimization. We use Jensen's wake decay model to represent multi-turbine wake effects. We develop mixed integer linear and quadratic optimization formulations and apply them to several example layout cases in the literature. Compared to previous approaches, our models produce layouts that tend to be more symmetric and that generate slightly more power. Our formulations solve quickly, allowing a decision maker to efficiently explore the impact of different turbine densities in a wind farm. |
2. | Examining the LEED rating system using inverse optimization Journal Article S. D. O. Turner, T. C. Y. Chan In: Journal of Solar Energy Engineering, vol. 135, no. Article No. 040901, 2013. @article{ChanTCY.J014, The Leadership in Energy and Environmental Design (LEED) rating system is the most recognized green building certification program in North America. In order to be LEED certified, a building must earn a sufficient number of points, which are obtained through achieving certain credits or design elements. In LEED versions 1 and 2, each credit was worth one point. In version 3, the LEED system changed so that certain credits were worth more than one point. In this study, we develop an inverse optimization approach to examine how building designers intrinsically valued design elements in LEED version 2. Because of the change in the point system between version 2 and version 3, we aim to determine whether building designers actually valued each credit equally, and if not, whether their valuations matched the values in version 3. Due to the large dimensionality of the inverse optimization problem, we develop an approximation to improve tractability. We apply our method to 306 different LEED-certified buildings in the continental United States. We find that building designers did not value all credits equally and that other factors such as cost, building type, and size, and certification level play a role in how the credits are valued. Overall, inverse optimization may provide a new method to assess historical data and support the design of future versions of LEED. |
1. | Examining the LEED rating system using approximate inverse optimization Proceedings Article S. D. O. Turner, T. C. Y. Chan In: Proceedings of the ASME 2012 International Mechanical Engineering Congress and Exposition, 2012. @inproceedings{ChanTCY.Oth004c, The Leadership in Energy and Environmental Design (LEED) rating system is the most recognized green building certification program in North America. In order to be LEED certified, a building must earn a certain number of points, which are obtained through achieving certain credits or design elements. Prior to LEED version 3, each credit was worth one point. In this study, we develop an inverse optimization approach to examine how building designers intrinsically valued design elements in LEED version 2. Due to the large dimensionality of the inverse optimization problem, we develop an approximation to improve tractability. We apply our method to 18 different LEED-certified buildings in the United States. We find that building designers did not value all credits equally and that other factors such as cost and certification level play a role in how the credits are valued. Overall, inverse optimization may provide a new method to assess historical data and support the design of future versions of LEED. |
Education
I enjoy developing innovative teaching methods using games and other interactive activities.
RELEVANT PUBLICATIONS
5. | Case ― Moneyball for Murderball: Using analytics to construct lineups in wheelchair rugby Journal Article T. C. Y. Chan, C. Fernandes, A. Loa, N. Sandholtz In: INFORMS Transactions on Education, vol. 24, no. 2, pp. 182-183, 2024. @article{ChanTCY.J112, |
4. | Case Article ― Moneyball for Murderball: Using analytics to construct lineups in wheelchair rugby Journal Article T. C. Y. Chan, C. Fernandes, A. Loa, N. Sandholtz In: INFORMS Transactions on Education, vol. 24, no. 2, pp. 175-181, 2024. @article{ChanTCY.J111, Motivated by the problem of lineup optimization in wheelchair rugby (WCR), this case study covers descriptive, predictive, and prescriptive analytics. The case is presented from the perspective of a new assistant coach of Canada’s national WCR team, who has been tasked by the head coach to use various analytics techniques to improve their lineups. Whereas the data and actors are fictitious, they are based on real data and discussions with the national team coach and sport scientists. To solve the case, students must conduct data analysis, regression modeling, and optimization modeling. These three steps are tightly linked, as the data analysis is needed to prepare the data for regression, and the regression outputs are used as parameters in the optimization. As such, students build proficiency in developing an end-to-end solution approach for a complex real-world problem. The primary learning objectives for the students are to understand the differences between descriptive, predictive, and prescriptive analytics, to build proficiency in implementing the models using appropriate software, and to identify how these techniques can be applied to solve problems in other sports or other application areas. |
3. | Constrained optimization for decision making in healthcare using Python: A tutorial Journal Article K. H. B. Leung, N. Yousefi, T. C. Y. Chan, A. M. Bayoumi In: Medical Decision Making, vol. 43, no. 7-8, pp. 760-773, 2023. @article{ChanTCY.J0108, Constrained optimization can be used to make decisions aimed at maximizing some quantity in the face of fixed limits, such as resource allocation problems in health where tradeoffs between alternatives are inherent, and has been applied in a variety of health-related applications. This tutorial guides the reader through the process of mathematially formulating a constrained optimization problem, providing intuitive explanations for each component within the problem. We discuss how constrained optimization problems can be implemented using software and provide instructions on how to set up a solution environment using Python and the Gurobi solver engine. We present 2 examples from the existing literature that illustrate different constrained optimization problems in health and provide the reader with Python code used to solve these problems as well as a discussion of results and sensitivity analyses. This tutorial can be used to help readers formulate constrained optimization problems in their own application domains. |
2. | Introducing and integrating machine learning in an operations research curriculum: An application-driven course Journal Article J. J. Boutilier, T. C. Y. Chan In: INFORMS Transactions on Education, vol. 23, no. 2, pp. 64-83, 2023. @article{ChanTCY.J0103, Artificial intelligence (AI) and operations research (OR) have long been intertwined because of their synergistic relationship. Given the increasing popularity of AI and machine learning in particular, we face growing demand for educational offerings in this area from our students. This paper describes two courses that introduce machine learning concepts to undergraduate, predominantly industrial engineering and operations research students. Instead of taking a methods-first approach, these courses use real-world applications to motivate, introduce, and explore these machine learning techniques and highlight meaningful overlap with operations research. Significant hands-on coding experience is used to build student proficiency with the techniques. Student feedback indicates that these courses have greatly increased student interest in machine learning and appreciation of the real-world impact that analytics can have and helped students develop practical skills that they can apply. We believe that similar application-driven courses that connect machine learning and operations research would be valuable additions to undergraduate OR curricula broadly. |
1. | Deal or No Deal: A spreadsheet game to introduce decision making under uncertainty Journal Article T. C. Y. Chan In: INFORMS Transactions on Education, vol. 14, pp. 53-60, 2013. @article{ChanTCY.J013, In this paper, I introduce a spreadsheet-based implementation of the game show Deal or No Deal. I describe how this game can be used in class to illuminate topics in decision making under uncertainty to students in both engineering and business. I show that specific scenarios encountered in the game can lead to rich discussions on topics like risk, utility, and probability. The game is easy to learn and play in class and usually receives a strong positive response from students. |
Redeploy
A software tool to optimize matching of available hospital staff to job requests during COVID-19
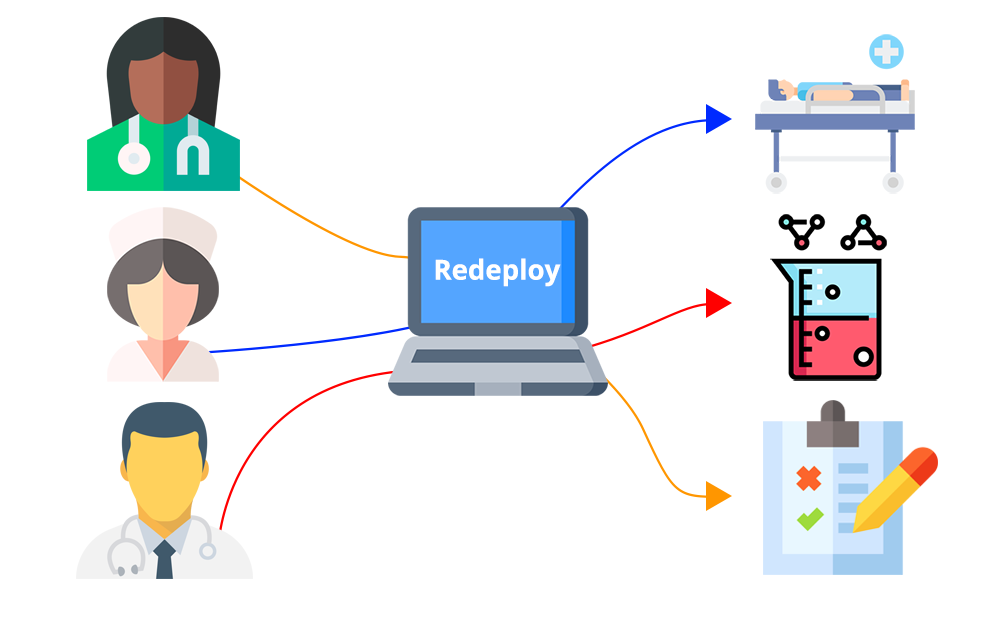
A suite of optimization models tailored for the 2017 and 2021 NHL Expansion drafts that allow users to modify objectives and constraints, and evaluate what-if scenarios.
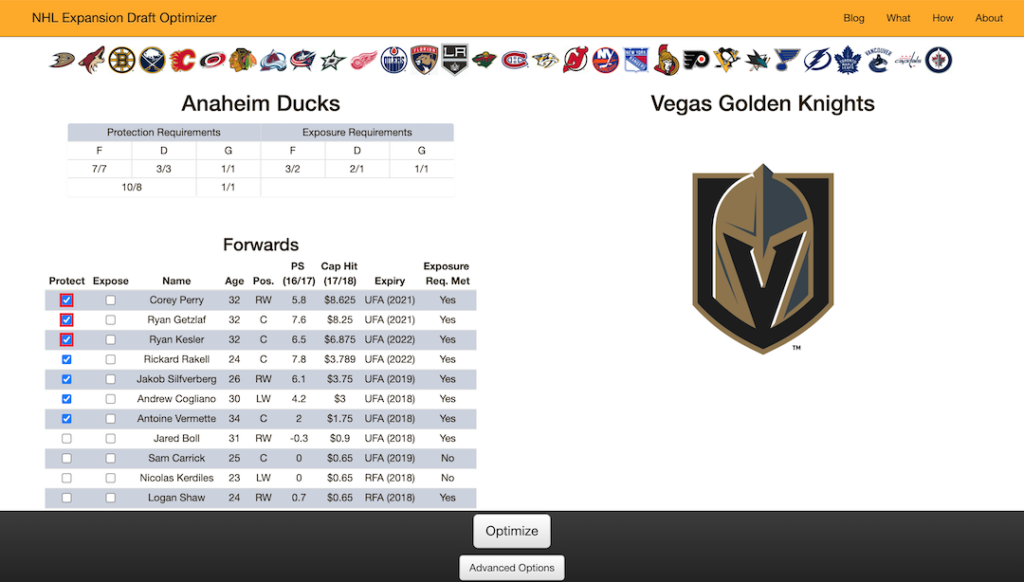
High-performance analytics for sports
An initiative to grow research, student training, industry partnerships, and equity, diversity and inclusion (EDI) in sports analytics.
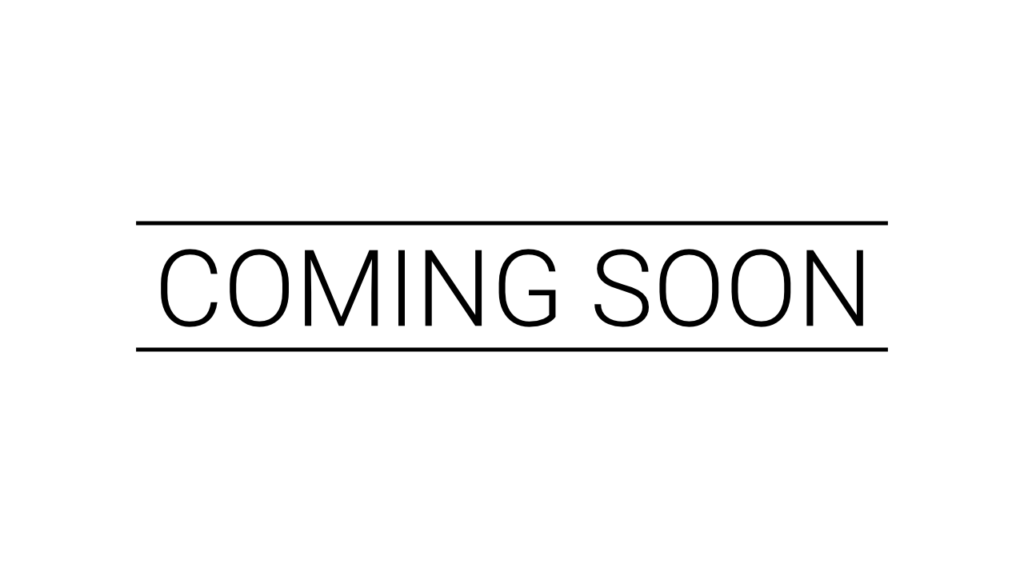
An international competition sponsored by the American Association of Physicists in Medicine to advance dose prediction methods for knowledge-based planning.
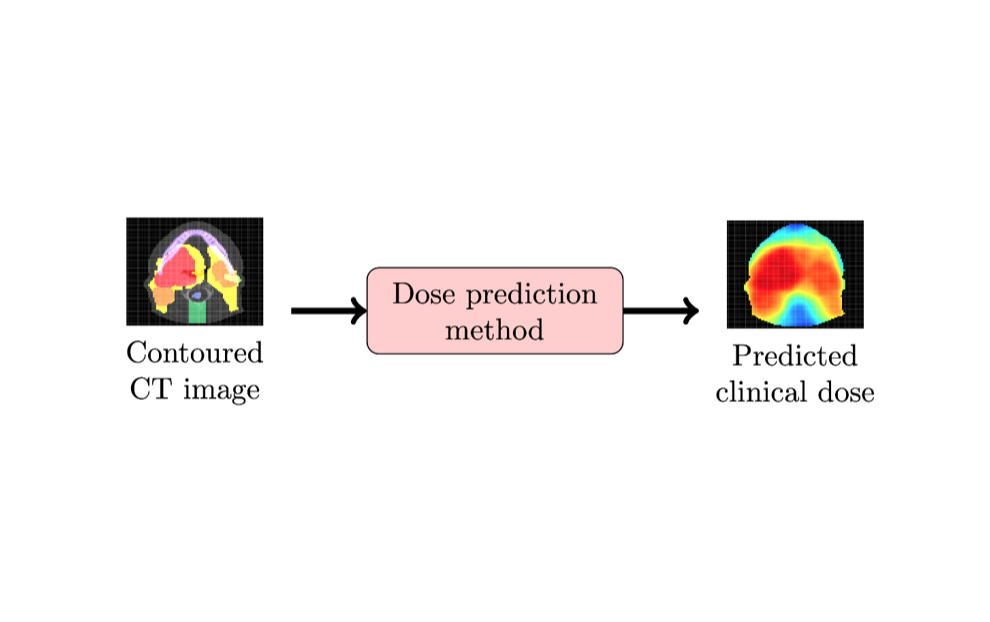
Support from the following sponsors is gratefully acknowledged
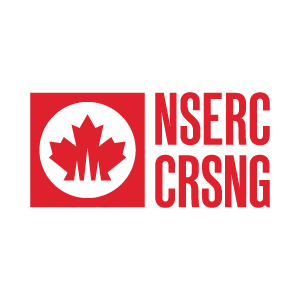
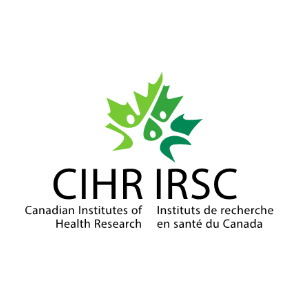
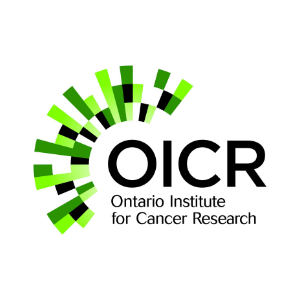
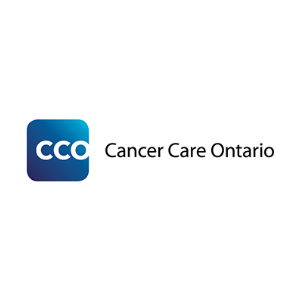
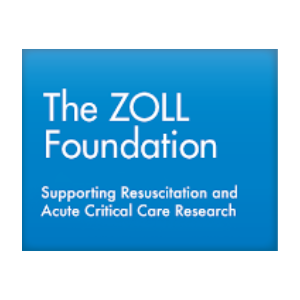
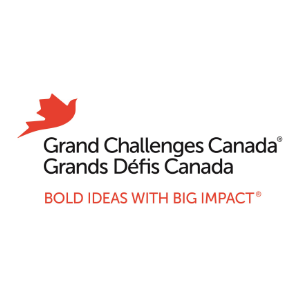
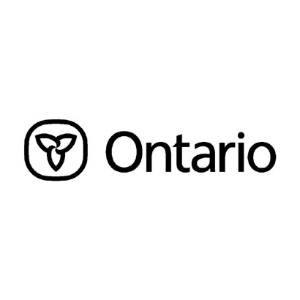
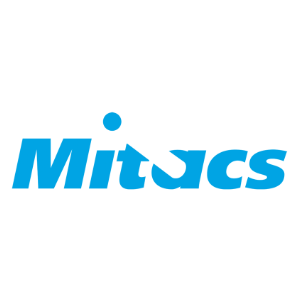
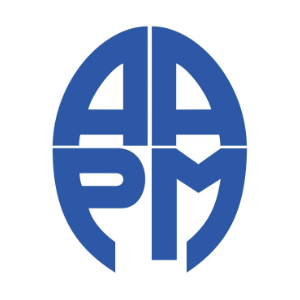
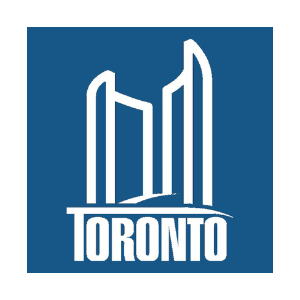
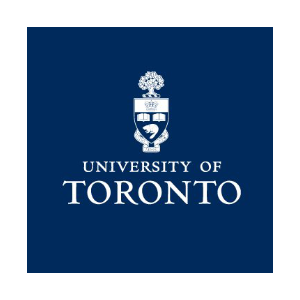